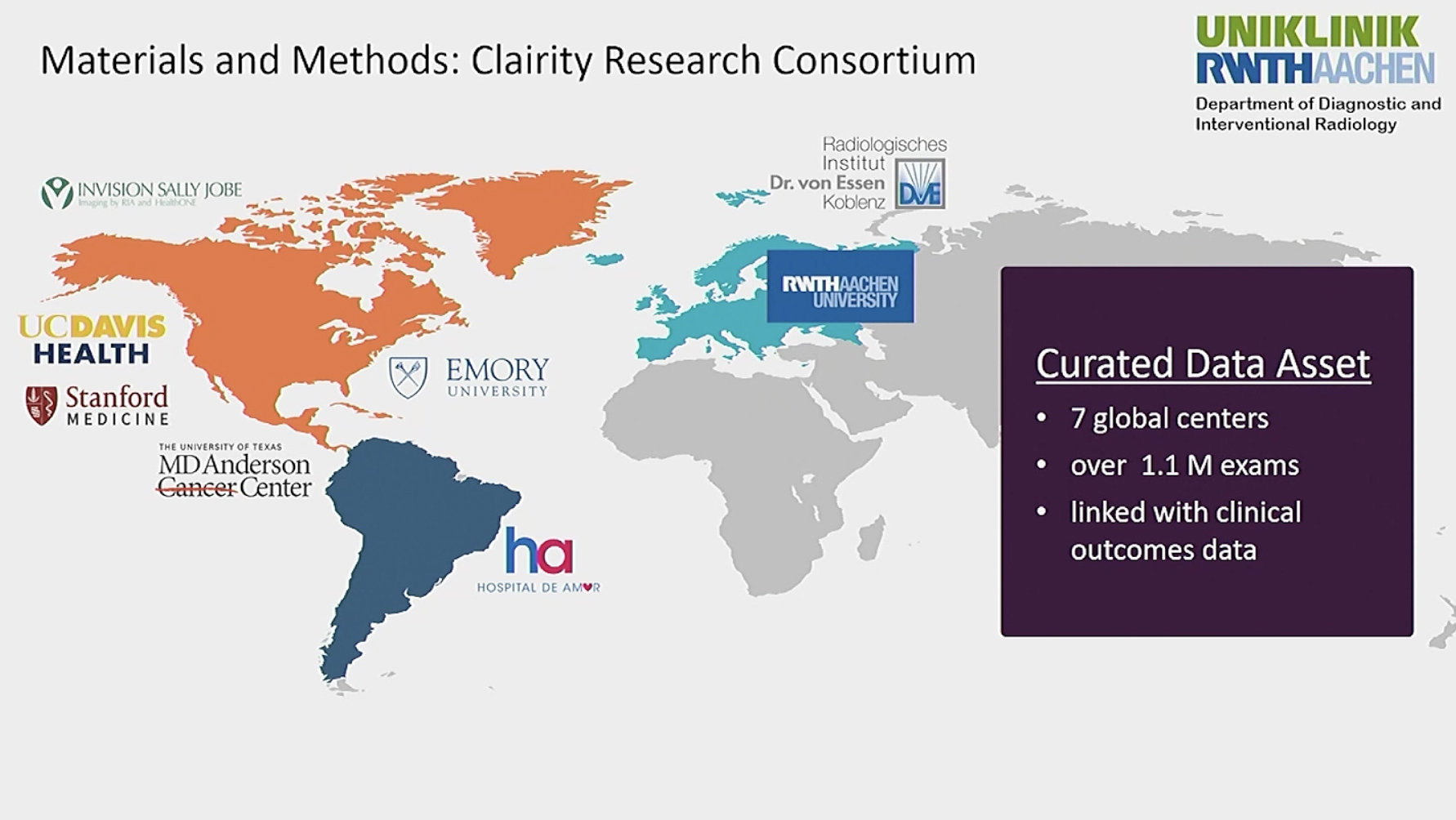
In tracking the latest findings from breast imaging specialists across the globe, ITN’s editorial team selected a representative sampling to share throughout the year from a range of the latest conferences and research presented.
