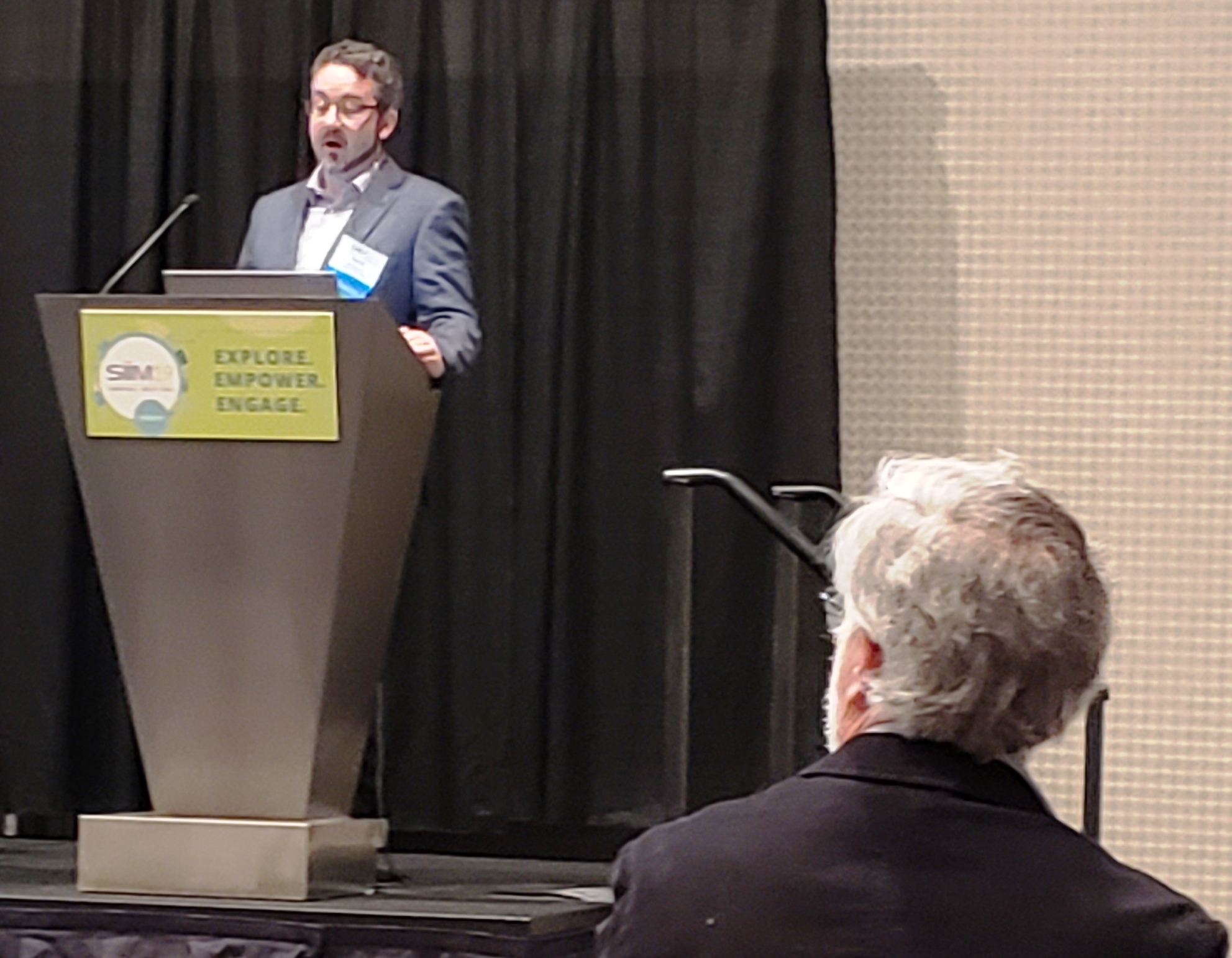
David Anderson presents information about the development of artificial Intelligence (AI) at SIIM 2019 as his father, Charles, looks on from a front row seat. The father-son team presented information at the SIIM annual meeting about their use of relatively shallow Deep Learning networks to analyze chest radiographs with AI.
Two simple neural networks are better than one complex one, according to a father-son team of entrepreneurs. On June 27 the two described “A Two-Stage Deep Learning Approach to Chest X-Ray Analysis” during the 2019 meeting of the Society of Imaging Informatics in Medicine in Denver.
Diagnostic decisions coming from models built using two “shallow” neural networks can lead to faster, more accurate and more interpretable artificial intelligence for radiography and other imaging modalities, according to David Anderson.
“When you have a literal deep (learning) network you have lots and lots of computational nodes and lots of layers of those nodes. A shallower network means that there are fewer computations so it is less math going on, which means you can look inside of it and see what it is doing — you can pull it apart,” Anderson told Imaging Technology News after the SIIM presentation.
He and his father, Charles, described their work at SIIM 2019 as a tag team — David presenting technical information first; Charles Anderson following with broader perspectives. Both men work at privately held Pattern Exploration, where Charles Anderson, Ph.D., is CEO; David is the machine learning developer.
David cited research1 & 2 into the automated analysis of chest X-ray images as the basis for the work done at Pattern Exploration. The use of “shallow” networks allows training with unlabelled data, which makes training less difficult and more efficient, he said. The use of these networks can also make the logic underlying AI less opaque.
Making the “Box” Transparent
Many have described deep learning as leading to algorithms that are “black boxes.” If shallower networks are used, however, the result “is not black at all — if you have the right tools,” David Anderson told ITN. “You can say, ‘Here is what this network is doing.’”
The two, five-layer networks that the Andersons developed to model X-ray analysis represent deep learning despite their relatively shallow depth. (Deep learning is a subset of machine learning, which is a type of artificial intelligence.) These two networks have separate tasks. One aligns the images into a standard orientation so the patient spine, for example, is presented vertically. The other does the classification.
Charles, who also serves as a professor of computer science at Colorado State University, focused on the potential impact of the research. He also raised the potential utility of a verbal interface. The computer science professor described during his presentation how such a verbal interface might be used. “It would allow you to ask a quick question — like you do with Siri — and come back with an answer in a spoken language,” he summarized later for ITN.
Supporting verbal Q & A would allow the radiologist to focus on the screen, rather than intermittently shifting attention to input devices. Radiologists have told Charles that their priorities are speed and confidence that their decisions are correct.
Greg Freiherr is a contributing editor to Imaging Technology News (ITN). Over the past three decades, he has served as business and technology editor for publications in medical imaging, as well as consulted for vendors, professional organizations, academia, and financial institutions.
Related content and references:
1. P. Rajpurkar, et al. (2017) CheXNet: Radiologist-Level Pneumonia Detection on Chest X-Rays with Deep Learning. https://arxiv.org/abs/1711.05225v3
2 I. M. Baltruschat, et al. (2018) Comparison of Deep Learning Approaches for Multi-Label Chest X-Ray Classification. https://arxiv.org/abs/1803.02315v1
How AI Might Provide a Safety Net for Patients and Providers
Editor’s note: This article is the ninth piece in a content series by Greg Freiherr covering the Society for Imaging Informatics in Medicine (SIIM) conference in June.
Related content:
How AI Might Provide a Safety Net for Patients and Providers
Smart Algorithm Extracts Data from Radiology Reports
PODCAST: Why Blockchain Matters In Medical Imaging
PODCAST: How to Fix Your Enterprise Imaging Network
PODCAST: 5 Low-Cost Ways To Slow Hackers