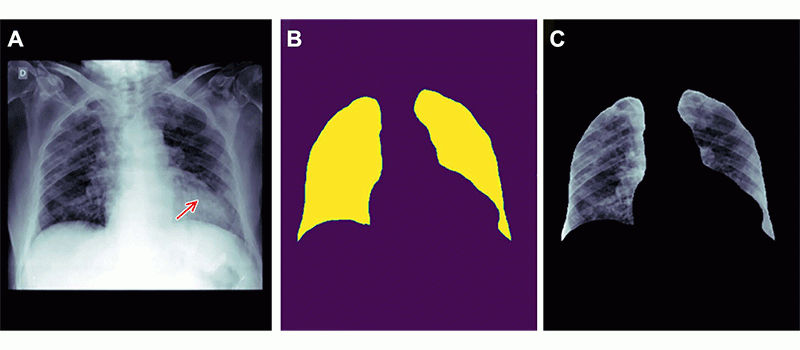
Example of how improper feature removal from imaging data may lead to bias. (A) Chest radiograph in a male patient with pneumonia. (B) Segmentation mask for the lung, generated using a deep learning model. (C) Chest radiograph is cropped based on the segmentation mask. If the cropped chest radiograph is fed to a subsequent classifier for detecting consolidations, the consolidation that is located behind the heart will be missed (arrow, A). This occurs because primary feature removal using the segmentation model was not valid and unnecessarily removed the portion of the lung located behind the heart.
https://doi.org/10.1148/ryai.210290 © RSNA 2022
August 26, 2022 — With the increasing use of artificial intelligence (AI) in radiology, it is critical to minimize bias within machine learning systems before implementing their use in real-world clinical scenarios, according to a special report published Wednesday, August 24, in the journal Radiology: Artificial Intelligence, a journal of the Radiological Society of North America (RSNA).
The report, the first in a three-part series, outlines the suboptimal practices used in the data handling phase of machine learning system development and presents strategies to mitigate them.
“There are 12 suboptimal practices that happen during the data handling phase of developing a machine learning system, each of which can predispose the system to bias,” said Bradley J. Erickson, M.D., Ph.D., professor of radiology and director of the AI Lab at the Mayo Clinic, in Rochester, Minnesota. “If these systematic biases are unrecognized or not accurately quantified, suboptimal results will ensue, limiting the application of AI to real-world scenarios.”
Dr. Erickson said the topic of proper data handling is gaining more attention, yet guidelines on the correct management of big data are scarce.
“Regulatory challenges and translational gaps still hinder the implementation of machine learning in real-world clinical scenarios. However, we expect the exponential growth in radiology AI systems to accelerate the removal of these barriers,” Dr. Erickson said. “To prepare machine learning systems for adoption and clinical implementation, it’s critical that we minimize bias.”
Within the report, Dr. Erickson and his team suggest mitigation strategies for the 12 suboptimal practices that occur within the four data handling steps of machine learning system development (three for each data handling step), including:
Data collection—improper identification of the data set, single source of data, unreliable source of data
Data investigation—inadequate exploratory data analysis, exploratory data analysis with no domain expertise, failing to observe actual data
Data splitting—leakage between data sets, unrepresentative data sets, overfitting to hyperparameters
Data engineering—improper feature removal, improper feature rescaling, mismanagement of missing data
Dr. Erickson said medical data is often far from ideally suited as input for machine learning algorithms.
“Each of these steps could be prone to systematic or random biases,” he said. “It’s the responsibility of developers to accurately handle data in challenging scenarios like data sampling, de-identification, annotation, labeling, and managing missing values.”
According to the report, careful planning before data collection should include an in-depth review of clinical and technical literature and collaboration with data science expertise.
“Multidisciplinary machine learning teams should have members or leaders with both data science and domain (clinical) expertise,” he said.
To develop a more heterogeneous training dataset, Dr. Erickson and his coauthors suggest collecting data from multiple institutions from different geographical locations, using data from different vendors and from different times, or including public datasets.
“Creating a robust machine learning system requires researchers to do detective work and look for ways in which the data may be fooling you,” he said. “Before you put data into the training module, you must analyze it to ensure it’s reflective of your target population. AI won’t do it for you.”
Dr. Erickson said that even after excellent data handling, machine learning systems can still be prone to significant biases. The second and third reports in the Radiology: Artificial Intelligence series focus on biases that occur in the model development and model evaluation and reporting phases.
“In recent years, machine learning has demonstrated its utility in many clinical research areas, from reconstructing images and hypothesis testing to improving diagnostic, prognostic and monitoring tools,” Dr. Erickson said. “This series of reports aims to identify erroneous practices during machine learning development and mitigate as many of them as possible.”
For more information: www.rsna.org