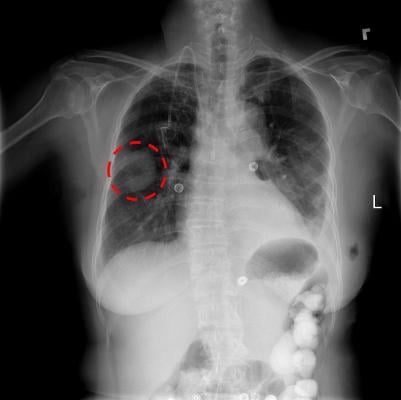
January 23, 2019 — An artificial intelligence (AI) system can interpret and prioritize abnormal chest X-rays with critical findings, according to a study appearing in the journal Radiology.1 This could potentially reduce the backlog of exams and bringing urgently needed care to patients more quickly.
Chest X-rays account for 40 percent of all diagnostic imaging worldwide. The number of exams can create significant backlogs at healthcare facilities. In the U.K. there are an estimated 330,000 X-rays at any given time that have been waiting more than 30 days for a report.
"Currently there are no systematic and automated ways to triage chest X-rays and bring those with critical and urgent findings to the top of the reporting pile," said study co-author Giovanni Montana, Ph.D., formerly of King's College London in London and currently at the University of Warwick in Coventry, England.
Deep learning (DL), a type of AI capable of being trained to recognize subtle patterns in medical images, has been proposed as an automated means to reduce this backlog and identify exams that merit immediate attention, particularly in publicly-funded healthcare systems.
For the study, Montana and colleagues used 470,388 adult chest X-rays to develop an AI system that could identify key findings. The images had been stripped of any identifying information to protect patient privacy. The radiologic reports were pre-processed using natural language processing (NLP), an algorithm of the AI system that extracts labels from written text. For each X-ray, the researchers' in-house system required a list of labels indicating which specific abnormalities were visible on the image.
"The NLP goes well beyond pattern matching," Montana said. "It uses AI techniques to infer the structure of each written sentence; for instance, it identifies the presence of clinical findings and body locations and their relationships. The development of the NLP system for labeling chest X-rays at scale was a critical milestone in our study."
The NLP analyzed the radiologic report to prioritize each image as critical, urgent, non-urgent or normal. An AI system for computer vision was then trained using labeled X-ray images to predict the clinical priority from appearances only. The researchers tested the system's performance for prioritization in a simulation using an independent set of 15,887 images.
The AI system distinguished abnormal from normal chest X-rays with high accuracy. Simulations showed that critical findings received an expert radiologist opinion in 2.7 days, on average, with the AI approach — significantly sooner than the 11.2-day average for actual practice.
"The initial results reported here are exciting as they demonstrate that an AI system can be successfully trained using a very large database of routinely acquired radiologic data," Montana said. "With further clinical validation, this technology is expected to reduce a radiologist's workload by a significant amount by detecting all the normal exams so more time can be spent on those requiring more attention."
The researchers plan to expand their research to a much larger sample size and deploy more complex algorithms for better performance. Future research goals include a multi-center study to prospectively assess the performance of the triaging software.
"A major milestone for this research will consist in the automated generation of sentences describing the radiologic abnormalities seen in the images," Montana said. "This seems an achievable objective given the current AI technology."
For more information: www.pubs.rsna.org/journal/radiology
Reference
1. Annarumma M., Withey S.J., Bakewell R.J., et al. Automated Triaging of Adult Chest Radiographs with Deep Artificial Neural Networks. Radiology, Jan. 22, 2019. https://doi.org/10.1148/radiol.2018180921