Focusing in on the application of deep learning (DL) models in breast screening, detection and risk prediction, what follows is an abbreviated compilation of an education session presented by a panel of esteemed experts during the 2023 Radiological Society of North America Scientific Session and Annual Meeting titled, “Breast Imaging: AI and Deep Learning Applications in Screening and Risk Assessment.”
The much-anticipated program was co-moderated by Manisha Bahl, MD, Director, Breast Imaging Fellowship Program at Mass Gen Brigham, as well as Physician Investigator (Cl) - Radiology, Mass Gen Research Institute, and an Associate Professor of Radiology at Harvard Medical School; Sarah Eskreis-Winkler, MD, PhD, Assistant Attending Radiologist at Memorial Sloan Kettering Cancer Center; and Wendy Burton DeMartini, MD, Associate Chair for Clinical Faculty Affairs, and Professor at Stanford University School of Medicine Department of Radiology, who is the past Division Chief of Breast Imaging.
The panel featured DeMartini, along with five additional abstract presenters in a highly anticipated session which provided some of the most current and significant study findings. Other highly regarded breast imaging specialists on the panel included: Andreas D. Lauritzen, PhD, from the Department of Computer Science at the University of Copenhagen in Denmark; and Leslie Lamb, MD, MSc, Mass Gen Brigham, both of whose findings are included in this summary; as well as Christiane K. Kuhl, MD, PhD, Chair, Department of Diagnostic and Interventional Radiology at University Hospital of Aachen, University of Aachen, RWTH (Germany); Olasubomi Jimmy Omoleye, MBBS, Postdoctoral Scholar at University of Chicago Medicine; and Ray C. Mayo, MD, associate professor in the Department of Breast Imaging, Diagnostic Imaging division, University of Texas MD Anderson Cancer Center.
Five-year Risk Model Study Findings
In presenting “Performance of a Deep Learning Image-Based Five-Year Breast Cancer Risk Model in Predicting DCIS and Invasive Breast Cancer,” DeMartini shared an overview of the research landscape regarding deep learning, noting, “Overall, deep learning AI applied to screening mammograms is promising to improve breast cancer risk assessment. There have now been multiple publications that have demonstrated that deep learning image-based mammography five year risk prediction models have AUCs better than traditional clinical risk models. The AUC is ranging in the 0.63 to 0.72 range. However, most traditional risk models, in addition to other limitations, typically are built to protect only invasive breast cancer, although the Tyrer-Cuzick model does predict both invasive and cancer and/or DCIS. But prediction of both invasive cancer and DCIS is important for the benefits of early detection." She added that there is, to date, limited data on the deep learning models’ performances for invasive cancer versus DCIS, which is what led to the study. The research was part of and performed with the Clairity Research Consortium, which is comprised of contributions from seven global centers representing more than 1.1 million exams, all linked to clinical outcomes.
The study was a retrospective, multicenter international study which included 31,016 consecutive bilateral 2D full field digital screening mammograms from a US screening center, and 10,673 from a European screening center — from January 2011 through December 2016. She explained that these exams were not part of model training. Cancer outcomes and type (pure DCIS vs any invasive breast cancer) were obtained from local tumor registries. Cancer rates were defined as total cancers diagnosed after the index mammogram/total exams and were calculated for DCIS (excluding invasive cancer cases) and invasive cancers (excluding pure DCIS cases). Model performance was compared using areas under the receiver operating characteristic curve (AUCs) (p<.05) with 95% confidence intervals.
The strengths, reported DeMartini, include that this was a multicenter US and European screening study with five-year follow-up, it included tumor registry linkage, and was inclusive of patients who are often excluded from other AI studies or deep learning studies, such as a prior history of breast cancer and implants. There were limitations noted, which included: the study was performed utilizing a single vendor (Hologic); type of mammogram was a 2D digital mammogram; and that it was conducted largely in a white non-Hispanic patient population.
In conclusion, offered DeMartini, the DL based five-year breast cancer risk model performed well with ACS in the 0.73 to 0.80 range, specifically reporting that it performed well for prediction of both invasive cancer and DCIS across multiset centers in the US and Europe. At the US center, the performance was significantly higher for invasive cancer compared to DCIS. Bottom line findings: The deep learning based five-year breast cancer risk prediction can effectively identify the risk of both invasive breast cancers and DCIS in US and European screening programs.
