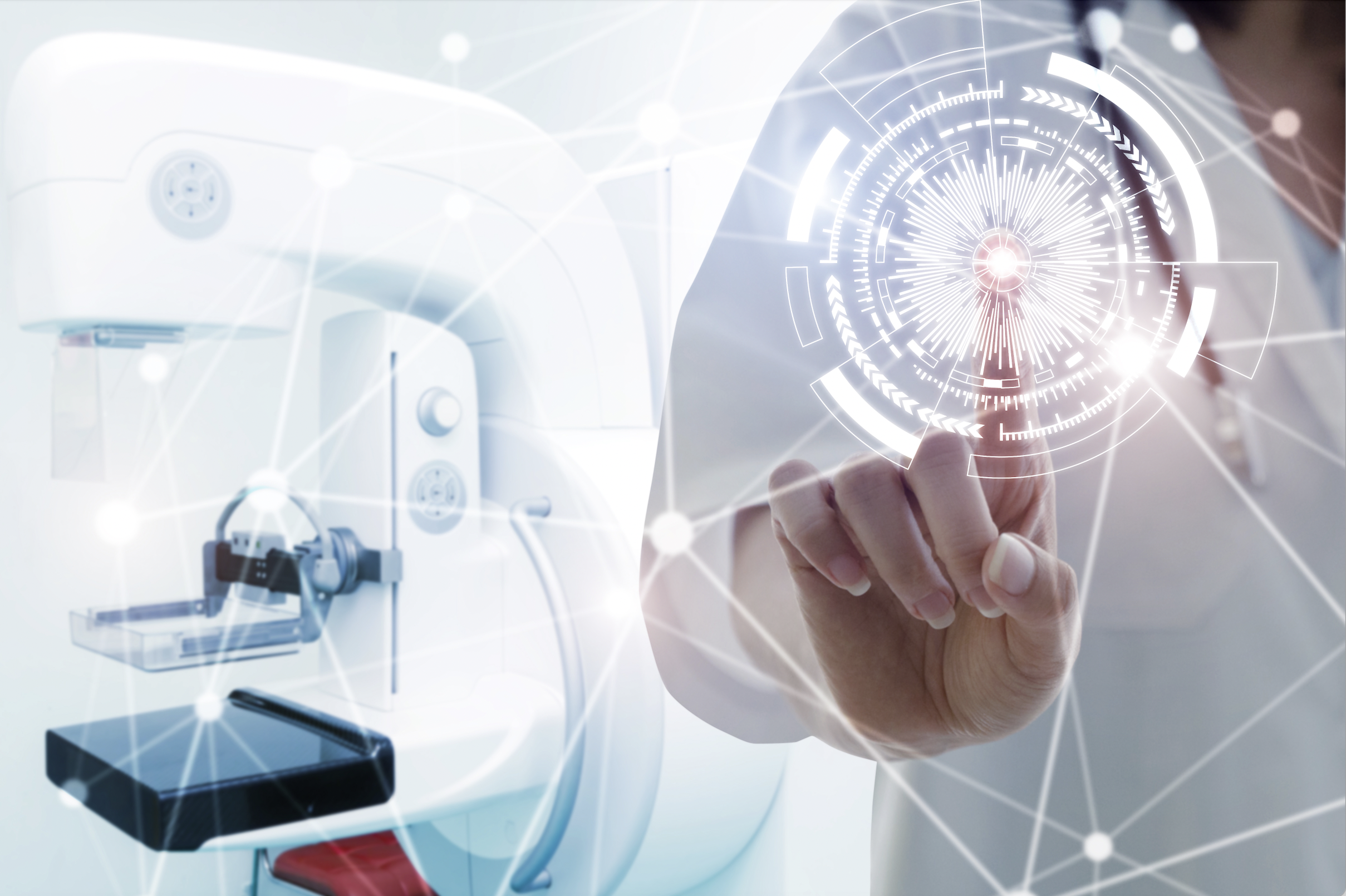
Breast cancer remains a significant health concern, responsible for more than 600,000 deaths annually. Early detection, particularly through screening, is pivotal in mitigating its impact. The current gold standard for breast cancer detection is annual screening mammography starting at age 40, as per the guidelines of the American College of Radiology and the American Cancer Society.¹ Annual mammographic screening has been shown to reduce breast cancer deaths and catch cancers at earlier and more treatable stages. While mammography has been instrumental in the early treatment of breast cancer, it has known limitations, particularly in the setting of dense fibroglandular breast tissue.² In recent years, there has been a surge of innovative technologies aimed at overcoming the limitations inherent in traditional screening approaches. These advances promise to both increase the accuracy and timeliness of cancer detection in the future.
