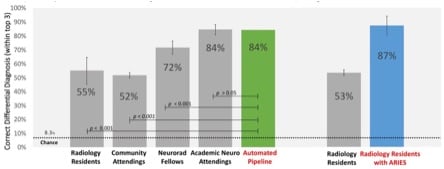
Smart software improved the performance of resident radiologists to the level of academic experts in neuroradiology, according to research presented at the 2019 meeting of the Society of Imaging Informatics in Medicine (SIIM). Even on its own, the automated system, called ARIES (Adaptive Radiology Interpretive Education System), outperformed groups of radiologists who are not experts in neurology.
The presenter of data indicating the clinical power of this software advised against using artificial intelligence in place of radiologists. ARIES or similar technology could, however, improve the efficiency and accuracy of radiological decision-making, according to Jeffrey D. Rudie, M.D., Ph.D., a radiology resident at the University of Pennsylvania. This kind of technology, he said, has the potential to help radiologists meet the rising complexity and volume of medical imaging.
The ARIES automated system, which quantitatively characterizes brain MRIs, was designed to support diagnosis, prognosis and scientific discovery, according to Rudie and colleagues. According to data that Rudie presented June 28 during a SIIM session focused on machine learning technologies, ARIES performed at the level of academic neuroradiologists in diagnosing complex MRIs for 35 rare and common brain diseases.
Test Results Demonstrate Clinical Power
Test data, presented as part of Rudie’s talk, titled “Deep Learning and Bayesian Inference System for Automated Brain MR Diagnosis Performs at Level of Academic Neuroradiologists and Augments Resident Performance,” showed that, when used as an interactive clinical decision support tool, the system augmented the performance of radiology residents to the level of academic neuroradiologists.
When its results were compared to those of different groups of radiologists, ARIES achieved the same accuracy (84 percent) as academic neuro attendings. The system substantially outperformed community radiologists who were right only 52 percent of the time and radiology residents who — on their own — achieved 55 percent accuracy. (With ARIES help, the accuracy of a subgroup of radiology residents soared to 87 percent.)
Based on these tests, the greatest impact from this type of technology, Rudie concluded, may be to augment the diagnostic skills of radiologists with limited experience.
How ARIES Works
The automated system, which Rudie framed as a “proof of concept system,” mirrors the perceptual and cognitive steps of image interpretation. He described these as the “two fundamental steps” that people are trained to take when interpreting medical images.
The logic of the automated system comes from a combination of convolutional neural networks, image processing, and Bayesian networks, Rudie said. Abnormal signal features and spatial and anatomic subregion features derived from atlas-based images were combined with clinical information such as patient age, gender and immune status.
Despite ARIES’ stellar solo performance, Rudie insisted at the SIIM meeting that the system not be viewed as better than radiologists. Instead, it should be seen as an aide — its machine intelligence complementing that of people.
To support this contention, Rudie cited the results achieved by pairing ARIES with a subset of radiology residents. In the test, ARIES boosted the decision-making of radiology residents to 87 percent accuracy (compared to 53 percent when the subgroup of radiology residents were separately tested.)
Rudie and colleagues concluded that AI-based clinical decision support technology, such as ARIES, could significantly improve the accuracy and efficiency of image interpretation.
Greg Freiherr is a contributing editor to Imaging Technology News (ITN). Over the past three decades, he has served as business and technology editor for publications in medical imaging, as well as consulted for vendors, professional organizations, academia, and financial institutions.
Editor’s note: This article is the tenth and final piece in a content series by Greg Freiherr covering the Society for Imaging Informatics in Medicine (SIIM) conference in June.
Related content:
Are Shallow Networks Better Than Deep Ones?
How AI Might Provide a Safety Net for Patients and Providers
Smart Algorithm Extracts Data from Radiology Reports
PODCAST: Why Blockchain Matters In Medical Imaging
PODCAST: How to Fix Your Enterprise Imaging Network
PODCAST: 5 Low-Cost Ways To Slow Hackers