“This certainly warrants further evaluation and subsequent studies … and certainly the clinical implication of this is that we can leverage these tools to contribute to improved stratification of patients for personalized risk reduction and screening recommendations,” said DeMartini.
AI’s Impact on Finding Small Invasive Cancers, Reducing Workload Explored
The highly anticipated session, “Preliminary Results of Implementing AI into Breast Cancer Screening in the Capital Region of Denmark,” was presented by University of Denmark’s Andreas D. Lauritzen, PhD.
Lauritzen detailed the study which assessed the screening quality before and after AI was implemented in a population-based breast cancer screening program. Based on a large prior simulation study, an AI system supporting a stratified reading protocol, and decision support was implemented into screening in the Capital Region of Denmark as of mid-Nov. 2021 to end of Dec. 2022. In the Capital Region of Denmark women aged 50-69 years are screened biennially. For women screened before AI implementation from Oct. 1, 2020, to Nov. 17, 2021, all screens were read independently by two breast radiologists. For women screened with AI from Nov. 18, 2021, to Dec. 31, 2022, all screens were assessed by the AI system Transpara (ScreenPoint Medical) producing an exam score from 1-10 reflecting the probability of breast cancer. Screens with an exam score ≤7 (≤5 before May 3, 2022) were read by AI and a single senior breast radiologist.
He further noted that the remaining women were read by two radiologists with access to decision support with markings by the AI system. Screen-detected breast cancers (invasive or DCIS) were histologically confirmed. Screening quality was measured using recall rate, cancer detection rate (CDR), rate of invasive cancers, rate of small cancers (≤1cm), rate of node-negative cancers, and false-positive (FP) rate. Outcomes were compared before and after AI (jointly for both exam score thresholds) using the Chi2 test. Importantly, the researchers also measured the reading workload reduction.
Results from the study were as follows: 59,573 women were screened before AI and 74,596 women with AI. The recall rate decreased from 3.10% to 2.42% (P < .001) in screening with AI. CDR increased from 0.69% to 0.83% (P < .01). Rate of invasive cancers decreased from 84.8% to 79.9% but not significantly (P = .10). Rate of small cancers increased from 38.9% to 48.0% (P = .04). Rate of node-negative cancers increased from 76.7% to 80.9%, but not significantly (P = .30). FP rate decreased from 2.41% to 1.66% (P < .001). Significantly, reading workload was reduced by 34.2%.
Lauritzen further presented an overview of findings by noting that screening with AI in the Capital Region of Denmark increased CDR while the recall and FP rates decreased. Small invasive cancers were more often diagnosed. Rates of invasive and node-negative cancers did not significantly change. The findings suggest that AI improved screening quality while considerably reducing radiologists’ workload. As such, regarding clinical relevance, he noted that screening with AI safely reduced both reading and clinical workload, and results indicated that invasive cancers were more frequently diagnosed while small, suggesting earlier detection.
In analyzing the research, Lauritzen offered this overview of findings and insights moving forward: “In summary, screening with the AI did considerably decrease the workload which was evident in the amount of reads in the recall rate and the false positive rate and positive predictive value, and also the rate of consensus meetings. In terms of the performance, there seems to be no deterioration of the screening performance when screening with AI. The cancer detection rate and the rate of small cancers might actually suggest that we have a small element of potential earlier detection.”
He noted that there were two important limitations to address, saying: “First of all, we have the slightly longer screening interval in the period of screening with AI, so that might account for a slight increase in the cancer detection as well.” Looking to the clinical implications, he added, “It’s important for all the women and also us that we actually diagnosed more small cancers and the negative rate is stable. And secondly, we have not been screening long enough with AI to actually gather full data on the interval cancers. But that is something that we’re working on and having screened for two years now, we can start looking at interval cancers, so that would be very exciting to see whether it is reflected in the screening sensitivity and specificity.”
Deep Learning's Value in Predicting Risk Across Races
Another important study, “Deep Learning Model Translates Imaging Biomarkers to Predict Future DCIS vs Invasive Breast Cancer Risk Across Races,” was presented by Leslie Lamb, MD, MSc, a diagnostic radiologist at Mass General Brigham and Associate Professor of Radiology at Harvard Medical School. Other MGH colleagues involved in the study included: Constance D. Lehmam, MD, PhD, also a Professor of Radiology at Harvard Medical School and co-founder of Clairity, Inc.; Sarah Mercaldo, PhD; and Mass Gen Data Manager/Analyst Andrew Carney.
