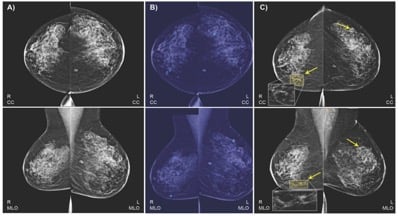
IBM collected a dataset of 52,936 images from 13,234 women who underwent at least one mammogram between 2013 and 2017, and who had health records for at least one year prior to the mammogram. The algorithm was trained on 9,611 mammograms. Image courtesy of Radiology.
July 19, 2019 — Breast cancer is the global leading cause of cancer-related deaths in women, and the most commonly diagnosed cancer among women across the world.1 From our perspective, improved treatment options and earlier detection could have a positive impact on decreasing mortality, as this could offer more options for successful intervention and therapies when the disease is still in its early stages.
Our team of IBM researchers published research in Radiology around a new artificial intelligence (AI) model that can predict the development of malignant breast cancer in patients within the year, at rates comparable to human radiologists. As the first algorithm of its kind to learn and make decisions from both imaging data and a comprehensive patient’s health history, our model was able to correctly predict the development of breast cancer in 87 percent of the cases it analyzed, and was also able to correctly interpret 77 percent of non-cancerous cases.
Our model could one day help radiologists to confirm or deny positive breast cancer cases. While false positives can cause an enormous amount of undue stress and anxiety, false negatives can often hamper how early a cancer is detected and subsequently treated.
When put to the test against 71 different cases that radiologists had originally determined as “non-malignant,” but who ultimately ended up being diagnosed with breast cancer within the year, our AI system was able to correctly identify breast cancer in 48 percent of individuals (48 percent of the 71 cases) – which otherwise would not have been flagged.
Breast Cancer Screening Today
Currently, digital mammography is the main imaging method of screening. Women typically undergo breast mammography every 1-2 years, depending on their familial history. The exam is then interpreted by radiologists who examine the images for the existence of a malignant finding.
Analyzing mammograms is a challenging task. The differences between lesions and background could be very subtle: There are multiple types of possible findings which differ from each other in shape, size, color, texture and other factors. A second reading of mammograms by an additional radiologist has been proven to increase sensitivity and specificity (2). However, a lack of trained radiologists and time limitations often makes it difficult to incorporate second readers as part of the standard screening procedure in many countries (3).
To help close the gap of readily available “second readers” and aid radiologists in their analyses, more and more systems are turning to computational models based on deep neural networks to analyze healthcare data.
Turning to AI to Improve Breast Cancer Prediction
Our team at IBM Research – Haifa hypothesized that a model combining machine learning and deep learning could be applied to assess breast cancer at a level both comparable to radiologists and with the capabilities to be accepted into clinical practice as a second reader.
Working with one of Israel’s largest healthcare organizations, we were able to work with clinically-collected, de-identified mammography images, which were also linked to holistic clinical data and biomarkers regarding each patient, such as thyroid function, reproductive history and other information.
Using this information, our team was able to create a unique and novel algorithm that is – to our knowledge – potentially the first to incorporate both mammograms and comprehensive electronic health record (EHR) data for the prediction of breast cancer. Built on deep learning models, our team was able to train this system to achieve an accuracy comparable to radiologists, as defined by the American benchmark for screening digital mammography.
Additionally, this model mapped connections between additional clinical risk features, such as iron deficiency, white blood cell profiles, metabolic syndrome and thyroid function, and was able to help reduce the likelihood of breast cancer misdiagnosis among the cases studied. We plan to continue analyzing these clinical risk elements to better understand their impact and connections to an individual’s personalized risk.
Our machine learning algorithms were successful due in large part to the wealth of data on which they were trained. Through IBM research partners Maccabi Health Services and Assuta Medical Center, two large health providers in Israel, our team was able to obtain a large set of de-identified, voluntarily collected mammography images that were also linked to rich and detailed record of the corresponding individual’s clinical data – such as a history of any cancer diagnoses, pregnancy history and status of menopause.
Additionally, this data also included follow-up from biopsies, cancer registry data, lab results, and codes for various other procedures and diagnoses. This astonishing amount of data provided a deep pool of information from which our machine learning models could learn, and allowed these algorithms to connect patterns and trends that may not have been possible otherwise.
Why It’s Not All That Simple
One of the largest challenges we faced in training our models was in combining the data from three different origins. Aside from the different formats, clinical data is often noisy or missing values. Additionally, we wanted to emulate the typical decision-making process of a radiologist into the algorithms.
For example, we wanted to use the two standard views, bilateral craniocaudal (CC) and mediolateral oblique (MLO) views, which may comprise routine screening mammography. In standard practice globally, radiologists typically compare these two views when assessing a lesion, and we wanted our model to work that way as well.
In this retrospective study, we collected a dataset of 52,936 images from 13,234 women who underwent at least one mammogram between 2013 and 2017, and who had health records for at least one year prior to the mammogram. We trained our algorithm on 9,611 mammograms and health records of women, with two objectives: to predict biopsy malignancy and differentiate normal from abnormal screening examinations.
We created a combined machine- and deep-learning model for those objectives, using as input the mammography’s standard four-view images and the detailed clinical histories.
We started by identifying a subset of the clinical features with the highest contribution to positive biopsy prediction. These were fused into a deep neural network (DNN) that was trained on each mammogram for each prediction task. Using the DNN, we extracted the probability of each prediction task, as well as imaging features, for each view. Finally, we concatenated the imaging features from both views as well as the entire set of clinical features into a single representation of a patient’s breast. The final probability for either cancer-positive biopsy or normal/abnormal differentiation were estimated using gradient boosting machines model.
Good Results and Some Surprises
Our model obtained an area under the receiver operating curve (AUROC) of 0.91, and specificity of 77.3 percent at a sensitivity of percent. The addition of clinical data to the mammograms has significantly increased the model’s AUROC and sensitivity.
When based on clinical data alone, our model obtained an AUROC of 0.78, improving breast cancer risk prediction in comparison to common risk models like Gail model. Moreover, we were able to identify clinical factors that may possibly contribute to elevated risk and which were not previously used by other models, such as white blood cell profiles and thyroid function tests.
More accurate prediction could hold the potential to reduce the number of women being sent for unnecessary tests – or experiencing the trauma of being needlessly assigned as high risk – by traditional models. Combining clinical data with imaging information could offer the potential to more accurately validate initial results.
Sources
1. Society AC. Global Cancer Facts & Figures. 3rd Edition. Atlanta: American Cancer Society; 2015.
2. https://pubs.rsna.org/doi/full/10.1148/radiol.2018171010
3. Leivo T, Salminen T, Sintonen H et al. Incremental cost-effectiveness of double-reading mammograms. Breast Cancer Res Treat 1999;54(3):261–267
Reference
Radiology, Predicting Breast Cancer by Applying Deep Learning to Linked Health Records and Mammography Images, Ayelet Akselrod-Ballin*, Michal Chorev* , Yoel Shoshan, Adam Spiro, Alon Hazan, Roie Melamed, Ella Barkan, Esma Herzel, Shaked Naor, Ehud Karavani, Gideon Koren, Yaara Goldschmidt, Varda Shalev, Michal Rosen-Zvi, Michal Guindy
Michal Chorev is a research staff member at IBM Research.