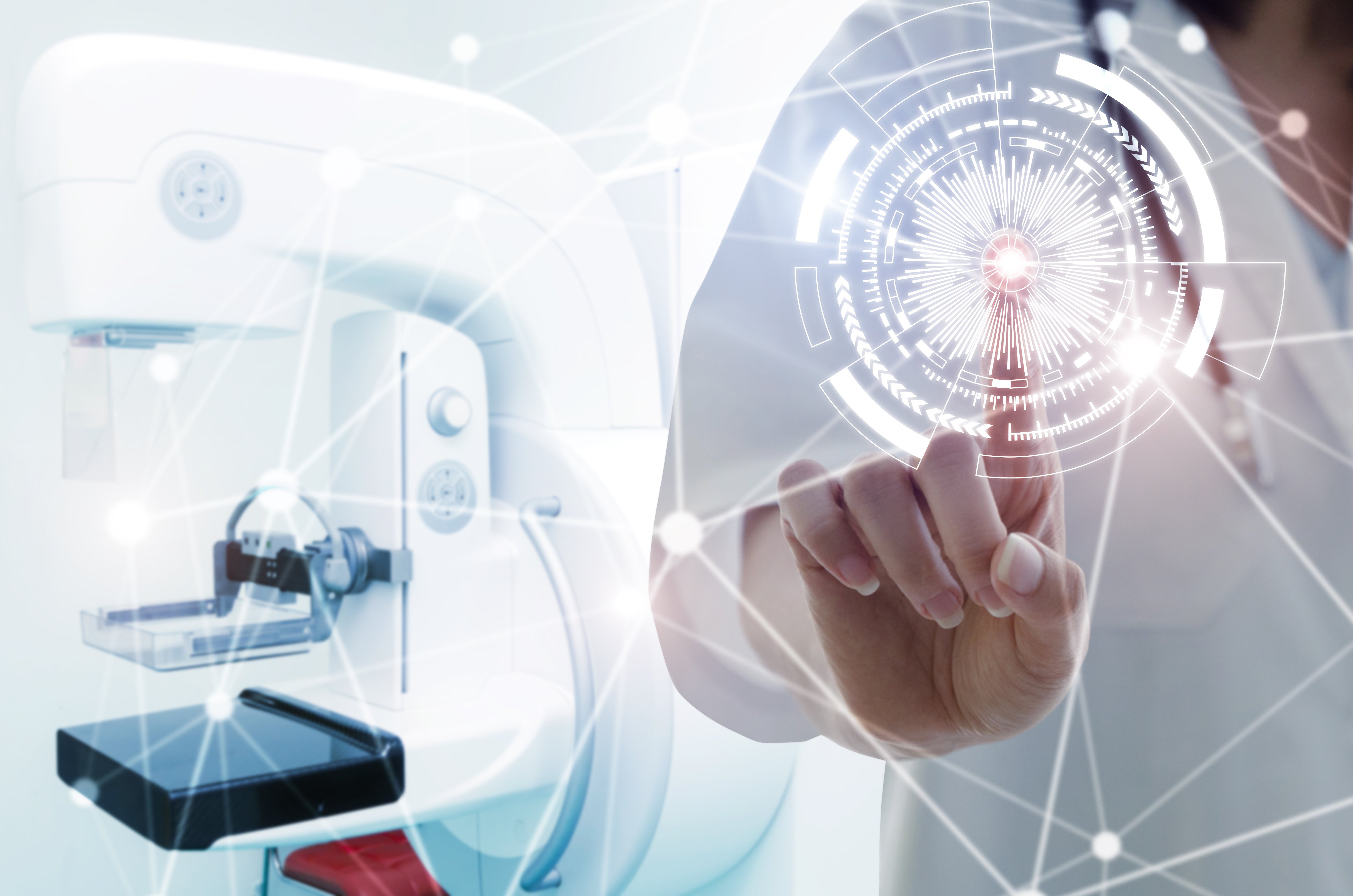
Women in the United States have a 1 in 8 (or about 13 percent) lifetime risk of being diagnosed with breast cancer during their lifetime. Death rates from breast cancer have been decreasing since 1989, and these decreases are thought to be the result of treatment advances, earlier detection through screening and increased awareness.1 The health community continues to strive for early detection of lesions — primarily through screening mammography, which is the most effective method for detecting early breast cancers and has been shown to reduce breast cancer-specific mortality.