Mammographic screening is designed to detect structural changes in the breast parenchyma caused by cancer over time. This requires compression of the breast, which can be challenging for many patients, and a small dose of radiation. Advanced imaging techniques such as cone beam breast computed tomography (CBCT), contrast-enhanced mammography (CEM), abbreviated breast magnetic resonance imaging (AB-MRI) and ultrasound tomography (UT) all seek to address the limitations of breast cancer by removing the need for compression, by avoiding ionizing radiation or by allowing functional imaging of the breast tissue to better identify early and interval breast cancers.
The rapid rise and development of artificial intelligence (AI) in radiology has been particularly notable in breast imaging, where it is being rapidly implemented into the clinical workflow. By using sophisticated algorithms capable of learning and adapting, AI has the capability to assist and augment radiologists as they interpret mammograms and other breast imaging. This has been shown to both increase accuracy and decrease false positive imaging. AI is also poised to usher in a new level of personalized breast cancer risk assessment, allowing more accurate identification of patients who could benefit from additional screening methods.
Advances in Imaging Technologies Contrast-Enhanced Mammography (CEM)
Contrast-enhanced mammography (CEM) builds on full field digital mammography by adding an injection of iodine-based contrast agent to highlight the areas of increased vascularity often associated with tumor growth. After injection, subtraction images are generated through dual-energy mammographic imaging, similar to how post-contrast subtraction images are used in breast MRI. The use of the contrast agent thus combines the tissue resolution of mammography with the functional imaging of the contrast agent, enhancing suspicious lesion visibility.
CEM has reported sensitivity rates between 91-100%, which compares favorably to even mammography combined with screening ultrasound, although not as high as that of breast MRI (97%). Recent studies have suggested that CEM may be comparable to MRI in both lesion detection and assessment of breast cancer disease extent.³ The higher detection rate of CEM compared to standard mammography (including digital breast tomosynthesis) shows the added value of physiologic imaging.
A drawback of CEM is the use of iodinated contrast, which causes a higher rate of contrast reactions than gadolinium-based MRI contrast and is contraindicated in chronic kidney disease. However, as CEM is a rapid, relatively low-cost exam that requires the same footprint as a standard mammographic unit, this may increase the accessibility of functional breast imaging in women who need additional screening or workup but cannot tolerate or access breast MRI. Future studies of CEM, particularly compared to abbreviated breast MRI, are needed.
Abbreviated Breast Magnetic Resonance Imaging (AB-MRI)
Breast MRI is currently recommended for breast cancer screening in patients at intermediate (>15%) and high lifetime risk (>20%) of breast cancer. This includes patients who are genetic mutation carriers known to be at increased risk of breast cancer (BRCA1, BRCA2, CHEK2 and others), women with a strong family history of premenopausal breast cancer, and those with a high lifetime risk as determined through breast cancer risk assessment. Breast MRI has also been shown to have high cancer detection rates in women at average risk of breast cancer, although with an increased chance of false-positive biopsies. However, breast MRI is currently underutilized by patients due to a combination of cost, limited availability in some regions and an inability to tolerate the examination length.
AB-MRI uses a shorter imaging time to detect breast cancer. Similar to CEM, the patient has intravenous contrast injected during the exam; unlike CEM, the contrast is gadolinium-based, which is less likely to cause a contrast reaction. The exam time is ten minutes or less, compared to 20-30 minutes for a standard breast MRI, which is better tolerated, less expensive and allows more women to undergo screening than traditional MRI.⁴
AB-MRI allows the combination of dynamic enhancement information in breast lesions with three-dimensional breast anatomy, including the simultaneous evaluation of axillary lymph nodes. Compared to routine breast MRI, there is no significant reduction in performance of AB-MRI compared with a full diagnostic MRI in either sensitivity or specificity.⁴ Recent multicenter studies comparing AB-MRI to digital breast tomosynthesis (DBT) have shown that AB-MRI detects more cancers than DBT in women with dense breasts and women with a personal history of breast cancer, although they are more likely to be recommended for short-term imaging follow-up examinations.⁵ AB-MRI has also been shown to be effective for the evaluation of patients with known breast cancer.
Future use of specific MRI techniques such as ultrafast imaging or additional advanced techniques in breast MRI may further improve specificity and decrease false-positive biopsies. For now, AB-MRI offers a way for more women at increased risk of breast cancer to access the supplemental screening modality that has the highest cancer detection rate.
Cone-beam Breast Computed Tomography (CBBCT)
Cone-beam breast computed tomography (CBBCT) is an attempt to improve on the limitations of both mammography and breast MRI. Both mammography and breast MRI require breast compression for accurate images to be taken, which is difficult for some patients to tolerate. Breast MRI allows the patient to lie prone but further requires the arms to be positioned over the patient’s head, which is difficult for a patient with a history of shoulder surgery or arthritis. CBBCT allows the patient to lie prone without compression for imaging and provides three-dimensional images at a higher resolution than breast MRI, including visualization of calcifications. The three-dimensional resolution allows improved resolution in dense breasts compared to digital breast tomosynthesis, with less tissue overlap.
