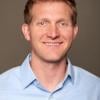
Tyler Blackwell is a board-certified medical physicist with extensive clinical experience in radiation therapy. He is active in several AAPM committees, has served as secretary-treasurer for the Northwest Chapter of AAPM, and is an ABR orals examiner.
BLOG: Auto-Segmentation in Radiation Oncology: Things to Consider
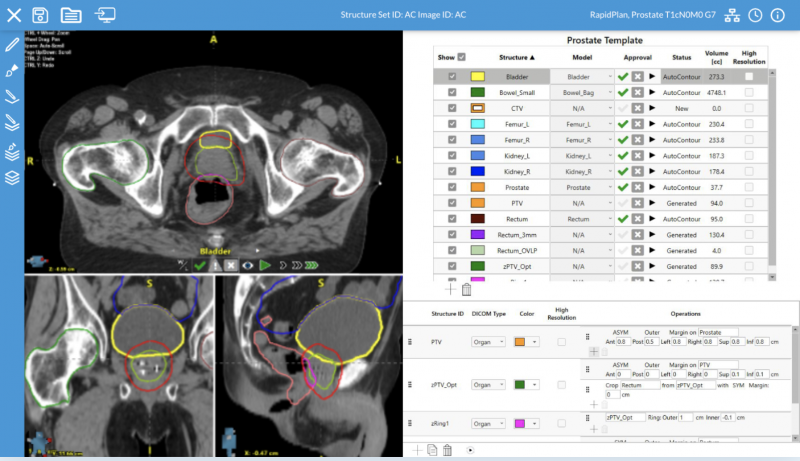
Automation is transforming the way we practice radiation oncology and advancing the standard of patient care. There have been major improvements, both in workflow and quality of treatment, as a direct result of automating various portions of the care path.
Contouring is a task that lends itself well to automation; it’s repetitive and tedious, yet requires skilled technical staffing. Often, critical OAR structures may be incompletely drawn or left out altogether to speed up this process, while manual contour errors may be missed due to user fatigue and an inefficient manual review process. So while auto-segmentation tools have been available for many years, widespread adoption of atlas-based solutions has been hindered by relatively high implementation costs and sub-par clinical results.
Recently, there is renewed excitement for auto-segmentation. The introduction of artificial intelligence and deep learning algorithms has allowed for more accessible, higher-quality, and consistent contours. With artificial intelligence (AI) coming to the fore, it’s important to understand what’s involved in designing clinical tools and what to consider when evaluating various solutions for implementation.
Design Philosophy
While there is plenty of enthusiasm when it comes to AI and automation, it’s prudent to apply some scrutiny to the technology before wading into the deep end. We need to integrate new technologies in a way that ensures that clinicians — with irreplaceable clinical experience — remain front and center. Instead of removing humans from the equation, automation best serves clinical goals when it allows skilled professionals to apply their skills more efficiently. By simplifying routine and mundane tasks, automation paves the way for structured time savings, freeing trained staff to focus on higher-priority responsibilities that require human judgment.
Software designers should pay close attention to reducing cognitive overhead, or the amount of effort required to understand the information presented. Time and effort directed toward mastering an unnecessarily complex automation tool or interpreting unclear results is counterproductive. The best workflows reduce the amount of processing, imports/exports, clicks, and extraneous manual input from the user.
But still, putting clinical judgment to use is essential. Ideally, new tools incorporating automation are simple and easy to use without facilitating an over-reliance on the technology, enhancing clinician input as opposed to replacing it. Vendors have to carefully consider a design philosophy that provides efficiency and quality gains but also incorporates human review and input. Without the involvement of clinical experts, there is a risk of propagating errors in a systematic fashion. In the context of AI contouring, this is best achieved by incorporating or even requiring a standardized, documented review process for auto-generated structures.
What to Consider When Deciding on an AI Contouring Workflow
With AI contouring now gaining significant traction in the clinic, there are several items worth considering when deciding on which commercial tool best suits your department:
Time savings: There is a growing body of research1,2 that has quantified time savings for different anatomical regions. Time savings varies on the individual organ models but is significant across the board. Furthermore, compared to atlas-based options, AI solutions typically involve dramatically less up-front configuration to get started.
Workflow: With various options available, it’s important to understand how any given solution integrates with your clinical workflow. What types of images can be used for contours? Are any exports or imports required? If the tools are to be used by both dosimetrists and radiation oncologists, an intuitive and user-friendly interface is key.
Accuracy: The goal of auto-segmentation is to generate reliable, accurate contours in less time. So it’s fair to question: does it produce clinically useful contours most of the time? To go further, do the contours created by the models align with your clinical preferences? Fewer edits and inputs equate to more time savings.
Overcoming limitations: Even with the large improvements to AI contour models in the past few years, auto-generated outputs aren’t always perfect due to extremes in patient anatomy and setup. For those contours that require more attention, does the software allow for comprehensive (but efficient) review and editing?
Standardization: Variability in contours among planners is well-documented,3 and discrepancies in structure volumes can potentially result in large deviations in dose delivery. ASTRO has developed initiatives and resources to encourage clinics to converge in delineation practices.4 Does the software help to provide consistency in structures across all planners and physicians to achieve higher levels of standardization?
Commercial partnership: Deep-learning for auto-contouring is an emerging development and has plenty of room for growth and improvement. Has the commercial partner demonstrated they are capable of rapid quality improvement and a willingness to incorporate critical feedback? AI structure models built based on one clinic’s contour needs, may not match another’s.
Clearly, there is nuance involved in the adoption of new clinical technologies. But ultimately, there are demonstrated and tangible benefits to incorporating AI to streamline contouring in radiation therapy. Time savings can reveal new opportunities to provide value on high-impact clinical activities, and accelerating the treatment planning process translates to shortening the simulation-to-treatment timeline. Automating structure generation also creates a scaffolding for consistency and standardization. For these reasons, among many others, auto-segmentation is seeing a clinical renaissance in the age of AI.
Learn more about how your department can benefit from faster, automated contours at Radformation.com.
References:
1: https://doi.org/10.1016/j.radonc.2019.10.019
2: https://doi.org/10.1002/mp.13200