Mammography imaging has evolved from single emulsion film, to a 2-D digital format, and finally to the multi-image platform digital breast tomosynthesis (DBT). Throughout this evolution, image quality has increased, and radiologists have been able to identify cancers more readily.
The introduction of DBT in 2011 has shown to decrease call-back rates while increasing the number of found cancers.2 However, DBT produces many more images for radiologists to interpret, and has significantly increased reading times. Radiologists who specialize in reading mammography studies are struggling to read the same number of studies despite the dramatic increase in the number of images presented for interpretation.
Most recently, artificial intelligence (AI) and machine learning (ML) have arrived and show great promise in increasing the accuracy of screening mammographic procedures and decreasing reading times. Applications in AI-driven computer-aided diagnosis (CAD), detection algorithms and breast ultrasound (US) are technologies to watch for in this important and evolving field.
Computer-aided Detection Versus Computer-aided Diagnosis Using AI
As early as 1988, radiologists were early adopters of using computers to automate detection of abnormalities. Traditional CAD algorithms are mathematical models that can determine whether an imaging feature associated with a disease is present.
For example, traditional CAD algorithms may be able to detect calcifications on mammograms but cannot differentiate between benign calcifications and those seen in the setting of ductal carcinoma in situ (DCIS), a non-invasive form of breast cancer. These early CAD systems were FDA approved for 2-dimensional images and provided a detection marking to the interpreting radiologist on the four images presented with each screening study.
The introduction of DBT presented radiologists with a significantly larger data set to review and assess for breast cancer. A screening DBT study contains the four traditional breast images, and also includes a tomo “sweep” of each breast. The total number of images obtained is dependent upon the thickness of the breast and slice thickness of each image. While the traditional CAD system could still be utilized on the traditional images, it could not assess the tomographic series.
Within the past 14 months, the U.S. Food and Drug Administration (FDA) approval for a CAD system specific to DBT has been obtained. Historically, CAD algorithms were hand-crafted and based on consultations with radiologists and could therefore be biased toward human thought processes. The increase in processing power available today has led to the ability to develop a data-driven and methodical machine learning process as opposed to a rule-based, problem-specific approach. Unlike traditional CAD algorithms, the AI approach has the potential to detect useful features and relationships that are either imperceptible to humans or are currently unknown.3 Using machine learning, this technology has the capacity to adapt and evolve with exposure to more data points, which allows for algorithms to identify discerning features that are best predictive of outcomes independently. As well, it allows the system to become more accurate over time and adapt to any changes in treatment protocols, disease profiles and patient characteristics.
This AI-driven CAD system has shown a marked (up to 50 percent) reduction in radiologists reading time and an improvement of cancer detection rates, coupled with lower unnecessary recall rates.
AI Detection Algorithms
The tech giants have also entered the field using an AI model that can automatically detect breast cancer. In a recently published article, a study working with data sets from the U.S. and the United Kingdom (U.K.) showed the AI model was able to more effectively screen for breast cancer using only the pixel data provided (no access to patient history or risk factors).
