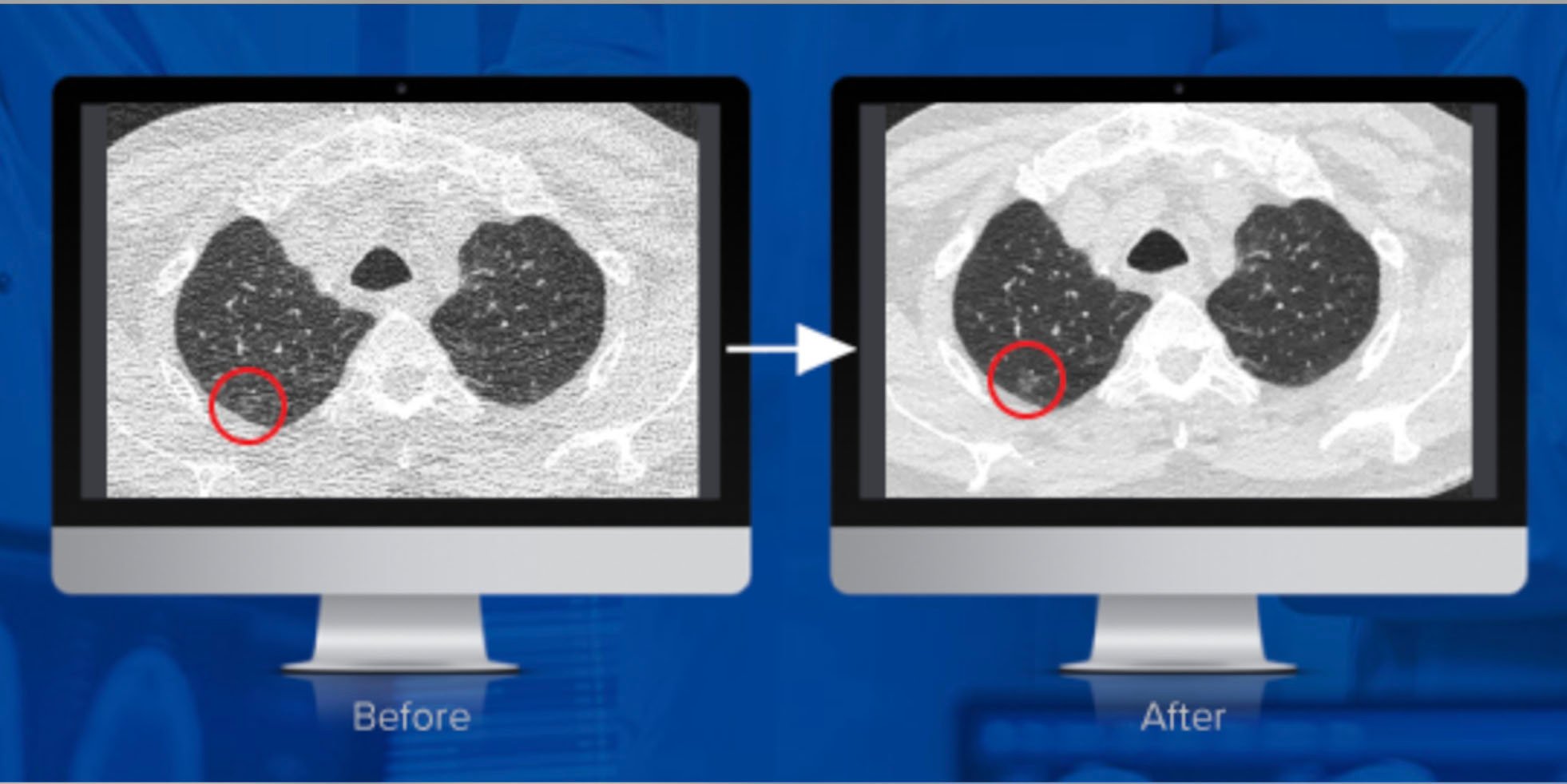
Studies conducted by Mass General Hospital and University of Virginia have concluded that PixelShine, a disruptive technology from AlgoMedica, significantly improved the diagnostic quality of CT scans acquired at reduced radiation dose. Here, you can see before and after noise reduction is applied.
Computed tomography (CT) imaging has broad diagnostic application and is the imaging gold standard for many clinical indications. However, CT imaging exposes patients to higher doses of radiation than other methods. It carries increased cancer risk for all patients, particularly those in higher-risk categories such as pediatric, obese or oncology patients who receive regular screening.
While low-dose and no-dose imaging techniques and modalities exist, a compromise often must be made between patient dose exposure, clinical utility and cost. Within CT, a direct correlation can be drawn between diagnostic image quality, clinical utility and radiation dose exposure. Lower-dose procedures produce noisier images, which can impact clinical utility, radiologist productivity and patient care. Conversely, with increased dose, image quality tends to improve, rendering subtle pathologies more visible — which ultimately benefits radiologists’ diagnostic confidence.
