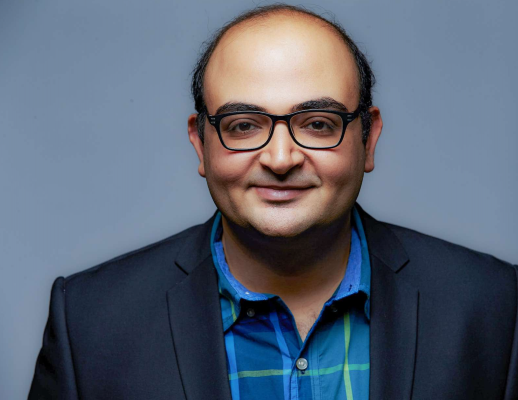
A new study from Mass General Brigham has found that large foundation models that incorporate a richer level of details may mitigate disparities between different demographic groups and enhance model security. Corresponding author Faisal Mahmood, PhD, of the Division of Computational Pathology in the Department of Pathology at Mass General Brigham referred to the findings as a "call to action" for scientists and regulators to utilize diverse data sets in research to benefit all patient groups. Image courtesy: Mass General Brigham
May 2, 2024 — A new study from Mass General Brigham has found that large foundation models that incorporate a richer level of details may mitigate disparities between different demographic groups and enhance model security. A detailed statement issued by MGH today on the study, “Demographic bias in misdiagnosis by computational pathology models,” reported findings of substantial variability in pathology AI models’ performance based on race, insurance type and age group, serving as a “call to action” to researchers and regulators to improve medical equity.
According to the authors, advanced artificial intelligence (AI) systems have shown promise in revolutionizing the field of pathology through transforming the detection, diagnosis, and treatment of disease; however, the under-representation of certain patient populations in pathology datasets used to develop AI models may limit the overall quality of their performance and widen health disparities. Findings, published on UNI and CONCH on April 19 in the journal Nature Medicine, emphasize the need for more diverse training datasets and demographic-stratified evaluations of AI systems to ensure all patient groups benefit equitably from their use.
This new study led by investigators from Mass General Brigham highlights that standard computational pathology systems perform differently — depending on the demographic profiles associated with histology images, but that larger “foundation models” can help partly mitigate these disparities.
“There has not been a comprehensive analysis of the performance of AI algorithms in pathology stratified across diverse patient demographics on independent test data,” said corresponding author Faisal Mahmood, PhD, of the Division of Computational Pathology in the Department of Pathology at Mass General Brigham/Harvard. He added, “This study, based on both publicly available datasets that are extensively used for AI research in pathology and internal Mass General Brigham cohorts, reveals marked performance differences for patients from different races, insurance types, and age groups. We showed that advanced deep learning models trained in a self-supervised manner known as ‘foundation models’ can reduce these differences in performance and enhance accuracy.”
A Call to Action for Physician-Scientists Leveraging AI
The emergence of AI tools in medicine has the potential to positively reshape the delivery of care. It is imperative to balance the innovative potential of AI with a commitment to quality and safety, according to the authors in the written statement. It further added that Mass General Brigham is leading the way in responsible AI, conducting rigorous research on new and emerging technologies to inform the incorporation of AI in medicine.
“Overall, the findings from this study represent a call to action for developing more equitable AI models in medicine,” Mahmood said. “It is a call to action for scientists to use more diverse datasets in research, but also a call for regulatory and policy agencies to include demographic-stratified evaluations of these models in their assessment guidelines before approving and deploying them, to ensure that AI systems benefit all patient groups equitably.”
Study Details
Details presented by MGH researchers about the study follow:
Based on data from the widely used Cancer Genome Atlas and EBRAINS brain tumor atlas, which predominantly include data from white patients, the researchers developed computational pathology models for breast cancer subtyping, lung cancer subtyping, and glioma IDH1 mutation prediction (an important factor in therapeutic response).
When the researchers tested the accuracy of these models using histology slides from over 4,300 patients with cancer at Mass General Brigham and the Cancer Genome Atlas, and stratified the results by race, they found that the models performed more accurately in white patients than Black patients. The models the team tested for subtyping breast and lung cancers and predicting IDH1 mutation in glioma found respective disparities of 3.7, 10.9, and 16 percent in producing correct classifications.
The researchers sought to reduce the observed disparities with standard machine learning methods for bias-mitigation, such as emphasizing examples from underrepresented groups during model training; however, these methods only marginally decreased the bias. Instead, disparities were reduced by using self-supervised foundation models, which are an emerging form of advanced AI trained on large datasets to perform a wide range of clinical tasks. These models encode richer representations of histology images that may reduce the likelihood of model bias.
Despite the observed improvements, gaps in performance were still evident, which reflects the need for further refinement of foundation models in pathology. Furthermore, the study was limited by small numbers of patients from some demographic groups. The researchers are pursuing ongoing investigations of how multi-modality foundation models, which incorporate multiple forms of data, such as genomics or electronic health records, may improve these models.
Mass General Brigham authors include co-first authors Anurag Vaidya, Richard Chen, and Drew Williamson, as well as Andrew Song, Guillame Jaume, Ming Lu, Jana Lipkova, Muhammad Shaban, and Tiffany Chen (MGB Department of Pathology).
The Mass General Brigham statement reported that the work was supported in part through funding by the Brigham and Women’s Hospital (BWH) President’s Fund, BWH and Massachusetts General Hospital Pathology, and National Institute of General Medical Sciences (R35GM138216) to Dr. Mahmood. Mass General Brigham is one of the nation’s leading biomedical research organizations with several Harvard Medical School teaching hospitals.
The Mahmood Lab at the Brigham and Women's Hospital, which just marked its 5th year, aims to utilize machine learning, data fusion and medical image analysis to develop streamlined workflows for cancer diagnosis, prognosis and biomarker discovery, according to an overview from MGH which further offers that the team is interested in developing automated and objective mechanisms for reducing interobserver and intraobserver variability in cancer diagnosis using artificial intelligence as an assistive tool for pathologists. The lab also focuses on the development of new algorithms and methods to identify clinically relevant morphologic phenotypes and biomarkers associated with response to specific therapeutic agents. The staff members develop multimodal fusion algorithms for combining information from multiple imaging modalities, familial and patient histories and multi-omics data to make more precise diagnostic, prognostic and therapeutic determinations.
Paper cited: Vaidya, A et al. “Demographic bias in misdiagnosis by computational pathology models” Nature Medicine DOI: 10.1038/s41591-024-02885-z
More information: www.massgeneralbrigham.org