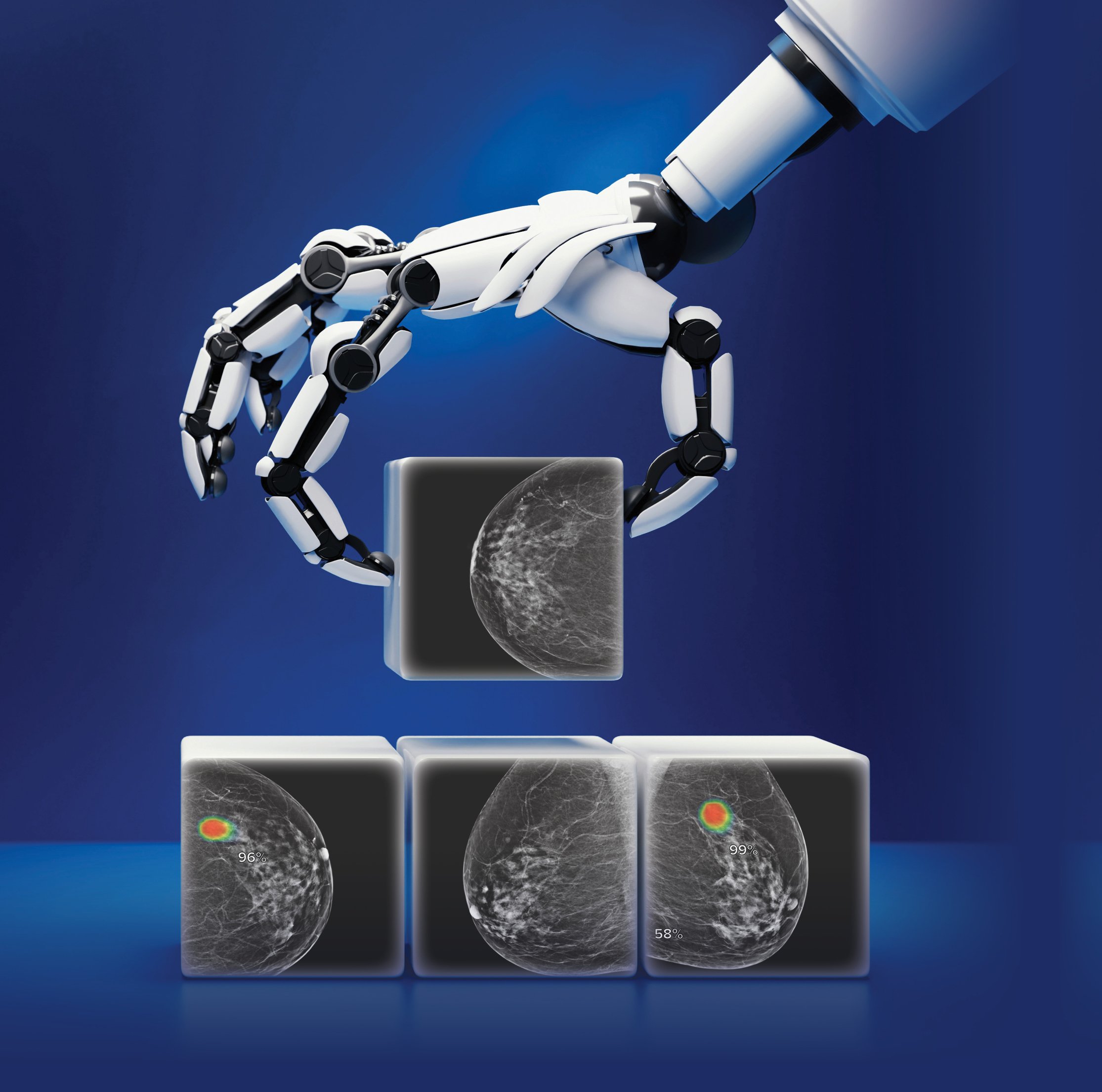
Findings from a clinical trial that used artificial intelligence (AI) in an effort to reduce false positives on breast ultrasound were presented by Linda Moy, MD, Center for Advanced Imaging Innovation and Research with NYU Langone Health Center for Advanced Imaging Innovation and Research (CAI2R), during RSNA 2021. Moy, a leader in radiology AI, is also a professor of radiology at NYU Grossman School of Medicine and a member of Perlmutter Cancer Center.
