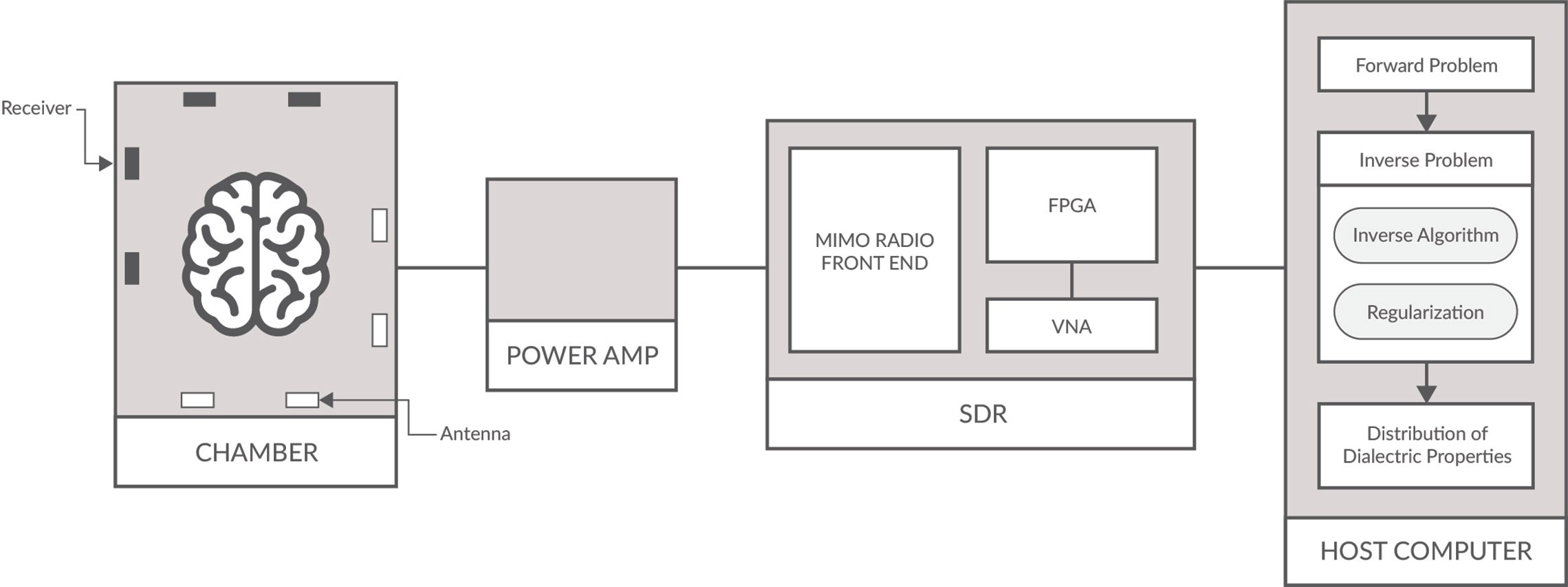
Figure 1: MWT Schematic of a typical setup for detecting malignant tissues/tumors.
While microwave tomography (MWT) imaging has been around for a few decades, advances in computing power, artificial intelligence (AI)/machine learning algorithms and various imaging algorithms have brought a resurgence of interest in MWT. This, coupled with advancements in microelectronics using microwaves, material science and embedded systems that allow for miniaturization of systems, continues to fuel interest in the field. If ongoing experiments and clinical trials prove that technology is capable of highly effective diagnosis, MWT may become an alternative to other medical imaging methods such as X-ray, magnetic resonance imaging (MRI), ultrasound and computed tomography (CT).
This technology is important for several reasons. For starters, MWT provides a means of pre-hospital diagnostics; this includes detection of tumors (particularly breast cancer and brain cancer), strokes, bone health, and proper organ functioning and pathology, which otherwise may go undetected. Moreover, the technology itself is fairly mobile compared to MRI/X-ray CT scans, which require very large and expensive equipment only found in hospitals and which radiologists must operate the equipment. Mobile services such as the one developed by Islam et al.1 have been in the works. Other benefits stem from the fact that MWT uses non-ionizing radiation, which has resulted in less health risks to patients, low operational costs and ease of use (one does not need to be a radiologist to use such devices).
There is an increasingly urgent need for an efficient and reliable technique to detect the earlier signs of cancer, particularly as cancer rates continue to increase. Current imaging systems, such as X-ray mammography, CT scan, ultrasound and MRI are often used to detect cancers. However, these techniques have been known for false positives (many biopsies performed are unnecessary) and missed detection, low-resolution scans, uncomfortable for patients, under-performing for the deep-lying or solid tumors, as well as being a time-consuming process until diagnosis.