The findings revealed that a DL image-only risk model can provide increased access to an equitable accurate risk assessment tool — for both DCIS and invasive malignancy prediction — across races. A snapshot summary of Lamb’s research, one of several recent MGH research team studies into AI/DL, follows.
“In the domain of precision medicine, risk-based screening has been elusive, because we have not been able to accurately identify the patient’s risk of developing breast cancer. Current screening guidelines all leverage traditional risk assessment models, such as Tyrer-Cuzick, Gail and the BCSC. These models were developed to predict the risk of malignancy and inform eligibility for chemo prevention and supplemental screening with MRI. The models have demonstrated moderate performance in predicting future breast cancer, with AUCs ranging from 0.52-0.62 at best, with worse performance in patients of color,” explained Lamb.
She noted that a deep learning algorithm was previously designed to predict a patient’s risk of developing breast cancer at multiple time points using mammographic image biomarkers alone. The purpose of this retrospective, multi-site study, she offered, was to compare the predictive accuracy of a DL image-only model to predict future DCIS vs invasive breast cancer across races.
The study included consecutive patients >30 years undergoing routine bilateral screening mammography (Jan. 10, 2009 to Jan. 10, 2018) at five facilities with at least five years of follow-up. In all, 83,871 bilateral screening mammograms in 48,984 patients met inclusion criteria. Lamb also noted the mean patient age was 59 years of age, and that women with a personal history of breast cancer were excluded. Notably, a DL 5-year model (Hologic) was used to assess risk.
The following results were shared:
• The AUC of DL model in predicting DCIS was 0.71 (95% confidence interval [CI]: 0.65, 0.77) and invasive malignancy was 0.70 (95% CI: 0.67, 0.74) across all patients.
• The AUC in predicting DCIS was significantly higher in Black vs White patients (0.92, 95% CI: 0.87, 0.97 vs 0.70, 95% CI: 0.64, 0.77, respectively, p<0.001).
• There was no evidence of a significant difference in predicting DCIS in Asian vs White patients (0.66, 95% CI: 0.32, 1.00 vs 0.70, 95% CI: 0.64, 0.77, respectively, p=0.798).
• There was no evidence of a significant difference in predicting invasive disease in Black vs White (0.73, 95% CI: 0.63, 0.83 vs 0.71, 95% CI: 0.67, 0.74, respectively, p=0.592) or Asian vs White (0.68, 95% CI: 0.54, 0.83 vs 0.71, 95% CI: 0.67, 0.74, respectively, p=0.988) patients.
Two key findings are worth noting: mammograms contain highly predictive biomarkers of future cancer risk, and a DL model using screening mammography alone can accurately discriminate patients at risk of developing DCIS and invasive disease across races. Lamb also noted that future studies are required to validate these results in larger cohorts of patients of diverse races and ethnicities, and to compare traditional models, which she and the team are currently pursuing.
SIDEBAR:
Preliminary Clinical Impact and Future Work
University of Copenhagen breast imaging specialist Andreas D. Lauritzen, MD, offered these significant findings from a study presented during RSNA23.
Workload:
• Reading workload reduced 33.5%
• Recall rate reduced 20.5%
• Reduced false positives (PPV: 22.6%-34.6%)
• Reduction in disagreements
Screening Performance:
• No deterioration in screening performance
• Potential earlier detection
Find more RSNA conference coverage here
Related Breast Imaging Content:
Single vs. Multiple Architectural Distortion on Digital Breast Tomosynthesis
Today's Mammography Advancements
Digital Breast Tomosynthesis Spot Compression Clarifies Ambiguous Findings
AI DBT Impact on Mammography Post-breast Therapy
ImageCare Centers Unveils PINK Better Mammo Service Featuring Profound AI
Radiologist Fatigue, Experience Affect Breast Imaging Call Backs
Fewer Breast Cancer Cases Between Screening Rounds with 3-D Mammography
Study Finds Racial Disparities in Access to New Mammography Technology