CT imaging protocols can be optimized to adjust dose according to patient and procedure requirements, but this process is complex and cumbersome, resulting in inefficient workflow and increased operational costs. Furthermore, older model CT scanners require higher dose to produce clear images. However, upgrading these devices is often out of reach due to the high associated capital costs. As such, older modalities are often limited to routine cases, resulting in inefficient workload balancing and increased wait times for higher-risk patients.
So, how can healthcare providers balance the requirement for high-quality, precise imaging with tight budgets and the need to reduce radiation exposure risk for their patients? New artificial intelligence-based deep learning reconstruction (DLR) and post-processing techniques have recently become available. These methods can consistently improve diagnostic image quality at the lowest attainable dose across all patients and procedures — far beyond what is possible with current reconstruction techniques. This presents a huge potential for imaging organizations to optimize CT imaging programs.
The Cascading Impact of CT Image Noise
Image “noise” is characterized by unwanted variations in pixel values that cause a grainy or blurry appearance in CT images. Image noise decreases the diagnostic utility of images and reduces the conspicuity of small pathologies. While noise is an inherent part of all CT images, it is more prevalent in lower-dose and thin-slice exams. The paradox between improving diagnostic image quality and reducing dose exposure has a complex and generally negative cascading impact on the clinical and operational aspects of CT workflow.
Poor quality images resulting from high noise are more difficult for radiologists to interpret, forcing them to spend more time carefully reviewing study information. This not only reduces reading efficiency and increases report turnaround time, but it also negatively impacts radiologists’ clinical confidence and the clinical value they can add.
Compounding these challenges is the fact that CT image noise varies across the many CT scanners typically found within a healthcare organization. Because radiologists read studies acquired by these many scanners, they are required to adapt their reading methodology for each. This results in inefficient reading workflow and reduced productivity. It can also be a significant contributor to radiologist frustration and fatigue, due to increased reading burden and constant challenges to their clinical confidence.
The Impact of Iterative Reconstruction
In the late 2000s, iterative reconstruction (IR) was introduced and remains a commonly used technique for improving the quality of CT imaging studies. While IR can reduce image noise and significantly moved the needle in terms of dose reduction, IR images can take substantially longer to process. This approach also has limitations in how much dose can be reduced before images take on a blurry or waxy appearance. IR algorithms are also vendor and scanner specific. This prevents organizations from upgrading all their CT scanners to IR technologies without sweeping and costly capital equipment replacements. The result has been a phased-in adoption of IR over many years, as CT systems are upgraded or replaced.
Images processed by IR have a different appearance and can introduce distinct artifacts and textures that radiologists have had to become familiar with before they can be confident in their clinical diagnoses. While the promise of IR has been largely realized, the limitations of IR cannot be ignored. We have now entered the next phase of CT image reconstruction and post-processing where AI technologies will help to overcome these limitations and push CT imaging into another era.
Challenging Use Cases
There are a number of clinical applications that are not well-suited for IR, because of the very low-dose and diagnostic requirement for sharp, high-contrast images with fine details. For instance:
- Low-dose lung screening programs that must balance the detection of very small nodules indicative of early-stage cancer versus the increased risk from the cumulative exposure of X-rays. These programs have a competitive advantage when they can promote their ultra-low dose to a population that is increasingly aware of the X-ray dose risks.
- Pediatric oncology patients who are highly sensitive to the damaging effects of cumulative radiation but are less suited to no-dose alternatives like ultrasound or magnetic resonance imaging (MRI).
- Obese patients who are subject to higher radiation dose in order to penetrate excess subcutaneous fat and obtain viable imaging.
- Small and complex anatomies such as cardiovascular, orthopedic and ENT temporal bone.
- 3-D post-processing, reconstruction, rendering and printing procedures that necessitate 3-D data sets with low noise and high contrast — which require clearly delineated spaces between anatomical boundaries in order to produce optimal results.
For the above use cases, the limitations of IR are felt more extensively. First, the “waxy” appearance typical of images with a heavy application of IR can slow radiologist workflow because of the increased time required to ensure a pathology is not missed or anatomy altered. In addition, IR cannot be applied to many ultra-low-dose exams at all, which results in the radiologist having to “read through” noisy, low diagnostic quality images that are difficult and time-consuming.
Unique Challenges of Older CT Scanners
The above challenges are further exacerbated on older CT scanners, which inherently produce noisier images because their tubes, detectors and automatic dose management software tend to be less sophisticated. Therefore, they generate more noise at any given dose level, often requiring higher dose to maintain diagnostic image quality.
While older scanners are reliable and well-suited for routine imaging, and therefore are very common, their inherent limitations make them less suitable for certain patient cohorts. As such, imaging facilities often do not use them for certain advanced procedures or patient types. This can increase patient backlog and wait times, and result in inefficient technologist workload balancing and modality utilization. These older scanners also have fixed costs for electricity, service and operation, which need to be offset with higher utilization. Any opportunity to increase their clinical utility has important financial and operational implications to the organization.