How MWT Works
The physics behind MWT is that it exploits tissue-dependent dielectric (i.e., the relative permittivity and conductivity) contrast to reconstruct signals and images using tomographic imaging techniques. Tomography stems from the Ancient Greek word tomos, meaning "slice/section" and graph "to write." The basic mechanism behind microwave tomography imaging is to stack slices or cross sections of images generated from the dielectric contrast between healthy tissues and lesions/malignancies of scattered microwaves. These microwaves tend to be in the range of hundreds of megahertz to tens of gigahertz. In MWT applications, a map or an image either in 2-D or 3-D is formed that shows different tissues’ electrical properties (dielectric properties) or the spatial distribution of a strong scatterer, such as a tumor inside the body.
The scattering process involves three steps. First, an external source generates an incident wave to propagate toward the scatterer/malignant tissue. Second, there is a microwave-matter interaction, where contrast current is induced in response to the incident EM field. Finally, reradiation, where induced contrast current radiates, which generates scattered fields that are collected at receivers before being processed to reconstruct an image. The presence of different dielectrics in the imaged area has an effect on the propagation of the microwaves. A malignant tissue usually has different electrical properties than the surrounding normal and healthy tissues, which can be differentiated in the image.
The hardware of such a MWT system (see Figure 1) generally consists of a host machine that controls one or several microwave signal transmitters and receivers, generally a vector network analyzer, an antenna array and a radio-frequency switch that switches between different antennas. The body or specimen to be imaged is surrounded by a number of transmit and receive antennas (sometimes making up a chamber for breast or brain imaging), which retrieve the spatial distribution of the dielectric parameters. Each transmitter antenna emits onto the body microwave signals for a duration of time and the scattered signal by the body is collected by all the receivers.
There are two main ways to approach the reception of the scattered microwaves using the host machines image reconstruction algorithms. Qualitative imaging algorithms use radar like techniques for image generation to differentiate the malignant tissues from the normal tissues as the malignant tissues are usually stronger scatterers than normal tissues. Various radar imaging algorithms can then be used to focus the tumor, such as confocal microwave imaging, beamforming and tissue sensing adaptive radar. In these techniques, an ultra-wideband signal is used to have accurate time domain resolution. On the other hand, quantitative imaging algorithms (i.e., for microwave tomography discussed in this article) give a map of the distribution of the various tissues with the values of electrical properties in the body. These algorithms are usually based on the inverse scattering problem (ISP); a problem formulated as partial differential equations and much studied in mathematics and physics.
Challenges Faced in the field of MWT and Current Research
Several commercial and industrial applications exist in microwave imaging, including airport security scanners which are able to scan for metallic materials such as weapons, as well as industrial applications such as underground imaging to find buried objects. Even so, the challenge in the medical field is that these tissues are living, and often hard to characterize dielectrically due to the complex nature of the cellular structure as well as various layers of different cells/proteins. The presence of multiple tissues with different properties results in a complex scattering environment which must be detected. Matters are made more challenging due to the area being imaged to be in the near-field; meaning, the distance between the receiver/antenna to the object being studied is of the range of less than one wavelength to several wavelengths. Applications of near field MWT imaging include breast and brain cancer detection.
When considering the radio receiver/transmitter, a number of issues need to be considered. This includes a very high dynamic range in order to successfully measure weak scattering fields among the noise floor. As well, the microwave power must be efficiently coupled to biological tissues, and there must be a proper frequency selection for specific penetration depth. The choice of frequency band is generally a trade-off between penetration depth and imaging resolution. A higher frequency can result in a better resolution, but experience greater attenuation when penetrating to human tissues, which are very lossy. Moreover, the radio equipment used in experiments and clinical trials can be economically challenging. For instance, Vector network analyzers (VNAs) are very expensive but often required to obtain the multichannel phase coherent microwave signals needed for MWT based medical imaging.
Challenges related to computation are numerous. Various imaging reconstruction algorithms exist, and show various levels of accuracy in detection in research studies on breast/brain cancer and stroke detection. However, it is still very difficult to create 3-D computationally efficient imaging algorithms which can model the effect of multiple antennas and a receiver feeding network for a large body where near-field scattering is measured. MWT image reconstruction systems are sensitive to the quality of received signals, such as temperature drift of measuring devices or changes in permittivity of body due to temperature that can occur during scattering measurement. Since imaging is based on the contrast between normal and malignant tissue, in terms of dielectric properties, the contrast may be difficult to differentiate and therefore complicate the imaging problem. This is what image reconstruction algorithms implementing ISPs must take into account. ISPs are concerned with determining the environment of an unknown scattering distribution, such as the shape, position, dielectric properties/permittivity or thermal properties, from the measure of scattered EM fields. These problems are notoriously difficult to solve due to being ill-posed and nonlinear. Many of the algorithms used to solve ISPs are based on the Born approximation, including the popular distorted Born Iterative Method (DBIM) used to solve nonlinear EM ISPs iteratively to reconstruct the spatial distribution of dielectric properties of the scatterer within a region V (i.e., the chamber). See Figure 2.
