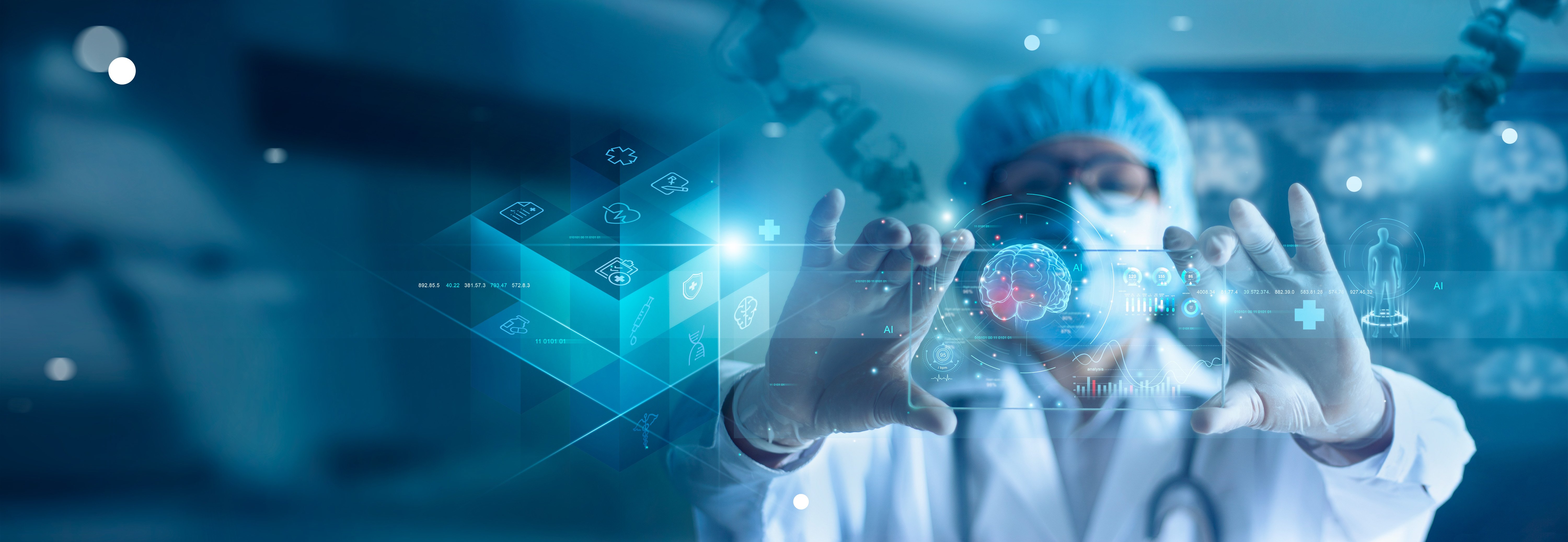
Reflecting the expansive growth in applying machine learning and AI tools to radiology, and toward establishing a greater understanding of this powerful tool, ITN’s editorial team is continuing its coverage of significant advances and expertise shared by those leading the way on this fast-moving frontier.
In this feature, the editorial team shares the second of a multi-part overview of information originally presented during an RSNA 2022 keynote session, “Back to the Basics: What Do Rads Need to Know About Radiology AI.” In addition to the two experts whose sessions are summarized here, the panel included Dania Daye, MD, PhD, Massachusetts General/Harvard-Martinos Center for Biomedical Imaging, and Walter Wiggins, MD, PhD, Duke Health, Qure.ai consultant, whose valuable insights were shared in the July/August issue.
Ahead of RSNA 2023, where updates, products and news of advances on this front will again be in the spotlight, ITN has comprised an overview of key points made during that important session. Here, a compilation of session points shared by two widely recognized leaders in the field, Katherine P. Andriole, PhD, and Linda Moy, MD, with an overview of their deep involvement and leadership in this field.
RSNA 2022 Gold Medal Recipient Katherine P. Andriole, PhD, is an Associate Professor of Radiology at Harvard Medical School, Brigham and Women’s Hospital and Director of academic research and education at the Mass General Brigham Data Science Office. She is a long-standing member of the RSNA Radiology Informatics Committee, serving on Machine Learning Steering and Data Standards sub-committees. A faculty member for the RSNA Imaging AI Certificate Program and subject matter expert for R&E Foundation’s Grant Oversight Committee, Andriole is co-director of the Imaging AI in Practice Demonstration. She recently co-chaired the Society for Imaging Informatics in Medicine (SIIM) Conference on Machine Learning in Medical Imaging (CMIMI) held in early October in Baltimore, Md.
Linda Moy, MD, is Professor of Radiology at the NYU Grossman School of Medicine with appointments at NYU Center for Advanced Imaging Innovation and Research and NYU Vilcek Institute of Graduate Biomedical Sciences. She is director of breast MRI (clinical and research) throughout NYU’s Health Network, and co-leads an international AI team across five NYU Langone Health institutions. Notably, Moy is current editor of the RSNA journal Radiology, the first woman to be named editor of this premier journal in the medical imaging field. In addition, Moy is Vice President of the Society for Breast Imaging (SBI).
