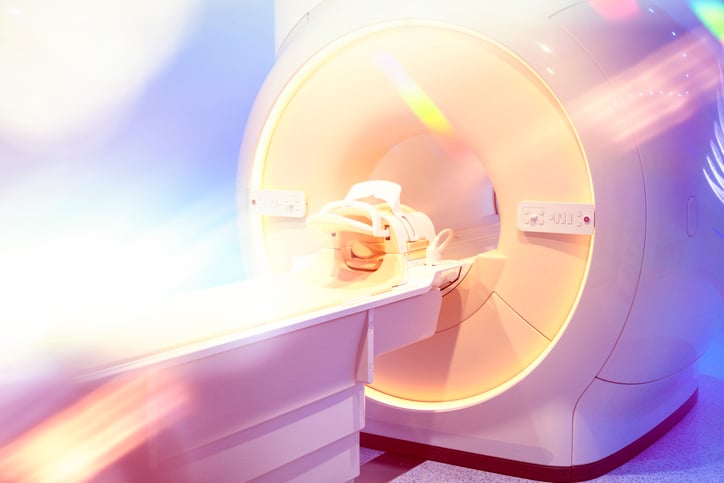
Appreciating the considerable advances in the clinical application of artificial intelligence (AI) within healthcare, the leadership of the American Society for Radiation Oncology (ASTRO), in planning its most recent annual meeting, recognized that, despite enthusiasm for AI implementation, considerable ethical challenges for practicing radiation oncologists exist. To answer those concerns, it offered “Exploring Ethical and Legal Implications of Artificial Intelligence in Medical Practice” during ASTRO 2023.
A stellar panel was assembled to identify priorities and opportunities to advance these initiatives toward patient outcomes and workflow efficiencies. Moderating the panel was Sanjay Aneja, MD, Assistant Professor within the Department of Therapeutic Radiology at Yale School of Medicine. Panelists included an interdisciplinary group of clinicians, physician scientists, data scientists and bioethicists to discuss various AI challenges. ITN is offering an abbreviated version of the panelists’ presentations here, and will continue to report on AI in RO.
What Radiation Oncologists Using AI in Clinical Practice Need to Know
Sushil Beriwal, MD, MBA, FABS, FASTRO, FICRO, is a Professor and Academic Chief at Allegheny Health Network (Pittsburgh, Pa.) who also holds the position of Vice President of Medical Affairs at Varian (Palo Alto, Calif.), and offered his expertise on “Artificial Intelligence (AI) Tools in Radiation Oncology Clinical Practice” during ASTRO 2023.
When I asked Beriwal what advice he offers early career radiation oncologists, and those leveraging the technology in clinical practice, he offered this: “The use of AI in medicine in general and in radiation oncology is part of daily practice for many practitioners. The adoption of various forms of AI, including segmentation, is saving time and adding uniformity to care.” He added, “We need to validate the available models as we incorporate them into our practices to make sure it performs well in the real world as in controlled environment, and is representative of our patient population.”
Beriwal defined AI as the simulation of human intelligence processes by machine, especially computer systems, noting it leverages the ability of a computer algorithms to approximate conclusions based solely on input data. The model ingests large amounts of labeled training data, analyzes the data for correlations and patterns, and uses patterns to make predictions about a future state. He emphasized the importance of inputting data. “Any model is as good as what data is used to derive that model, so whenever one evaluates an AI model or product, it’s vital to be very sure about the presentation of that data of the population which it is going to treat,” said Beriwal.
He identified the top goals of AI in radiation therapy: 1) improve efficiency and save time; 2) offer uniformity of care; 3) improve quality of care; 4) enhance access to care; and 5) help with training. He then addressed how ROs use AI in day-to-day practice: image acquisition, segmentation, physician plan of care, treatment planning, quality assurance, treatment delivery and outcome prediction.
“Current AI products are not going to replace us, I just want to put that on the record,” said Beriwal, adding that AI should be seen as a way to assist physicians. His session covered a wide range of areas where AI is applicable for radiation oncology, from image acquisition and treatment planning to workflow, offering examples of areas where it is most useful, such as in prostate cancer. He stated: “We do realize the importance of MRI in prostate cancer, but we always do a CT scan for those calculations. Now, we can convert with the AI algorithm, the MRI, the Dixon sequence to synthetic CT. So we can contour MRI and use synthetic CT for those calculations. The published data on the synthetic CT for brain and pelvis have shown the dose calculation is within 1% of what you would calculate with planning CT.”
Focusing on image enhancement, he said that ROs can decrease the exposure of a CT scan or decrease acquisition time for MRI, and use AI to enhance the images. “That’s a good value proposition for the patient, with less exposure to the X-rays or less time on the MRI scanner by using AI algorithm to improve the image,” said Beriwal.