Current research in the field of MWT for biological studies includes using in-viro clinical experiments, as well as tissue-mimicking phantom design, fabrication and material characterization. Proof-of-concept designs include portable microwave head imaging systems,2 which are capable of detecting strokes, tumors as well as hemorrhages. In recent years, advances in AI and machine learning, particularly in the field of neural networks and machine learning, are allowing for breast cancer imaging/scanning in near real time. Deep learning has demonstrated promise for solving and/or improving solutions to the inverse scattering problem in medical imaging. Moreover, the use of neural networks to improve tissue classification in 2-D images has gathered stream. Many models in machine learning use prior data to train classification models for such things are dielectric constants of different tissues and other surrounding or background mediums/materials. This is not ideal, however, since for clinical trials of MWT to be shown effective, these will need to recovered from the scattered field data itself and taken into account.
One such experiment demonstrating the power of deep learning on breast cancer detection is from Edwards et al.3 The researchers found that by using a two-stage process, they are able to predict “the bulk parameters of the fibro glandular region within the breast and use that as prior information for a 3-D inversion of the field data to reconstruct regions of high complex-valued permittivity compared to some known tissue background within the breast.” Although current research is able to demonstrate proof-of-concept for detecting malignancies, tumors and other abnormalities, a great deal of work is yet to be done before MWT for medical imaging can be found in the marketplace and in hospitals.
The Use of Software Defined Radio (SDR) for MWT
A software defined radio (SDR) consists primarily of a radio front end and digital backend. The radio front end contains the receive (Rx) and transmit (Tx) functionality to receive signals, with the highest bandwidth SDRs having a being between 0-18 GHz. The SDRs digital back-end contains an FGPA with on-board DSP capabilities for various filtering, modulation, demodulation, upconverting and down converting of carrier wave signals. See Figure 3.
Significant advances in software defined radio (SDR) as an embedded system has made them appealing for medical researchers in MWT. The main appeal is that commercial off-the-shelf SDRs can be easily implemented to collect the imaging data, which reduces development time and overall cost while increasing portability; one of the benefits that MWT may bring to medicine. High performance SDRs also provide multiple input multiple output (MIMO) phase coherent and RF shielded cross-channel isolation needed for MWT imaging systems. This is especially relevant for breast and brain MWT imaging experiments and clinical trials which require many antenna/receivers.
SDRs are flexible compared to traditional hardware-based radio devices, which are limiting for cross functionality and face the problem that they can only be modified through physical hardware changes. SDRs provide the capability of adaptable software operation and reprogrammable FPGAs for waveform generation, adaptive gain, error correction, frequency hopping, switching and other functionalities needed for MWT. As well, FPGAs enable researchers to test and simulate new MWT imaging techniques and algorithms, and also types of optimization and calibration schemes. They can also function as a VNA, therefore reducing the costs of development. Lastly, SDRs with very high data throughput allows for the transmission of vast amounts of scattering measurements from antenna array to be sent to host system where image reconstruction occurs.
Brendon McHugh has a degree in theoretical and mathematical physics from the University of Toronto and works at Per Vices as a Field Applications Engineer and Technical Writer. Per Vices Corporation is an industry leader in developing & deploying high performance, high-bandwidth software defined radio platforms to support a wide variety of applications within defense, civil, aerospace, medical, telecommunications, broadcasting and wireless management industries.
References:
- https://www.nature.com/articles/s41598-019-51620-z#Sec1. Accessed Aug. 13, 2021.
- https://www.researchgate.net/publication/331334839_Portable_Microwave_Head_Imaging_System_Using_Software-Defined_Radio_and_Switching_Network. Accessed Aug. 13, 2021.
- https://arxiv.org/pdf/1909.11175.pdf. Accessed Aug. 13, 2021.
General References:
https://www.mdpi.com/2079-9292/10/6/674. Accessed Aug. 13, 2021.
https://www.jpier.org/PIER/pier167/07.20030705.pdf. Accessed Aug. 13, 2021.
https://www.hindawi.com/journals/ijap/2019/4074862/. Accessed Aug. 13, 2021.
https://ieeexplore.ieee.org/abstract/document/8739478. Accessed Aug. 13, 2021.