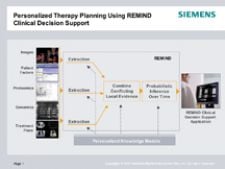
The more knowledge a physician has about a disease, the better equipped he or she is to care for the patient. That is the idea behind leveraging clinical information for decision support. The future of knowledge-driven clinical decision support is about being able to analyze all available information from patient records and make inferences based on this information.
One of the most cutting-edge clinical decision-support projects today is underway between Siemens Medical Solutions and MAASTRO (Maastricht Radiation Oncology), a leading radiation oncology treatment center in the Netherlands. They are jointly developing an application called REMIND Clinical Decision Support (CDS).
The REMIND platform (Reliable Extraction and Meaningful Inference from Nonstructured Data) includes data extraction such as natural language processing or proteomic analysis, data combination and probabilistic inference. REMIND is designed to search through all patient records, analyzing demographics, clinical factors, imaging information, treatment plans, delivered treatment, genomics and proteomics.
To get to the bottom of this new data mining technology, Imaging Technology News interviewed Professor Philippe Lambin, the clinical head of radiation oncology at MAASTRO, the Radiology Oncology Treatment Center in The Netherlands, about REMIND technology.
How is decision support currently being used in the clinical setting?
We want to develop it for lung cancer, and, for each patient, we want to be able to compare different types of treatment, and for each treatment have a prediction of complication and outcome. For late lung cancer it would be specifically survival at two years and late lung complication and early esophageal complications. These predictions should be validated using objective numbers to make a decision and take into account the wishes of the patients.
Are there any statistics to demonstrate how decision support improves patient outcome?
While there is more experience in radiology, in radiotherapy (RT) there aren’t any statistics to demonstrate how decision support improves patient outcome. There is modeling of theoretical considerations.
We know in RT that the higher dose you can give, the better the outcome. The dose is determined not so much by the outcome you want to reach, but more by the complication. If you have better predictions of the complications, you can anticipate through various treatments what the maximum tolerated dose will be and therefore have a better outcome. This is modeling of theoretical considerations, but to my knowledge there is no real clinical data in radiation therapy.
What are some of the challenges in developing a decision-support solution?
The biggest challenge in my eyes is to get good data, complete clinical data, to build the predictive algorithm. Because the algorithms are quite powerful to predict you also need to gather independent sets of data. We call them training data sets. You make a first algorithm, and then you have to validate this algorithm on independent validation data sets. It’s even better if data sets come from different centers.
Linked to that you need to build a large database to gather all of this data, and you need a database for imaging, validating data and also comparing quality of the treatment. That is the difference between radiotherapy and radiology – the quality of the treatment. You could have, in theory. a tumor with very good prognosis, but if the treatment is bad the patient will die. So you have to incorporate quantitative data on quality of treatment. And that is not so obvious.
What are some of the key features of the solution Siemens is developing in collaboration with MAASTRO?
A tool will tell the doctor what would be the extra diagnostic procedure that would be most efficient to improve the prediction. You can also give advice about an extra diagnostic procedure that would be most cost-efficient. The system can tell you that this PET scan will be the most efficient diagnostic tool to further improve your prediction. But a blood sample would be the most cost-efficient. So it would less efficient not to use the PET scan, but it’s the most cost-efficient method because a blood sample is much cheaper than a PET scan. This will be part of the REMIND platform.
To date, what has your research involved?
There are applications for research and development of new treatments and drugs. With molecular targeting drugs, we know that these new, very expensive drugs are only efficient on a subgroup of patients. The decision support will help the researchers focus on specific subgroups of patients who are more likely to benefit from the treatment. That will make it possible to have shorter, less expensive clinical trials.
There already is a prototype of REMIND, and version two was shown at ASTRO 2007 (American Society for Therapeutic Radiology and Oncology), but it is not yet commercialized. In patient care it is a tool to help the doctor, not a determinant.
How will decision support develop in the future?
We are extending it to several centers, and will let them develop the tool – and add more patients, more data and greater validation data set for training.
For patient care, the ultimate step is to validate the decision tool using evidence-based medicine approaches. The way we see it is when we are quite confident with the tools, we will compare, for example, state-of-the-art treatments for lung cancer versus individualized treatments based on the decision support, where in theory all of the patients could have received different treatments. The hypothesis is that with individualized treatments based on decision support, the outcome should be better and with less complications, and it will also be more cost-effective.