He also zeroed in on segmentation, noting that it is a tool which has rapidly developed in the last five years. “This is a tool which we are using on day-to-day practice in our own clinic. We need to make sure we know what metrics are to be used to validate the product. When we start talking about the target, we need to understand which guidelines will be used, as a particular practice may or may not be consistent with the solution that is available.” He emphasized the importance of knowing whether a model represents all the demographics, and whether it adequately represents the type of population to be treated.
“It is important when we look at the solution to see what metrics were used to evaluate the AI model in comparison to what a human would do,” Beriwal said. Further, it’s important to evaluate the product or the solution with respect to what methodology was used, and how much time was saved. He discussed the work of his team which conducted evaluation work in their clinic using qualitative scoring, like visual scoring from one to four. In one clinic they implemented solutions for both target and organs at risk (OAR). They found that overall, the satisfaction score was very high. The majority of OAR had very minimal edits. The mean editing score was less than two, and the satisfaction score was more than four — saying that these are practical solutions which physicians and dosimeters like.
Berwali spoke of one of the plenary presentations at the last ESTRO congress, where the researchers looked at OAR contouring across the globe, from developing countries and developed countries. “They found that inter-observation variation, the contouring bias and the contouring time all of them were much better. So imagine the access to care in places where expertise is not available. This could be the solution which could improve quality of care and also save time, making them efficient and faster.”
He shared information from his team’s own evaluation in their own network. They looked at contouring for organs by different physicians for different contouring. They found that in the majority, more than 95% of the time, the contouring was acceptable with minimal minor edits. Only less than 5% of the time the ratings were below three. Beriwal reinforced that it performs well, most of the time, but added that, infrequently, it does notl. To this he said, “We as a physician have the greatest responsibility to make sure we review them and accept them. Because if 5% of the time it is not acceptable, that can reflect on your practice. That’s why it is important that these are AI assist and not AI replacement.”
In addressing data and validation, he emphasized the importance of all demographics being included. One of the solutions he and his team evaluated was pelvic lymph node contouring for CTV. This data and model was generated on male pelvis and prostate cancer, and validated on male pelvis. They wanted to see how this whole auto segmentation of pelvic node performs in female pelvis. To that end, they looked at 50 patients with the same algorithm, and found that 96% of the time, the contours were acceptable with minor or no edits. It did well, but it did not perform as well in women as with men. So it was usable, but the fact that the model was not trained on women’s pelvis makes it a bit less acceptable and that’s a very important concept when evaluating a model in your practice: finding out what data set was used to create and validate the model.
In helping identify key considerations for the use of AI in RO, he pointed out the value of the number of cases in a model, saying: “There is always opportunity to improve the model. They are not an all or none phenomena, as there are situations where it does not perform well. With feedback, with the addition of more data sets, the model can improve. This I found to be a common theme. For now we look at it and fix it, but maybe in future we would want to be sure to add datasets to the model to improve it. That’s a very important thing to realize, that these are not all or none phenomenon, but these are a matter of AI being a constant improvement process.”
Another aspect where AI is being used is in treatment planning. The benefits are multiple, including saving time, making a homogenous plan, sparing organ risk, and use in clinical trials, among others. He then noted, “But the issue is sometimes it cannot capture our own preferences. We all have our own biases and nuances, and that can be hard to catch,” adding that the planning could be knowledge-based or deep learning.
Turning to the important issue of adaptive workflow, he said currently those who do adaptive radiation therapy are using AI in every aspect, from treatment planning to delivery to predicting outcomes, offering multiple examples of ways it benefits the physician and patient.
“These are evolving issues and there is a constant need for improvement,” said Berwali, emphasizing, “The model needs to be tested and improved as we get better in practice, because we can get a better plan and better distribution as there is improvement in the modeling.”
What are the challenges for AI in RO? According to Beriwal, there are a few primary concerns to keep in mind. Creating a dataset is critical. The segmentation model may be dependent on CT and MRI. Due to this, he said, it is important to make sure it is determined whether it is applicable in the CT scanner or MRI scanner, or not. Further, models are inherently unstable if it uses a small dataset. As any additional data set can change the model, it’s very important to understand the size which was used to create it.
Ethical Considerations of ChatGPT, Cybersecurity Issues
Specifically addressing this topic was Skyler B. Johnson, MD, Assistant Professor, Department of Radiation Oncology, University of Utah School of Medicine, and the Huntsman Cancer Institute.
In presenting “Ethical considerations of AI Chatbots-ChatGPT and Beyond,” Johnson explored the ethical implications for physician users in radiation oncology and cancer patients in the context of these technologies, offering a critical examination of potential benefits as well as challenges in medicine, hoping to raise awareness and promote responsible usage of AI chatbots in healthcare.
After offering a summary of recently published journal articles and research, he said this of the examples offered: “This just highlights, in my opinion, the need for stringent accuracy and reliability measures. It’s my hope that in the future this is what most of the research done in this space will address.” He offered a cautionary note on application of these technologies, saying they “should be used to complement human instructors, not necessarily replace human instructors, because there is a lot of value for human instructors, and we have to balance the technological advances with human expertise. They should be working in collaboration.”