Led by researchers from the Department of Radiology at NYU Langone Health and its Laura and Isaac Perlmutter Cancer Center, the team’s AI analysis is believed to be the largest of its kind.
In addition to Moy, who served as study co-investigator, the study was conducted by the following team: Senior investigator Krzysztof J. Geras, PhD, co-lead investigators Yiqiu “Artie” Shen, Farah Shamout and Jamie Oliver; and co-investigators Jan Witowski, Kawshik Kannan, Jungkyu Park, Nan Wu, Connor Huddleston, Stacey Wolfson, Alexandra Millet, Robin Ehrenpreis, Divya Awal, Cathy Tyma, Naziya Samreen, Yiming Gao, Chloe Chhor, Stacey Gandhi, Cindy Lee, Sheila Kumari- Subaiya, Cindy Leonard, Reyhan Mohammed, Christopher Moczulski, Jaime Altabet, James Babb, Alana Lewin, Beatriu Reig and Laura Heacock.
The study, published in the journal Nature Communications (Sept. 24, 2021), was supported by the U.S. National Science Foundation (NSF), offered this overview:
Researchers working on an initiative supported by the U.S. National Science Foundation trained AI to identify breast cancer using data obtained from previously conducted ultrasounds. The AI tool significantly increased accurate diagnoses.
“If our efforts to use machine learning as a triaging tool for ultrasound studies prove successful, ultrasound could become a more effective tool in breast cancer screening, especially as an alternative to mammography, and for those with dense breast tissue,” said Moy. “Its future impact on improving women’s breast health could be profound,” she added. The study summary is presented here.
Breast ultrasound images show cancer (at left, as dark spot in center and, at right, in red, as highlighted by a computer). Image courtesy of Nature Communications
Abstract:
Ultrasound is an important imaging modality for the detection and characterization of breast cancer. Though consistently shown to detect mammographically occult cancers, breast ultrasound has been noted to have high false-positive rates.
In this work, AI system that achieves radiologist-level accuracy in identifying breast cancer in ultrasound images is presented.
Developed on 288,767 exams, consisting of 5,442,907 B-mode and color Doppler images, the AI achieves an area under the receiver operating characteristic curve (AUROC) of 0.976 on a test set consisting of 44,755 exams. In a retrospective reader study, the AI achieves a higher AUROC than the average of ten board-certified breast radiologists (AUROC: 0.962 AI, 0.924 ± 0.02 radiologists). With the help of the AI, radiologists decrease their false positive rates by 37.3% and reduce requested biopsies by 27.8%, while maintaining the same level of sensitivity. This highlights the potential of AI in improving the accuracy, consistency and efficiency of breast ultrasound diagnosis.
Materials and Methods — Model
• Developed an AI system using a DCNN trained on a Globally-Aware Multiple Insurance Classifier
• Weakly supervised model that automatically identified malignant and benign lesions without requiring manual annotations from radiologists
• Pathology was used as the reference standard
• Details ib data pre-processing, labeling, annotation and ground truth
• Dataset was split on patient level into training (60%), validation (10%) and test databases (30%).
NYU Breast Ultrasound Dataset
• AI system was trained using internal dataset of 288,767 ultrasound exams with 5,442,907 total images acquired from 143,203 patients between 2012-2019
• 20 imaging centers that perform screening and diagnostic ultrasound exams
• 28,914 of these exams were associated with at least one biopsy procedure
• 5,593 of which had biopsies yielded malignant findings.
