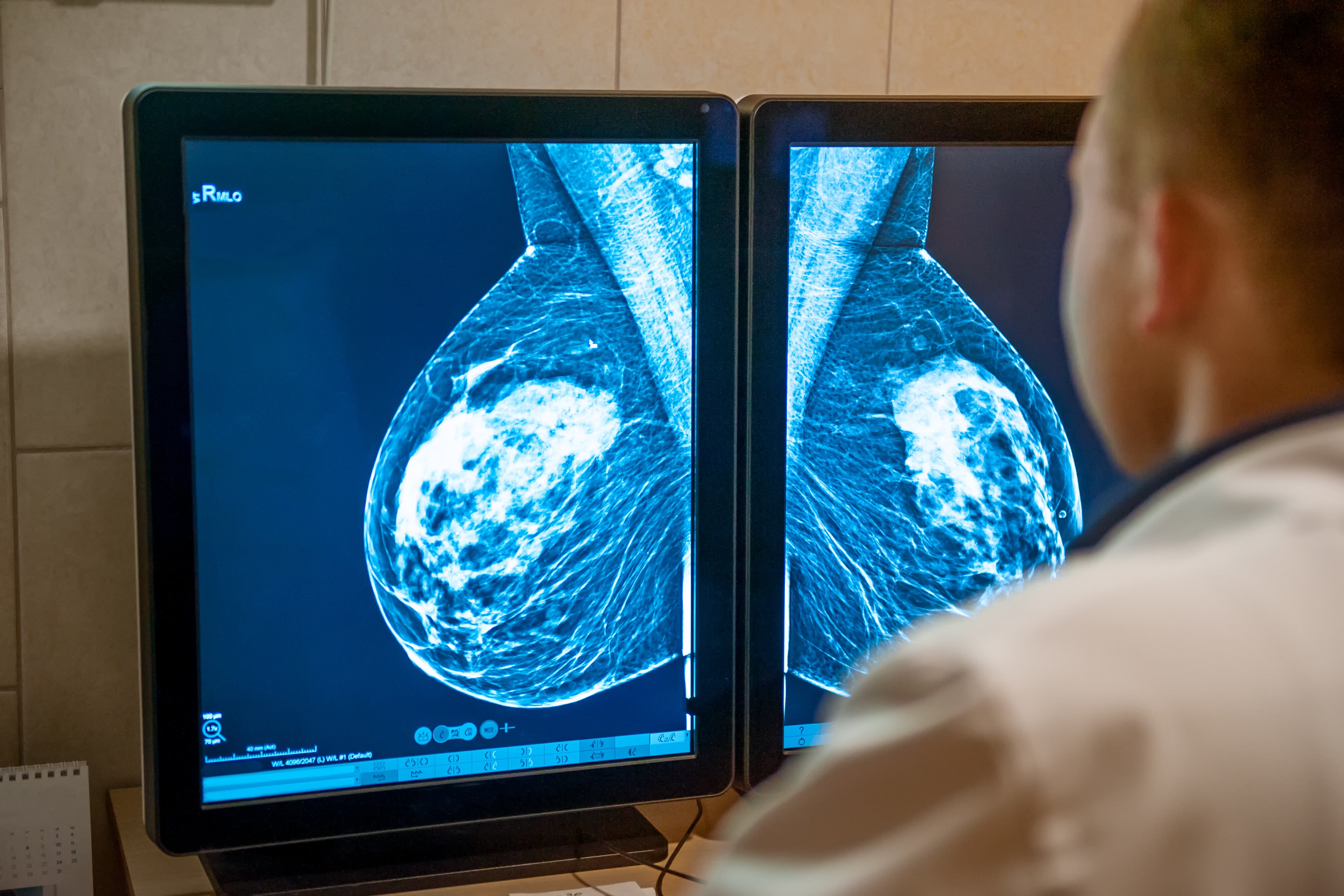
Contrary to what many people believe, artificial intelligence (AI) has played a role in breast screening technology for a number of years. Hologic, for example, has been leveraging AI in device development as early as 2006, when it trained its first piece of technology to classify a patient’s breast density through breast tissue pattern and texture analysis. More than a decade later, AI has continued to progress. The benefit of time has not only allowed R&D professionals to learn more about the technology, but has also allowed the AI technology to become more precise thanks to the growing body of images in the database. The industry has carefully watched AI’s technological evolution, slowly showing its support as more benefits are realized, and today we’re seeing many radiologists lean in and embrace AI with open arms.