Studies of contrast-enhanced CBBCT have shown that it has improved sensitivity compared to mammography and approaches the sensitivity of breast MRI.⁶ Current limitations of CBBCT are that it requires iodinated contrast, with the same drawbacks of CEM. Although CEM is readily installed in a mammography footprint, CBBCT requires specialized equipment installation that cannot be repurposed for other imaging exams, unlike an MRI. Further studies of CBBCT comparing it to other modalities used for supplemental screening, particularly CEM and FAST MRI, are needed.
Ultrasound Tomography (UT)
Supplemental screening ultrasound is the most common supplemental screening modality for women at increased risk of breast cancer due to its accessibility, relatively low cost and ease of use. However, screening ultrasound has a relatively low additional cancer detection rate and, when performed by a radiologist or technologist, is dependent on the skill of the operator. Recent FDA-approved ultrasound tomography (UT) improves on standard breast ultrasound by capturing tomographic, three-dimensional views of the breast tissue to improve lesion detection and characterization. Similar to CBBCT and MRI, the patient lies prone, and there is minimal compression compared to mammography.
UT does not require ionizing radiation or injected contrast. However, unlike CEM, CBBCT and FAST MRI, this means it evaluates tissue structure alone without physiological information from contrast inflow, limiting its cancer detection rate compared to these modalities. UT, however, shows potential in supplemental breast cancer screening, assessing breast density, tumor volume, and tumor response to neoadjuvant therapy.⁷
Personalized Risk Assessment
Updated American College of Radiology breast cancer risk screening guidelines suggest all women undergo risk assessment by age 25 to see if earlier or more intensive breast cancer screening is needed. This is particularly recommended for Black and Ashkenazi Jewish women, who are more likely to be diagnosed at a younger age and with more aggressive breast cancer types. Current risk assessment models are based on personal and family history of breast cancer and are population-based; they are used to stratify women at low, intermediate and high lifetime risk of breast cancer. However, these models are static and do not take into account changes in risk factors over time.
Personalized breast cancer screening is rapidly becoming a reality, with multiple trials in progress to assess if screening intervals can be modified further as patients are more precisely identified as high or low risk based on additional information including genetics. Recent studies have shown that including information from the patient’s current mammogram or breast MRI can further pinpoint a patient’s short- and intermediate-term risk of breast cancer.⁸ More importantly, these AI imaging-based risk models do not require extensive personal and family histories, appear to be more robust across different ethnicities, and can be analyzed at the time of the screening breast exam instead of requiring a separate office visit. In the not-so-far-off future, these imaging-based risk models can be combined with liquid biopsy tests to allow even more precise estimation of breast cancer risk.
The AI Frontier in Breast Imaging
Breast imaging remains at the forefront of clinical AI implementation in radiology, with over 22 FDA-approved clinical algorithms. Radiologists have used computer-aided detection since the 1990s to assist in the interpretation of mammograms. However, these detection programs ultimately were not proven to increase cancer detection rates. New advances in machine learning and artificial intelligence computer-aided detection have leveraged large datasets of more than a million mammograms. These AI systems can detect cancer at rates comparable to that of experienced breast imagers, with the best performance coming from the combination of a human and AI reader and are now in routine clinical use at many practice sites.
Recent large prospective studies have confirmed that the use of AI in mammography and digital breast tomosynthesis is comparable or better to human reader performance with no significant increase in recall rates.⁹ These studies were performed, however, in countries where two radiologists routinely read each mammogram. While this is likely generalizable to the United States, similar large-scale studies are needed to confirm these performance results.
AI-based computer-aided diagnosis (CADx) and detection (CADe) systems for breast ultrasound and breast MRI have shown similar high performance in distinguishing benign from malignant lesions with similar or greater accuracy than radiologists. Like mammography and DBT studies, the best performance comes from the hybridization of expert readers and AI systems, with the greatest gains in performance seen in less experienced readers. Studies of AI have shown that it cannot just decrease false-positive recalls and biopsies but also potentially identify more biologically aggressive cancer subtypes, response to neoadjuvant therapy, and risk of axillary metastases in all breast modalities.¹⁰
AI-based models have similar promise in the triage of more concerning exams to be prioritized by radiologists, in the assessment of mammographic breast density and in improving breast imaging quality, with commercially approved AI models for all three applications. Large language models (LLMs) are being used to assist in writing and proofreading radiologist reports.¹⁰ Future directions in AI in breast imaging include the development of multimodal models that can incorporate electronic health record information such as laboratory values and patient history along with the pertinent medical imaging to improve not only diagnosis but reporting as well.