AI and ML Fundamentals
In presenting “Basic Principles of Artificial Intelligence/Machine Learning in Radiology,” Andriole was both educational and supportive to attendees who ranged from early career novices to those currently utilizing available technology in their clinical practices.
She offered an introduction to algorithms, establishing participants in the role of data science officers in a hospital radiology department. Segments presented were: an overview of basic principles, focused on machine learning in radiology; terminology; a primer on how it works at a high level; discussion of enabling factors and existing limitations; and how radiologists can go forward and deliver. Excerpts are offered here.
“In level setting the related terminology, I hear many people use the terms artificial intelligence (AI), machine learning (ML) and deep learning interchangeably,” Andriole explained. “There is a distinction. When talking particularly about machine learning, that is working with pixel data or the images themselves,” she noted. Generally, today, practitioners are conducting what is called supervised machine learning, where a mathematical model is trained based on showing it examples where we know the answer, where there is a label (stroke/no stroke, tumor/no tumor, and so on).
Artificial intelligence is a broader field where we try to enable the machine to act like a human. Machine learning uses statistics, or math, to learn from experience, and that is to learn from data. And deep learning is just a subset of that with multiple hidden layers. The distinction: what deep learning is, in particular with imaging, is it learns discriminatory features that best predict the outcome. Is there a tumor present or absent? Is it benign or malignant? This is different from what you’re used to with your computer aided detection systems in breast imaging, which are classical machine learning where the features that were detected were human find. Here it is data driven. Deep learning is a subset of machine learning, a subset of AI.
When talking about machine learning, the focus is often on using a convolutional neural network (CNN). A convolutional neural network is used where you can see the output of the previous layer becomes the input into the next step. The goal is to optimize the weights to identify how much is carried forward to the next step. Convolution is a mathematical operation that helps us bring local spatial patterns in the data, she said, further explaining that they are referred to as neural networks, because they’re interconnected, like we think neurons are, not one to one but one to many.
Andriole walked through a supervised machine learning set, in order to show how one would build a model to detect hemorrhage or stroke on a head CT. “We show it a training set where we have data labeled with no features of stroke, as well as features of stroke, and the mathematical model iterates until we optimize a function or minimize the loss function,” she explained. “What is the loss function? It’s just the difference between what the model says is happening, and what is the true answer. As we do this, we optimize or minimize that loss function, such that when we show it an image set or a case that it has never seen in the development of the model, it can predict stroke. Mathematically, we are pulling out features from that data. What features? All kinds of features that you as a radiologist are innately looking for as you interpret images, such as compress differences, size, shape, boundary, symmetry and texture, which is very important.”
Multiple steps are involved in building a model and the preprocessing steps are 60-70% of the efforts. The first vital element is developing a clinically relevant question. A data cohort definition is extremely important, she emphasized, noting that if this is not right, the model will not be as interpretable. Collecting, normalizing and annotating the data, a tedious process, is next in building the model. There is difficult, complex data structured in the electronic health record, unstructured data in radiology reports, medical imaging data, genomic data, as well as population health data. For imaging, multiple different vendors have different protocols for doing complex exams. This may impact the model, so getting a sampling of different protocol types can be useful.
Data set labeling is very tedious because a clear objective gold standard has yet to be established. Once you’ve done those preprocessing steps, then what you do, in terms of building your models, is start with that data cohort and build a training set on which the model will be developed and improved. Next, the developer trains a model, setting the parameters and hyper parameters of the model. Then there is a review of what’s learned, connecting the data together, and looking at the loss and performance functions.
Important questions to ask: What is the question to be solved? What was the data cohort used to train and test the model? On what cases does it fail? What performance metrics were used? May I see if it generalizes to my data? And what kind of quality assurance and monitoring is necessary? Always, always, always do the critical gut check, stressed Andriole. If it doesn’t make sense clinically, you take over the driving wheel. This requires team science with clinicians, data scientists, radiologists and others working together.
“AI has tremendous promise to automate not just the diagnosis, but also to assist workflow throughout the imaging chain. Hopefully it can help get high quality care to underserved areas. It’s a new tool for you, but it’s not magic, it’s math,” said Andriole.
How to Best Assess the Value of AI in a Radiology Practice
Focused on the practical application of AI in a radiology practice, Moy focused on offering a series of fundamental questions and considerations that are necessary to be asked by those looking to engage the technology.
She urged participants to ask the following: What is this AI system? How do I integrate it? How much does it really cost? The underlying question is whether this AI solution will work in your practice. What are the specific use cases? How can we ensure that this is the AI system that is required? Most AI systems have a narrow scope to one or two functions. Next, ask what problem the AI solution is solving, Who is coordinating the application design? She stressed the importance of performing a return on investments (ROI) assessment. What will the ROI be? And what stakeholders were at the table to calculate that investment? A benefit analysis will also answer a key question. That is, who does the application primarily benefit: our mission or our patients?