AI’s Impact on Breast Cancer Screening
During the 2018 Radiological Society of North America (RSNA) annual meeting, Hologic polled more than 300 attendees, including radiologists and radiology administrative professionals, and found that all participants agreed that there is an application for AI in breast cancer screening. Additionally, respondents indicated that AI will have the greatest impact in the future on personalized screening pathways through the creation of new risk models. While AI very much has a present-day application, it’s clear that its greatest impact is still yet to come as we strive to deliver more personalized care.
Currently, as we all know, there are standard breast screening guidelines for the “average risk” patient without any known risk factors like a family history of breast cancer and increasing patient age. These are two of the biggest risk factors among building a unique profile indicating how “at-risk” a patient really is, and all factors combined can subsequently influence an individual’s screening protocol — including the age she starts, frequency and even the imaging modality for screening.
Breast density is a specific example of a risk factor where AI is particularly useful for determining a patient’s screening pathway. Women with very dense breasts are four to five times more likely to develop breast cancer than women with less dense breasts, making it important to be able to classify each patient’s density.1,2 Doing so requires the patient to get a mammogram, though. Without AI, radiologists must visually assess the images from the exam to determine a woman’s breast density using the Breast Imaging Reporting and Data System (BI-RADS) founded by the American College of Radiology (ACR) in 1993. Utilizing AI technology, however, removes any subjectivity associated with this manual process by automatically categorizing each patient’s density based on the thousands of breast images that it is trained by radiologists to know. As a result, radiologists can more confidently provide the most appropriate screening plan for each individual moving forward.
Aside from breast density, it has also been suggested in more recent studies that parenchymal texture features, which are measures of the local distribution of the parenchymal tissue, may also provide complementary information regarding an individual woman’s risk for breast cancer.3,4 This is yet another example of how risk stratification is being used, for which AI can offer assistance.
AI and Risk Assessment
AI will continue to play a significant role in risk stratification and risk assessment to drive more personalized patient care and outcomes. Patient history, family history, evidence-based clinical conditions, image-based phenotypes (e.g. texture/pattern/AI based features) and many more such attributes and innovations will further augment and strengthen the personalized health profile assessment along with risk models. There are countless possibilities to impact the future of global healthcare and our journey in AI is the first big step toward breakthrough that will change the landscape of personalized medicine. It’s not a matter of “if,” it’s a matter of when.
Samir Parikh is the global vice president of research and development for Hologic. In this role, he is responsible for leading and driving innovative advanced solutions across the continuum of care to drive sustainable growth of the Breast and Skeletal Health Division.
References:
1. Boyd NF, Guo H, Martin LJ, et al. Mammographic density and the risk and detection of breast cancer. N Engl J Med. 356(3):227-36, 2007.
2. Yaghjyan L, Colditz GA, Collins LC, et al. Mammographic breast density and subsequent risk of breast cancer in postmenopausal women according to tumor characteristics. J Natl Cancer Inst. 103(15):1179-89, 2011.
3. H. Li et al., “Computerized texture analysis of mammographic parenchymal patterns of digitized mammograms,” Acad. Radiol. 12(7), 863–873 (2005).
4. Manduca et al., “Texture features from mammographic images and risk of breast cancer,” Cancer Epidemiol. Biomarkers Prev. 18(3), 837–845 (2009).