Over 42 million exams are performed each year between the U.S. and the U.K. Despite the widespread adoption of mammography, interpretation of images remains challenging. Traditional CAD systems, and even early AI studies, have shown little evidence of the ability of AI systems to translate between different screening populations and settings without additional training data. However, in this study, evidence demonstrating the ability of the system to generalize from the U.K. to the U.S. data sets was provided, and the algorithm proved capable of surpassing human experts in its ability to predict breast cancer. Potential clinical applications for this platform could be used to reduce the workload involved in the double-reading process that is used in the UK, while still preserving — or even augmenting — the standard of care.4
Breast MRI
Breast MRI and breast US are two modalities used either in conjunction with traditional screening mammography, or as an initial screening tool for young women with increased risk factors for developing breast cancer. Because neither of these technologies utilize ionizing radiation to produce images, they can be preferable for women who are pregnant or are in childbearing years. Research was presented in 2019 that demonstrated the successful use of advanced algorithms on breast MRI where researchers provided MRI images to a neural network, which is a collection of computer-based algorithms that have the capacity to autonomously recognize patterns and relationships within an underlying dataset in a way that mimics human thought and learning processes. Using this technology, computers were able to analyze and identify the presence of breast tumors, taking into account and learning from the feedback provided by trained radiologists and re-analyzing when necessary to ensure continuous improvement and accuracy of the algorithms.5
Breast Ultrasound
Several companies have already invested in AI solutions that can identify anatomical features, auto-annotate pathologies to reduce labeling errors and make more accurate measurements. While in its early stages, the rapidly growing field of AI has shown potential for clinical applications in breast US. The Japanese Journal of Radiology has published a study showing that AI and ML can identify benign and malignant breast masses with better performance (50 percent-time reduction) than the human radiologists who participated in the study.6
The rise of AI within the radiology community has been both celebrated and has caused concerns for radiologist’s job security. The future of imaging interpretation will most likely not be replaced by machines, but rather enhanced by these technologies which are showing promise in decreasing image interpretation times with an increase in accurate diagnosis.
Barbara Smith is a Partner at Paragon Consulting Partners, LLC. With over 25 years in the medical imaging space and an unparalleled understanding of the needs of clinical staff and care providers, she oversees the radiologic and women’s imaging service lines. Her knowledge of clinical systems and their impact on daily workflows makes her an invaluable asset to clinical system selection, implementation and optimization.
Related Mammography Content:
Animation to Bring Clarity to Dense Breasts
Improving Clinical Image Quality for Breast Imaging
Breast Imaging in the Age of Coronavirus
Abbreviated MRI Outperforms 3-D Mammograms at Finding Cancer in Dense Breasts
VIDEO: Use of Breast MRI Improved Cancer Detection in Dense Breasts in Dutch Study — Interview with Gillian Newstead, M.D.
Screening MRI Detects BI-RADS 3 Breast Cancer in High-risk Patients
References:
1. U.S. Breast Cancer Statistics www.breastcancer.org/symptoms/understand_bc/statistics. Accessed Jan. 13, 2020.
2. Society of Breast Imaging: www.sbi-online.org/RESOURCES/WhitePapers/TabId/595/ArtMID/1617/ArticleID/595/Digital-Breast-Tomosynthesis-for-Screening-and-Diagnostic-Imaging.aspx. Accessed Jan. 13, 2020.
3. Detecting Breast Cancers with Mammography: Will AI Succeed Where Traditional CAD Failed? pubs.rsna.org/doi/abs/10.1148/radiol.2018182404?journalCode=radiology. Accessed Jan. 13, 2020.
4. International evaluation of an AI system for breast cancer screening. www.nature.com/articles/s41586-019-1799-6.epdf?author_access_token=V_LKV2xpSv9G1dhANYeWM9RgN0jAjWel9jnR3ZoTv0M5zwPVx5jT4z_z-YkUZTBT6_1AtRXi8QouJM7xB-oSN-cVBoH7f_QTgx-yQN3UBEVfkvO1_5urNT-CZHGCEQNGlCuO69tMQYak4SmdoDqyzg%3D%3D. Accessed Jan. 13, 2020.
5. How artificial intelligence could impact breast MRI www.gehealthcare.com/article/how-artificial-intelligence-could-impact-breast-mri. Accessed Jan. 13, 2020.
6. How AI is Impacting Breast Health www.diagnosticimaging.com/breast-health-ultra sound/how-ai-impacting-breast-health. Accessed Jan. 13, 2020.