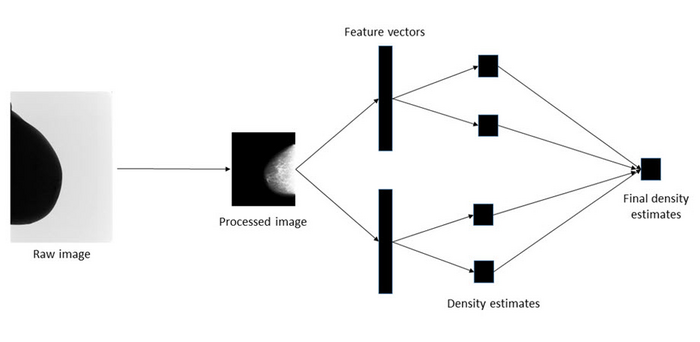
To estimate breast density, the researchers used two pre-trained deep learning models to extract features from mammograms, producing feature vectors. Each vector was then used to produce a separate density estimate using linear regression or a multi-layer-perceptron. Finally, these separate estimates were combined to produce a single prediction of breast density. Squires et al., doi 10.1117/1.JMI.10.2.024502.
April 25, 2023 — Breast cancer is the most common cancer to affect women worldwide. According to the American Cancer Society, about 1 in 8 women in the United States will develop breast cancer in their lifetime. While it is not possible to entirely prevent breast cancer, various medical organizations advise regular screening to detect and treat cases at the early stage. The breast density, defined as the proportion of fibro-glandular tissue within the breast, is often used to assess the risk of developing breast cancer. While various methods are available to estimate this measure, studies have shown that subjective assessments conducted by radiologists based on visual analogue scales are more accurate than any other method.
As expert evaluations of breast density play a crucial role in breast cancer risk assessment, developing image analysis frameworks that can automatically estimate this risk, with the same accuracy as an experienced radiologist, is highly desirable. To this end, researchers led by Prof. Susan M. Astley from the University of Manchester, United Kingdom, recently developed and tested a new deep learning-based model capable of estimating breast density with high precision. Their findings are published in the Journal of Medical Imaging.
“The advantage of the deep learning-based approach is that it enables automatic feature extraction from the data itself,” explains Astley. “This is appealing for breast density estimations since we do not completely understand why subjective expert judgments outperform other methods.”
Typically, training deep learning models for medical image analysis is a challenging task owing to limited datasets. However, the researchers managed to find a solution to this problem: instead of building the model from the ground up, they used two independent deep learning models that were initially trained on ImageNet, a non-medical imaging dataset with over a million images. This approach, known as “transfer learning,” allowed them to train the models more efficiently with fewer medical imaging data.
Using nearly 160,000 full-field digital mammogram images that were assigned density values on a visual analogue scale by experts (radiologists, advanced practitioner radiographers, and breast physicians) from 39,357 women, the researchers developed a procedure for estimating the density score for each mammogram image. The objective was to take in a mammogram image as input and churn out a density score as output.
The procedure involved preprocessing the images to make the training process computationally less intensive, extracting features from the processed images with the deep learning models, mapping the features to a set of density scores, and then combining the scores using an ensemble approach to produce a final density estimate.
With this approach, the researchers developed highly accurate models for estimating breast density and its correlation with cancer risk, while conserving the computation time and memory. “The model’s performance is comparable to those of human experts within the bounds of uncertainty,” says Astley. “Moreover, it can be trained much faster and on small datasets or subsets of the large dataset.”
Notably, the deep transfer learning framework is useful not only for estimating breast cancer risk in the absence of a radiologist but also for training other medical imaging models based on its breast tissue density estimations. This, in turn, can enable improved performance in tasks such as cancer risk prediction or image segmentation.
Read the Open Access article by S. Squires et al., “Automatic assessment of mammographic density using a deep transfer learning method,” J. Med. Imaging 10(2) 024502 (2023), doi 10.1117/1.JMI.10.2.024502.
For more information: https://spie.org/
Related Breast Density Content:
VIDEO: FDA Update on the US National Density Reporting Standard - A Discussion on the Final Rule
Creating Patient Equity: A Breast Density Legislative Update
AI Provides Accurate Breast Density Classification
VIDEO: The Impact of Breast Density Technology and Legislation
VIDEO: Personalized Breast Screening and Breast Density
VIDEO: Breast Cancer Awareness - Highlights of the NCoBC 2016 Conference
Fake News: Having Dense Breast Tissue is No Big Deal
The Manic World of Social Media and Breast Cancer: Gratitude and Grief
Related Breast Imaging Content:
Single vs. Multiple Architectural Distortion on Digital Breast Tomosynthesis
Today's Mammography Advancements
Digital Breast Tomosynthesis Spot Compression Clarifies Ambiguous Findings
AI DBT Impact on Mammography Post-breast Therapy
ImageCare Centers Unveils PINK Better Mammo Service Featuring Profound AI
Radiologist Fatigue, Experience Affect Breast Imaging Call Backs
Fewer Breast Cancer Cases Between Screening Rounds with 3-D Mammography
Study Finds Racial Disparities in Access to New Mammography Technology