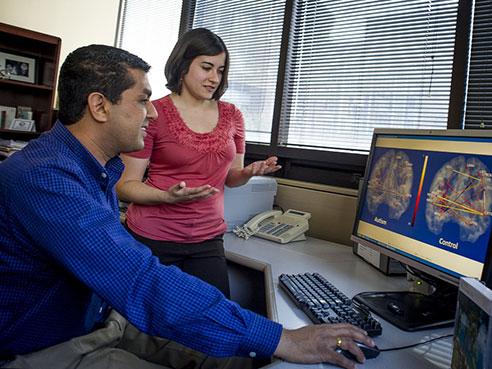
Rajesh Kana, Ph.D., and Lauren Libero, Ph.D., utilized structural MRI to analyze three factors that could lead to earlier diagnosis of autism spectrum disorder. Image courtesy of the University of Alabama at Birmingham.
March 9, 2015 — Rajesh Kana, Ph.D., of the University of Alabama at Birmingham, and colleagues are the first to combine three different measures of the brain to distinguish people with autism spectrum disorder (ASD) from matched, typically developing peers. This multimodal approach, published online in the journal Cortex, uses anatomy, the connectivity between different brain regions and levels of a neurochemical for measurement; this is distinct from many previous studies that have used a single neuroimaging measure. While those studies uncovered widespread functional and anatomical brain abnormalities in ASD, the results were not highly consistent, possibly reflecting the complex brain pathology in autism spectrum disorders.
At this time, autism diagnosis is based on behavior. Kana’s multimodal neuroimaging-based classification is a step toward a possible biomarker for autism and possibly diagnosing autism at an early age, perhaps as early as 6 months, when the brain is very plastic and intervention might be more effective. “But that’s a long, long way off,” said Kana, an associate professor in the Department of Psychology in the UAB College of Arts and Sciences and an associate scientist in UAB’s Civitan International Research Center.
This preliminary study needs to be validated with a larger sample, Kana said; but it “emphasizes that the brain abnormalities in autism may not be confined to a single area. Rather, they are distributed across different areas at multiple levels and layers.”
Kana, corresponding author of the study, examined 19 high-functioning adults with ASD and 18 typically developing peers, who were matched for age and intelligence. Using the 3-Tesla scanner in the UAB Civitan Functional Neuroimaging Laboratory, Kana’s group performed structural magnetic resonance imaging (MRI) to measure brain cortical thickness (volume data), diffusion tensor imaging to measure the connectivity of white-matter fibers of the brain and proton magnetic resonance spectroscopy to measure brain neurotransmitters like N-acetylaspartate. The brain’s white-matter areas are like electrical cables that link different regions of the brain.
The same MRI machine does all three measurements, using different settings for each. The volume data take 10 minutes, connectivity measurements take 12 minutes and the spectroscopy takes 30 minutes. While some participants were comfortable in the tight space of an MRI, others needed training in a nonfunctioning simulator MRI before any testing.
Kana’s group found significant differences in some specific measurements using each of the three neuroimaging approaches. They then combined certain of these key differences into a decision tree model — akin to the differential diagnosis flowchart used by clinicians. This decision-tree model gave a classification accuracy of 91.9 percent for distinguishing ASD subjects from the controls.
“Autism is such a heterogeneous disorder, and every patient presents with different symptoms and levels of severity,” said Lauren Libero, Ph.D., first author of the study. “That makes it really challenging to try to find one explanation within the brain for the very complex symptoms of autism.”
“This study is important,” said Libero who is now a post-doctoral scholar at the University of California-Davis MIND Institute (Medical Investigation of Neurodevelopmental Disorders), “because we found that different combinations of alterations in the brain could lead to the same or different levels of symptom severity. This could explain why previous studies have found varying results when it comes to which areas of the brain are affected in autism. There likely is not one uniform pattern affecting everyone with autism.”
“We also found that combining different MRI techniques led to better classification of our participants with autism,” Libero said. “Most previous studies have focused on using one technique at a time, even though we have evidence that there are alterations in the brain in autism in terms of structure, white-matter connectivity, and brain chemical concentrations. When we are looking at a disorder that is so complex, multiple modalities of investigation can be more efficient to separate autism from other disorders, or to identify subgroups within autism. Our study found a way to combine measures of brain structure, white matter diffusion, and neurochemical concentration to classify our participants by their diagnosis, as well as their level of autism severity.”
Specific significant findings in the Libero et al. Cortex paper include:Increased cortical thickness in ASD participants, compared to controls, across the left cingulate, left pars opercularis of the inferior frontal gyrus, left inferior temporal cortex and the right precuneus;
- Reduced cortical thickness in the right cuneus and right precentral gyrus;
- Reduced white matter connectivity (as measured by reduced fractional anisotropy and increased radial diffusivity) for two discrete clusters on the forceps minor of the corpus callosum; and
- Reduction in N-acetylaspartate in the dorsal anterior cingulate cortex.
Just three of these significant differences — radial diffusivity in the right forceps minor, cortical thickness in the left pars opercularis and fractional anisotropy in the left forceps minor —yielded the best decision tree for distinguishing ASD participants from controls.
Kana and colleagues also built a decision tree with five of the significant findings that sorted ASD participants by disease severity.
For more information: www.uab.edu