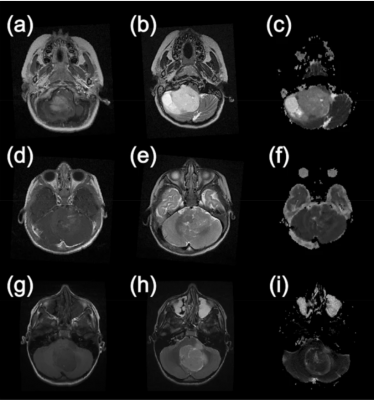
Example MR images from paediatric brain tumour patients. This first column shows T1-weighted images following the injection of gadolinium contrast agent. The second column shows T2-weighted images and the final column shows apparent diffusion coefficient maps calculated from diffusion-weighted images. (a–c) are taken from a patient with a Pilocytic Astrocytoma, (d–f) are from a patient with an Ependymoma and (g–i) were acquired from a patient with a Medulloblastoma. Image courtesy of Nature Research Journal
February 17, 2021 — Diffusion weighted imaging and machine learning can successfully classify the diagnosis and characteristics of common types of pediatric brain tumors a UK-based multi-center study, including WMG at the University of Warwick has found. This means that the tumour can be characterised and treated more efficiently.
The largest cause of death from cancer in children are brain tumors in a particular part of the brain, called the posterior fossa. However, within this area, there are three main types of brain tumor, and being able to characterize them quickly and efficiently can be challenging.
Currently a qualitative assessment of magnetic resonance imaging (MRI) by radiologists is used, however overlapping radiological characteristics can make it difficult to distinguish which type of tumor it is, without the confirmation of biopsy. In the paper, 'Classification of paediatric brain tumours by diffusion weighted imaging and machine learning', published in the journal Scientific Reports, led by the University of Birmingham including researchers from WMG, University of Warwick. The study found that tumor diagnostic classification can be improved by using non-invasive diffusion weighted imaging, when combined with machine learning (AI).
Diffusion weighted imaging involves the use of specific advanced MRI sequences, as well as software that generates images from the resulting data that uses the diffusion of water molecules to generate contrast in MR image. One can then extract an Apparent Diffusion Coefficient (ADC) map, analysed values of which can be used to tell you more about the tumor.
The study involved 117 patients from five primary treatment centers across the UK with scans from twelve different hospitals on a total of eighteen different scanners, the images from them were then analysed and region of interests were drawn by both an experienced radiologist and an expert scientist in paediatric neuroimaging. Values from the analysis of Apparent Diffusion Coeffcient maps from these images' regions have been fed to AI algorithms to successfully discriminate the three most common types of pediatric posterior fossa brain tumours, non-invasively.
Professor Theo Arvanitis, Director of the Institute of Digital Health at WMG, University of Warwick and one of the authors of the study explained:"Using AI and advance Magnetic Resonance imaging characteristics, such as Apparent Diffusion Coefficient (ADC) values from diffusion weighted images, can potentially help distinguish, in a non-invasive way, between the main three different types of pediatric tumours in the posterior fossa, the area of the brain where such tumours are most commonly found in children.
"If this advanced imaging technique, combined with AI technology, can be routinely enrolled into hospitals it means that childhood brain tumors can be characterised and classified more efficiently, and in turn means that treatments can be pursued in a quicker manner with favourable outcomes for children suffering from the disease."
Professor Andrew Peet, NIHR Professor in Clinical Paediatric Oncology at the University of Birmingham and Birmingham Children's Hospital adds:
"When a child comes to hospital with symptoms that could mean they have a brain tumour that initial scan is such a difficult time for the family and understandably they want answers as soon as possible. Here we have combined readily available scans with artificial intelligence to provide high levels of diagnostic accuracy that can start to give some answers. Previous studies using these techniques have largely been limited to single expert centres. Showing that they can work across such a large number of hospitals opens the door to many children benefitting from rapid non-invasive diagnosis of their brain tumour. These are very exciting times and we are working hard now to start making these artificial intelligence techniques widely available."
For more information: www.