Once a decision is made to move forward, you really need to do your homework and ask the vendors a lot of questions. The devil is in the details, especially when it comes to quality data...When you’re setting the standard, it should beyond the expertise of a radiologist because you want a model to outperform the radiologists. The main point is that no machine learning algorithm can produce something from nothing. The key issue is whether the data is suitable to answer the primary question.
“We need to test the AI system on data it has not seen before,” said Moy. “Small datasets can cause mis-estimation of population accuracy.”
She emphasized the need to test the AI system on data it has seen before. The vast majority of papers that were accepted in Radiology this year had one or more external datasets. You have to realize you are creating a dataset, and it takes a significant commitment and effort to do a pilot study and test it.
Speaking to interoperability, Moy reinforced a key consideration, which is asking whether you trust it? She noted, “This is essential because the scientific community, colleagues and patients need to trust a model. Many AI systems are interpretable black boxes. This introduces the concept of bias. As we have this concept of bias, you introduce bias because data sets a small, single center even a single vendor. We want AI systems to generalize across heterogeneous patient populations. To mitigate AI bias we really need humongous and diverse datasets. So I would say our mindset should be building trust while maintaining skepticism.”
In reinforcing the important considerations which radiology teams should keep in mind, Moy also introduced the concept of automation bias where radiologists may be over-reliant on AI solutions, offering this insight: “So first, right now there is a lack of safety indicators and radiologists may be following an inappropriate system. This is happening exceedingly quickly.”
Moy presented a range of studies and papers, one of which showed that more inexperienced radiologists are more likely to trust the AI system. There are two types of automation bias, which she said both occur in the malfunction of the algorithm, and it’s either not observed or just disregarded. AI decisions are based on features that are not perceptible to the human eye. The other are commission errors based on erroneous acceptance of a machine decision. She noted this happened in early generations of computer-aided detection.
Integrating AI Systems into Practice: Assessing Impact on Efficiencies, Infrastructure
Next, Moy talked about integrating AI systems into a practice. She recommends this before signing a contract: “You have to ask yourself this litmus test question: Is this a system a ‘nice to have’ versus a ‘must have’ use case? Is it because there is a perception that your practice will be subpar if you don’t have AI? Right now, most practices do not, but these answers are important to keep in mind. Currently, there are a lot of tools, but the reality is that if you decide to purchase an AI tool, it should be purely for solving problems that you encounter in your practice.”
Using one use case example in reinforcing her recommendation, she asked if the AI system will detect more than pneumonia on a chest X-ray. She said, “Beyond lesion detection, what other bells and whistles can the system perform for you? Most importantly: is the solution aligned with your hospital’s enterprise’s goals? The potential of AI has been that it can increase practice efficiency by reducing either upstream and downstream costs. Downstream is where it helps radiologists, and it seems upstream is where most efficiencies are coming into play today ... it’s recommended to perform a local evaluation to show that an AI system will work in the practice, and that radiologists ask vendors for a free trial and a no obligation opportunity to test the AI product. These all help to determine whether the AI practice will augment or distract your workload.”
Moy further urged those considering such systems to focus on key questions, encouraging participants in the session to proceed with full understanding, noting: “What are the IT infrastructure requirements? How will AI impact your operational workload? Is the system interoperable with your existing system design? If not, the time savings may not be feasible. Contact the IT team as early as possible when first considering purchasing an AI system to ensure you are incorporating their expertise going forward.”
Importance of Value Proposition
Moy reinforced the significant factors that should be considered when deciding where and how and what AI model best fits into a radiology practice moving forward. She added, “The value proposition is key. Right now, there is a lot of hype. Asking questions of vendors to ensure what is really possible versus hype. Is that really possible? Will the AI solution really pay for itself? It’s critical to assess the costs such tools will impose, and realistically determine if and where the model fits best into our practice moving forward.”
The other hard issue is really measuring new efficiencies, especially if it’s not going to be the higher volume, or decreased interpretation time but what you expected. “I also think it’s hard because these are now soft measures ... How do you measure less burnout among radiologists, or how do you gauge whether there is a fear of them quitting? So this is important, but difficult, to measure,” she stressed.
Moy concluded with a final note: “Once a practice has decided to take the plunge, after the trial period, there is the issue of integrating the system, which takes months. There are direct costs in the contract but also hidden costs as it can take a long time to train radiologists. There are costs to monitoring the AI solution, upgrades and other essential components which the RSNA journal Radiology has published in multiple articles.”
Related Artificial Intelligence Content:
AI Essentials in Radiology: Experts Weigh In
AiMed Global Summit 2023 to Focus on “Changing Healthcare One Connection at a Time” in San Diego
AiMed Global Summit’s Lineup Announced
AiMed 2023: Changing Healthcare One Connection at a Time
Find more AiMed23 conference coverage here
The Pros and Cons of Using ChatGPT in Clinical Radiology: An Open Discussion
ChatGPT Passes Radiology Board Exam
JNM Explores Potential Applications for ChatGPT in Nuclear Medicine and Molecular Imaging
New Research Suggests AI Image Generation Using DALL-E 2 has Promising Future in Radiology