Johnson spoke to the value of future research in key areas: Clinical decision support; academic and educational support, including AI-enhanced curriculum development; administrative efficiency, such as automated documentation, data analytics and reporting; ethical and regulatory considerations, specifically guidelines for ChatGPT use, regulatory compliance and safety audits.
In offering key takeaways, he highlighted both the challenges and opportunities for radiation oncologists: 1) ChatGPT offers valuable contributions to radiation oncology, education and administrative tasks; 2) Ethical considerations and limitations must guide its responsible use in critical medical contexts. To this he said, “We have to trust some of its outputs but verify its accuracy and reliability, as there are many limitations that we have to be aware of;” and 3) Future developments may enhance reliability but rigorous evaluation is essential.
Speaking to the issue of “AI Cybersecurity and Patient Safety” was Junying Zhao, PhD, MBBS, University of Oklahoma Health Sciences Center. Zhao addressed important considerations, cautionary measures and critical decisions and actions to be made by healthcare providers, administrators and clinicians as these potentially damaging and costly threats continue to wreak havoc on healthcare delivery.
Legal and Regulatory Implications
Tony Quang, MD, JD, is an attending physician in the department of radiation oncology at the Long Beach VA Medical Center (Long Beach, Calif.), who — as the only panelist who is both a practicing radiologist and lawyer — offered his unique insights into “Legal and Regulatory Implications of AI in Clinical Oncology.”
He reinforced that artificial intelligence permeates every aspect of healthcare and is a very important topic for radiation oncologists. Addressing laws and liability, he urged the audience to think of it as accountability. “Accountability in place of liability is probably more palatable to group of clinicians, physicists and technical experts. Accountability is important because it’s through that which we can be focused on quality, especially quality care for patients,” stated Quang.
Notably, Quang reinforced that AI is not just algorithms, but these tools involve regulations, liabilities, informed consent, privacy and ethics. He referenced the involvement by and action from ASTRO resolutions, ASTRO PAC, innovators, leaders and venture capitalists — all who are very much a part of this, as he remained focus on regulations and liability. Quang identified the various types of liability: Medical malpractice, institutional, vicarious, product, enterprise and privacy (HIPAA). In noting challenges ahead, Quang said there are a lot of concerns, the largest being that there may be incongruent decisions from the physician versus the AI platform.
As a radiation oncologist who treats lung cancer, he referenced the ways ROs use data to train a model to diagnose and detect cancers, noting that in RO, it is used for formal registration, for segmentation, measurements, image enhancements and also clinical support decision. He addressed the multiple types of solutions of AI: White box interpretable and black box explainable.
Focusing on US Food and Drug Administration (FDA) regulation of AI, he noted the FDA follows a functional approach rather than AI specific framework. He reported there are currently three pathways based on patient risk exposure: Class I and Class II, De novo review, and 510 K process with substantial equivalent predicate.
On the AI legal oncology landscape, and the regulatory role of the FDA, he offered actionable insights to help the audience understand the medical legal aspects of AI in practice for clinical support decisions:
1. Learn how to better use and interpret AI algorithms.
2. Encourage professional organizations to take active steps to evaluate practice-specific algorithms, and provide guidelines for implementation.
3. Ensure administrative efforts to develop and deploy algorithms in hospitals.
4. Check with the malpractice carrier.
5. Be part of the AI development process.
Quang reinforced the importance of physicians playing a leading role in defining the standard of care for AI use, adding that medical malpractice liability using AI in clinical practice essentially remains unsolved, with evolving legal framework.
SIDEBAR:
Key Takeaways on Artificial Intelligence in Radiation Oncology
• AI in radiation therapy is still in early phase, but rapidly progressing
• It has the potential to improve care, make it faster, efficient and homogeneous
• Auto segmentation, treatment planning and quality assurance are already being used
• AI has made adaptive plans/treatment feasible
• Physicians have the responsibility to ensure AI performs well in their practice
• The dataset used to generate and validate a model may influence its performance
• The regulatory pathway for approvals is different for predictive or practice changing applications
Find more ASTRO conference coverage here
Related Content:
AI Essentials in Radiology: Experts Weigh In
AiMed Global Summit 2023 to Focus on “Changing Healthcare One Connection at a Time” in San Diego
AiMed Global Summit’s Lineup Announced
AiMed 2023: Changing Healthcare One Connection at a Time
Find more AiMed23 conference coverage here
The Pros and Cons of Using ChatGPT in Clinical Radiology: An Open Discussion
ChatGPT Passes Radiology Board Exam
JNM Explores Potential Applications for ChatGPT in Nuclear Medicine and Molecular Imaging
New Research Suggests AI Image Generation Using DALL-E 2 has Promising Future in Radiology