Results
• On a test set of 44,755 exams, the AI system achieved an AUC of 0.976 for identifying exams with malignancies
• Among the 663 reader study exams, the AI system had an AUC of 0.962, outperforming the average of ten radiologists (0.924 +/- 0.02). p<0.001
• At the average radiologist’s sensitivity (90.1%), the AI system had a higher specificity (85.6% vs. 80.7%, p<0.001)
• The AI system recommended fewer biopsies (19.8% vs. 24.3%) p<0.001.
Reader Study — Hybrid Model
• The hybrid models improved radiologist’s AUC from 0.929 to 0.960
• At the radiologist’s sensitivity levels, the hybrid models:
• Increased radiologist’s average specificity from 80.7% to 88.4% (p<0.001)
• Increased radiologist’s PPV from 27.1% to 39.2% (p<0.001
• The hybrid models decreased the average biopsy rate from 24.3% to 17.2% (p<0.001)
• The reduction in biopsies using the hybrid models represented 29.4% of all recommended biopsies.
Conclusion
• The AI system detected and diagnosed cancer on breast ultrasound with accuracy that exceeds that of experienced board-certified radiologists
• AI decision support decreased unnecessary biopsies
• The hybrid decision-making models may potentially enhance the performance of breast imagers without the added cost of a second human reader
• The system could be harnessed to support decision-making where there are shortages of radiologists.
The study’s conclusion offered the researcher’s perspective on future clinical applications and the impact of artificial intelligence tolls on the effort to improve the accuracy of breast cancer imaging.
In it, the authors offered this on their findings:
“In conclusion, we examined the potential of AI in U.S. exam evaluation. We demonstrated in a reader study that deep learning models trained with a sufficiently large amount of data are able to produce diagnosis as accurate as experienced radiologists. We further showed that the collaboration between AI and radiologists can significantly improve their specificity and obviate 27.8% of requested biopsies. We believe this research could supplement future approaches to breast cancer diagnosis. In addition, the general approach employed in our work, mainly the framework for weakly supervised classification and localization, may enable utilization of deep learning in similar medical image analysis tasks.”
SIDEBAR:
Artificial Intelligence System for Automated Triage of Breast Ultrasound Exams
Following is a clinical snapshot of a second study presented by Linda Moy, MD, during the RSNA 2021 session: “Breast Imaging: Advanced Breast Ultrasound.”
Authors included Jamie Oliver, BA, Beatrice Reig, MD, MPH, Yiming Gao, MD, Alan Lewin, MD, Linda Moy, MD, Laura Heacock, MD.
Hypothesis: A DL model trained to triage breast ultrasound exams as cancer-free can improve radiologist efficiency and specificity without compromising the sensitivity.
Purpose: To train an AI system to triage breast exams with the goal of reallocating radiologists’ time towards exams with high suspicion of malignancy.
Materials and Methods — Dataset
AI system was trained using an internal dataset of 288,767 ultrasound exams with 5,442,907 total images acquired from 143,203 patients between 2012-2019.
• 20 imaging centers that perform screening and diagnostic ultrasound exams
• 28,914 of these exams were associated with at least one biopsy procedure
• 5,593 of which had biopsies yielded malignant findings
Results
• On a test of 44,755 exams, the AI system achieved an AUC of 0.96 identifying exams with malignant lesions
• When the triage system evaluated 3,553 exams which originally assessed as B1-RADS 3, it reclassified 60%, 70%, and 80% of exams with the lowest AI scores as benign without missing any malignancies.
• AI system may obviate the need for follow-up imaging
Discussion
• Using a high sensitivity threshold, our DL model may function as a standalone system
• Triage 60-80% of breast ultrasound exams from the radiologist worklist, with a false-negative rate of 0.008-0.03%
• Using a high sensitivity threshold, our DL model placed 978 (2.2%) exams into an enhanced assessment workflow, with high PPV of 69.6%
Clinical Relevance
• AI decision support decreased unnecessary biopsies and follow-up exams
• The system could be harnessed to support decision-making where there are shortages of radiologists.