While some new AI-based CT image reconstruction software is only available on certain premium models of new scanners, other vendor neutral solutions have emerged in the marketplace. These vendor neutral solutions are a critical development, because they can be universally applied across all new and older model CT scanners from all vendors. This provides significant financial value by extending the diagnostic life of all CT scanners and deferring capital and professional services costs. It can also improve operational efficiency and reduce patient backlogs and wait times by balancing CT imaging workflow, improving modality utilization and harmonizing image quality across the entire organization.
Machine Learning Is a New Opportunity
The application of new AI-based machine learning technologies to CT imaging provides a new variable that clinical and technical stakeholders can use to directly manage both dose and image quality — and, therefore, indirectly all of the subsequent financial, clinical and operational factors they impact.
Image noise resulting from low-dose CT procedures causes a negative cascading effect on the quality, efficiency and cost of imaging services. AI-based DLR and post-processing techniques are able to process CT images in a matter of seconds — to reduce image noise across a much broader range of doses and exam types than IR.
With these techniques, the compromise between dose and quality can be eliminated, delivering clinical, operational and financial benefits. Because some of these are vendor neutral technologies, they enable image quality to be harmonized across all CT scanners. Radiologists can more quickly and confidently interpret images without the need to accommodate the image quality variability common today. This holds the opportunity for radiologists to increase their reading productivity, as it could shorten the time needed to read exams coming from certain scanners.
This will likely be more impactful on exams coming from older scanners or challenging exams that are inherently noisier or include more subtle anatomy in pathology. Financially, operational costs associated with inefficient workflow are significantly reduced, capital equipment replacements can be deferred and reimbursements increase as a result of greater overall productivity.
From the patient perspective, concern regarding the negative effects of radiation can be addressed by leveraging DLR and post-processing to offer screening services that achieve the lowest attainable dose. In doing so, imaging organizations are better positioned to attract new referrals and patients, especially for programs targeted at higher-risk populations such as low-dose lung screening. In fact, the recent expansion of low-dose lung screening eligibility creates a significant opportunity for healthcare organizations to take advantage of this new technology.
Early adopters of this new technology have an opportunity to explore the impact on various radiology productivity areas — including (but not limited to) the degree to which:
- Dose can be universally reduced across all CT scanners and patient populations
- Changes in the conspicuity of subtle pathologies
- Improved radiologist report turnaround time and clinical confidence
- Applicability in ED settings where the processing speed of IR can limit its clinical utility
- Financial ROI from increased patient throughput, modality and technologist utilization, and extending the useful life of CT scanners
The Potential of DLR
AI-based deep learning reconstruction and post-processing holds huge promise for a positive impact on clinical, operational and financial aspects of CT imaging workflow. It has the potential to reduce radiation dose exposure both dramatically and consistently below current industry guidelines and well beyond what was previously attainable with existing iterative reconstruction technologies.
Comprehensively reducing image noise and harmonizing image quality for all CT scanners and all types of CT studies can have a profound impact on the quality, efficiency and cost of imaging procedures — particularly for higher-risk patients and challenging use cases like pediatric and lung screening studies. Even where low-dose protocols are in place, the use of these new AI-derived approaches can further improve diagnostic image quality, modality utilization and radiology workflow efficiency.
As patients become increasingly concerned about the possible negative effects of radiation, more are actively seeking screening programs that can offer the lowest attainable dose. As Low As Reasonably Attainable (ALARA) can have new meaning with the use of the new DLR and post-processing approaches. Which, in turn, can give provider organizations a competitive advantage. They will stand out among other low-dose screening programs by offering their patients the safest and most reliable CT screening programs.
Early adopters of DLR and associated AI post-processing technology stand to realize immediate returns in terms of image quality, diagnostic accuracy, and technologist, radiologist and patient satisfaction. Longer-term, improved operational efficiency, increased patient throughput and optimized modality utilization have the potential to deliver additional significant improvements in operational and financial performance.
Mikael Strindlund is president and CEO of AlgoMedica Inc. Before joining AlgoMedica, he was most recently CEO at Hermes Medial Solutions AB, a Swedish Medical IT company focusing on Nuclear Medicine. Prior to that, Strindlund was global business leader for Philips Healthcare’s Computed Tomography division. He has also held positions as Business Unit Leader at large corporations such as Siemens Medical and Getinge Maquet, as well as assignments as CEO of smaller publicly listed MedTech companies.
Related Imaging Content:
Initial Results Reported for First Crowd-Sourced CT Image Quality Study
Reducing Patient Radiation Dose to Meet the Regulatory Need
Medical Imaging Radiation Exposure in U.S. Dropped Over Past Decade
Radiation Dose Management Market worth $422.65 million by 2025