Although the further use of AI in both radiology and medicine has enormous potential, existing concerns about patient privacy, potential bias, interpretability and potential model drift remain and are important to address.¹¹ Nonetheless, the rapid clinical adoption of AI-based CAD programs for breast imaging demonstrates that once validated, these new models can improve radiologist accuracy and patient care.
A New Era of Precision
There have been significant strides in combating breast cancer through innovative imaging technologies and AI. These advancements not only enhance early detection but also promise personalized risk assessments, which are crucial in reducing breast cancer mortality. Moreover, the integration of AI in radiology can bolster the accuracy and timeliness of cancer detection, ushering a new era of precision in breast imaging. Although challenges remain, particularly in the growing implementation of AI in breast cancer evaluation, the future of breast imaging in radiology is bright. Through new advances in screening, detection and risk assessment, radiologists can continue to reduce the mortality associated with breast cancer and improve patient care.
Laura Heacock, MS, MD, is a breast imaging specialist in the Department of Radiology at NYU Langone Perlmutter Cancer Center, where she uses advanced imaging technologies to provide comprehensive screening, diagnostic and interventional imaging services for breast diseases.
REFERENCES:
1. https://www.breastcancer.org/screening-testing/mammograms/recommendatio…
2. Hussein, Heba, et al. “Supplemental breast cancer screening in women with dense breasts and negative mammography: a systematic review and meta-analysis.” Radiology 306.3 (2023): e221785.
3. Neeter, Lidewij MFH, et al. “Comparing the diagnostic performance of contrast-enhanced mammography and breast MRI: A systematic review and meta-analysis.” Journal of Cancer 14.1 (2023): 174. https://www.ncbi.nlm.nih.gov/pmc/articles/PMC9809339/
4. Geach, R., et al. “The potential utility of abbreviated breast MRI (FAST MRI) as a tool for breast cancer screening: a systematic review and meta-analysis.” Clinical Radiology 76.2 (2021): 154-e11.
5. Comstock, Christopher E., et al. “Comparison of abbreviated breast MRI vs digital breast tomosynthesis for breast cancer detection among women with dense breasts undergoing screening.” Jama 323.8 (2020): 746-756.
6. Wienbeck, Susanne, et al. “Contrast-enhanced cone-beam breast-CT (CBBCT): clinical performance compared to mammography and MRI.” European radiology 28 (2018): 3731-3741.
7. Littrup, Peter J., et al. “Multicenter study of whole breast stiffness imaging by ultrasound tomography (SoftVue) for characterization of breast tissues and masses.” Journal of Clinical Medicine 10.23 (2021): 5528.
8. Acciavatti, Raymond J., et al. “Beyond breast density: risk measures for breast cancer in multiple imaging modalities.” Radiology 306.3 (2023): e222575.
9. Lång, Kristina, et al. “Artificial intelligence-supported screen reading versus standard double reading in the Mammography Screening with Artificial Intelligence trial (MASAI): a clinical safety analysis of a randomized, controlled, non-inferiority, single-blinded, screening accuracy study.” The Lancet Oncology 24.8 (2023): 936-944.
10. Taylor, Clayton R., et al. “Artificial Intelligence Applications in Breast Imaging: Current Status and Future Directions.” Diagnostics 13.12 (2023): 2041.
11. Morgan, Matthew B., and Jonathan L. Mates. “Ethics of artificial intelligence in breast imaging.” Journal of Breast Imaging 5.2 (2023): 195-200.
Related Breast Density Content:
VIDEO: FDA Update on the US National Density Reporting Standard - A Discussion on the Final Rule
One on One … with Wendie Berg, MD, PhD, FACR, FSBI
Task Force Issues New Draft Recommendation Statement on Screening for Breast Cancer
Creating Patient Equity: A Breast Density Legislative Update
AI Provides Accurate Breast Density Classification
VIDEO: The Impact of Breast Density Technology and Legislation
VIDEO: Personalized Breast Screening and Breast Density
VIDEO: Breast Cancer Awareness - Highlights of the NCoBC 2016 Conference
Fake News: Having Dense Breast Tissue is No Big Deal
The Manic World of Social Media and Breast Cancer: Gratitude and Grief
Related Breast Imaging Content:
Single vs. Multiple Architectural Distortion on Digital Breast Tomosynthesis
Today's Mammography Advancements
Digital Breast Tomosynthesis Spot Compression Clarifies Ambiguous Findings
AI DBT Impact on Mammography Post-breast Therapy
ImageCare Centers Unveils PINK Better Mammo Service Featuring Profound AI
Radiologist Fatigue, Experience Affect Breast Imaging Call Backs
Fewer Breast Cancer Cases Between Screening Rounds with 3-D Mammography
Study Finds Racial Disparities in Access to New Mammography Technology