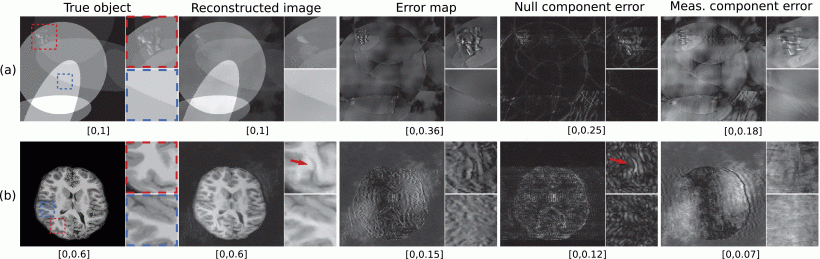
From left-to-right are examples of a true object, a reconstructed estimate of the object produced by use of a U-Net from tomographic measurements, the total error map, the error in the null component of the reconstructed object, and the error in the measurement component of the reconstructed object. The two rows correspond to different objects. In each case, the true object is outside the respective training data distribution of the U-Net and phase noise was added to the measurements prior to image reconstruction. Image courtesy of IEEE Xplore
January 12, 2022 — Machine-learning methods are being actively developed for computed imaging systems like magnetic resonance imaging (MRI). However, these methods occasionally introduce false, unexplainable structures in images, known as hallucinations, that can lead to incorrect diagnoses.
Researchers at the Beckman Institute for Advanced Science and Technology and the Computational Imaging Science Laboratory have defined a mathematical framework for identifying hallucinations, a first step toward reducing their frequency.
This work, “On hallucinations in tomographic image reconstruction,” is published in IEEE Transactions on Medical Imaging in a special issue on machine learning methods for image reconstruction.
Most modern medical imaging devices — such as MRI, computed tomography (CT) and PET — do not record images directly. Instead, they employ a computational procedure known as image reconstruction. Researchers worldwide are seeking to develop improved image reconstruction methods that use deep learning to circumvent the limitations of traditional methods. Deep learning, like all machine-learning methods, allows algorithms to self-improve by learning from characterized datasets. Advanced DL-based methods can reduce imaging scan times and radiation doses. Although DL-based image reconstruction methods hold great potential for such purposes, they sometimes produce images that appear plausible, but contain false structures that may confound a medical diagnosis.
“This work is an excellent example of how our research group is making foundational contributions to the rapidly evolving field of imaging science,” said Mark Anastasio, the principal investigator of this study and head of the Department of Bioengineering at the University of Illinois Urbana-Champaign.
“Because our framework for analyzing hallucinations in biomedical images will enable researchers to quantitatively assess their image reconstruction methods in new and meaningful ways, we expect the impact of our study to be large."
Lead authors Varun Kelkar and Sayantan Bhadra, both graduate students in Anastasio’s group, anticipate that hallucination maps will help researchers and radiologists identify hallucinations and assess how detrimental they might be. Ultimately, this leads to a deeper understanding of how DL reconstruction methods should be trained to prevent patient misdiagnosis.
“An effective reconstruction method is based on understanding the physics of the imaging system, but also uses realistic assumptions about how an object should appear,” Bhadra said. “DL-based image reconstruction methods are about designing the interplay of these elements by … training deep neural networks on large databases of previously existing clinical images.”
Though DL-based image reconstruction methods are gaining popularity in the field, evidence suggests that they may be unstable, meaning small changes to collected measurements could cause large differences in the resulting image. These challenges are exacerbated when image sampling is incomplete (in other words, when fewer raw imaging measurements are collected to reduce time and costs).
“Although DL has been shown to be useful in many contexts, there’s a lot going on inside these methods that are … still viewed as a black box by many researchers,” Bhadra said. “That makes it hard to tell when a medical abnormality might actually be a false structure created by the machine-learning method. The novelty of our work is in mathematically defining those false structures, which we call hallucinations.”
Kelkar, Bhadra, and colleagues provide a formal definition of hallucinations based on fundamental principles of imaging science. Through numerical studies, they evaluated the presence and impact of hallucinations across both DL-based and non-data-driven reconstruction methods. These methods were used to reconstruct images of adult and pediatric brain tissue — with the DL-based method trained only on adult brain images — and evaluate the effect of bias from the training images.
“Our goal in mathematically defining hallucinations was to separate sources of systemic error, like measurement errors or noise, from errors that arise due to inaccurate assumptions about the to-be-imaged subject that are built into the image reconstruction method,” Kelkar said.
The impact of a generated hallucination is highly dependent on the type of tissue being imaged and the disease being monitored. Sophisticated image processing methods can be applied to identify how a particular diagnostic task may be affected by the presence of hallucinations.
“A hallucination could be something minor, such as a slight imperfection at the boundary of a tissue, or it could be something major, like introducing an entire fold of tissue in the brain,” Kelkar said.
For more information: www.beckman.illinois.edu/