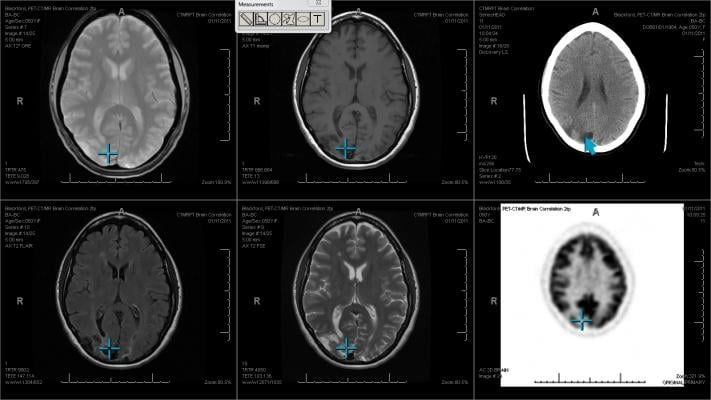
January 31, 2018 — Researchers used machine learning techniques, including natural language processing algorithms, to identify clinical concepts in radiologist reports for computed tomography (CT) scans, according to a new study. The study was conducted at the Icahn School of Medicine at Mount Sinai and published in the journal Radiology. The technology is an important first step in the development of artificial intelligence (AI) that could interpret scans and diagnose conditions.
From an ATM reading handwriting on a check to Facebook suggesting a photo tag for a friend, computer vision powered by artificial intelligence is increasingly common in daily life. AI could one day help radiologists interpret X-rays, CT scans and magnetic resonance imaging (MRI) studies. But for the technology to be effective in the medical arena, computer software must be taught the difference between a normal study and abnormal findings.
This study aimed to train this technology how to understand text reports written by radiologists. Researchers created a series of algorithms to teach the computer clusters of phrases. Examples of terminology included words like phospholipid, heartburn and colonoscopy.
Researchers trained the computer software using 96,303 radiologist reports associated with head CT scans performed at The Mount Sinai Hospital and Mount Sinai Queens between 2010 and 2016. To characterize the “lexical complexity” of radiologist reports, researchers calculated metrics that reflected the variety of language used in these reports and compared these to other large collections of text: thousands of books, Reuters news stories, inpatient physician notes and Amazon product reviews.
“The language used in radiology has a natural structure, which makes it amenable to machine learning,” said senior author Eric Oermann, M.D., instructor in the Department of Neurosurgery at the Icahn School of Medicine at Mount Sinai. “Machine learning models built upon massive radiological text datasets can facilitate the training of future artificial intelligence-based systems for analyzing radiological images.”
Deep learning describes a subcategory of machine learning that uses multiple layers of neural networks (computer systems that learn progressively) to perform inference, requiring large amounts of training data to achieve high accuracy. Techniques used in this study led to an accuracy of 91 percent, demonstrating that it is possible to automatically identify concepts in text from the complex domain of radiology.
"The ultimate goal is to create algorithms that help doctors accurately diagnose patients,” said first author John Zech, a medical student at the Icahn School of Medicine at Mount Sinai. “Deep learning has many potential applications in radiology — triaging to identify studies that require immediate evaluation, flagging abnormal parts of cross-sectional imaging for further review, characterizing masses concerning for malignancy — and those applications will require many labeled training examples."
“Research like this turns big data into useful data and is the critical first step in harnessing the power of artificial intelligence to help patients,” said study co-author Joshua Bederson, M.D., professor and system chair for the Department of Neurosurgery at Mount Sinai Health System and clinical director of the Neurosurgery Simulation Core.
Researchers at Boston University and Verily Life Sciences collaborated on the study.
For more information: www.mountsinai.org