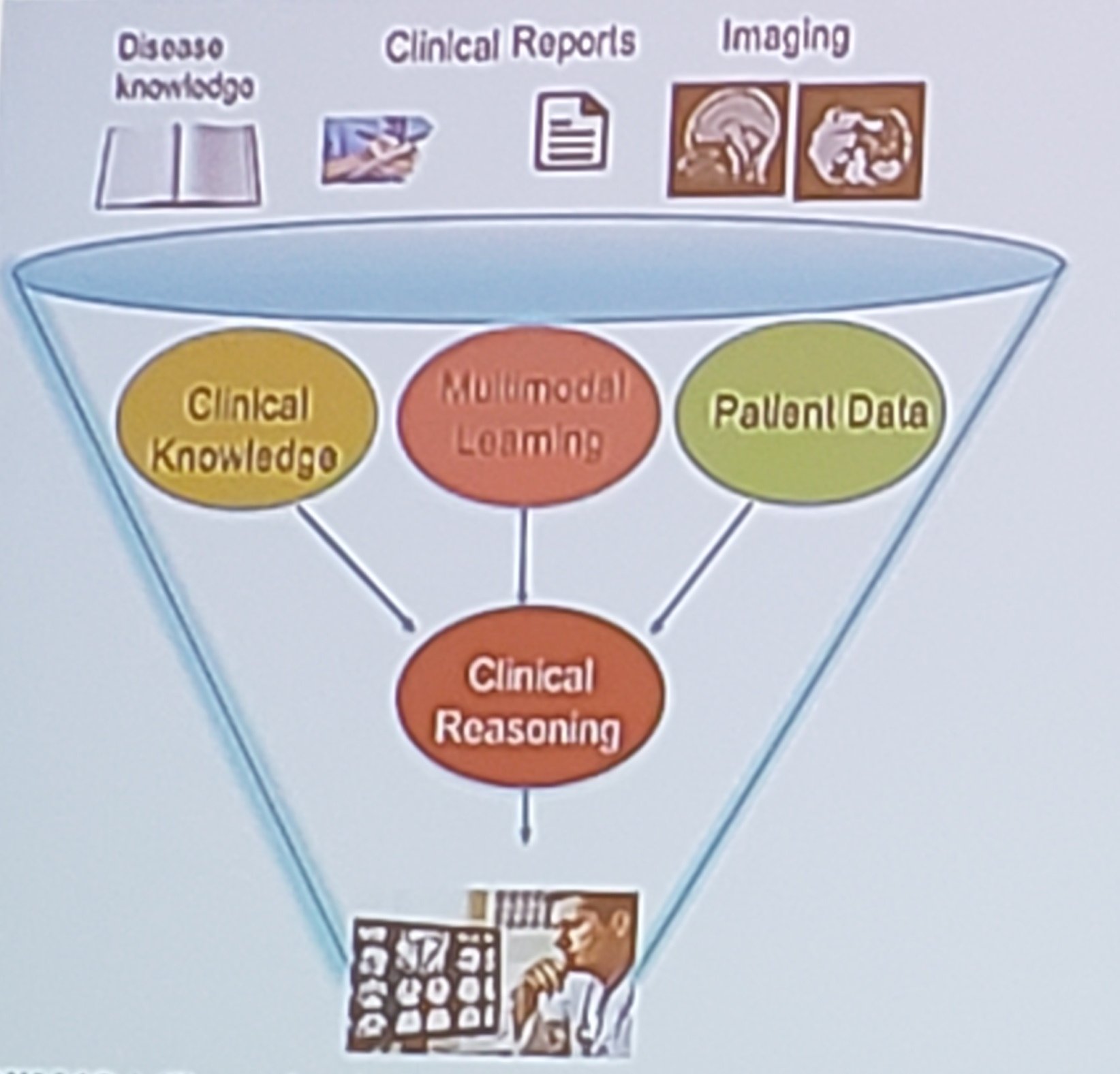
Radiology reports may contain information essential to figuring out a patient’s condition. But this information is often locked in free text, which can make it difficult to search, analyze and summarize report data. That’s where natural language processing (NLP) might come in.
On June 26, 2019, IBM researcher Ashutosh Jadhav, Ph.D., described at the annual meeting of the Society for Imaging Informatics in Medicine (SIIM) in Denver an algorithm that uses artificial intelligence to extract information from the free text of radiology reports.
“There is a lot of information hidden in these reports,” Jadhav told ITN after his presentation during the Natural Language Processing session. Included in that information are things like the physician’s findings. The extracted data might be used to automatically populate data fields in PACS.
Automatic structuring of radiology reports could unleash the power of information otherwise locked in these reports, he told an audience attending his presentation titled “Automatic Extraction of Structured Radiology Reports.” Jadhav and his colleagues are working to further improve the prototype, he said, noting that he cannot predict if or when the algorithm will be productized. “Our work is majorly focused on the research aspect,” he told ITN after his presentation. Jadhav is a research staff member at IBM Research Almaden, IBM's Silicon Valley innovation lab.
Extensive Research
In developing the NLP algorithm, Jadhav and colleagues had to overcome challenges including missing section labels, inconsistent section ordering, and inconsistent section formatting. Differences among institutions and individual radiologists should not be issues, he told ITN, because “we have a really big sample size and we are processing reports from multiple institutes.”
Radiology reports from more than 200,000 chest X-rays were used to develop the algorithm. These reports were randomly split into training and testing data sets. The training data set, comprised of about 80 percent of these reports, was used to develop NLP rules. The remaining 20 percent comprised the data set used for testing. Some 200 reports, randomly selected from the testing set, were used to determine how well the algorithm identified sections and corresponding section texts.
Greg Freiherr is a contributing editor to Imaging Technology News (ITN). Over the past three decades, he has served as business and technology editor for publications in medical imaging, as well as consulted for vendors, professional organizations, academia, and financial institutions.
Editor’s note: This article is the seventh piece in a content series by Greg Freiherr covering the Society for Imaging Informatics in Medicine (SIIM) conference in June.
Related content:
Why Blockchain Matters In Medical Imaging
DeepAAA Uses AI to Look Automatically For Aneurysms
Making AI Safe, Effective and Humane for Imaging
5 Low-Cost Ways To Slow Hackers
Imaging on Verge of Game-changing Transformation
How to Fix Your Enterprise Imaging Network
Imaging on Verge of Game-changing Transformation
VIDEO: AI That Second Reads Radiology Reports and Deals With Incidental Findings
AI Detects Unsuspected Lung Cancer in Radiology Reports, Augments Clinical Follow-up