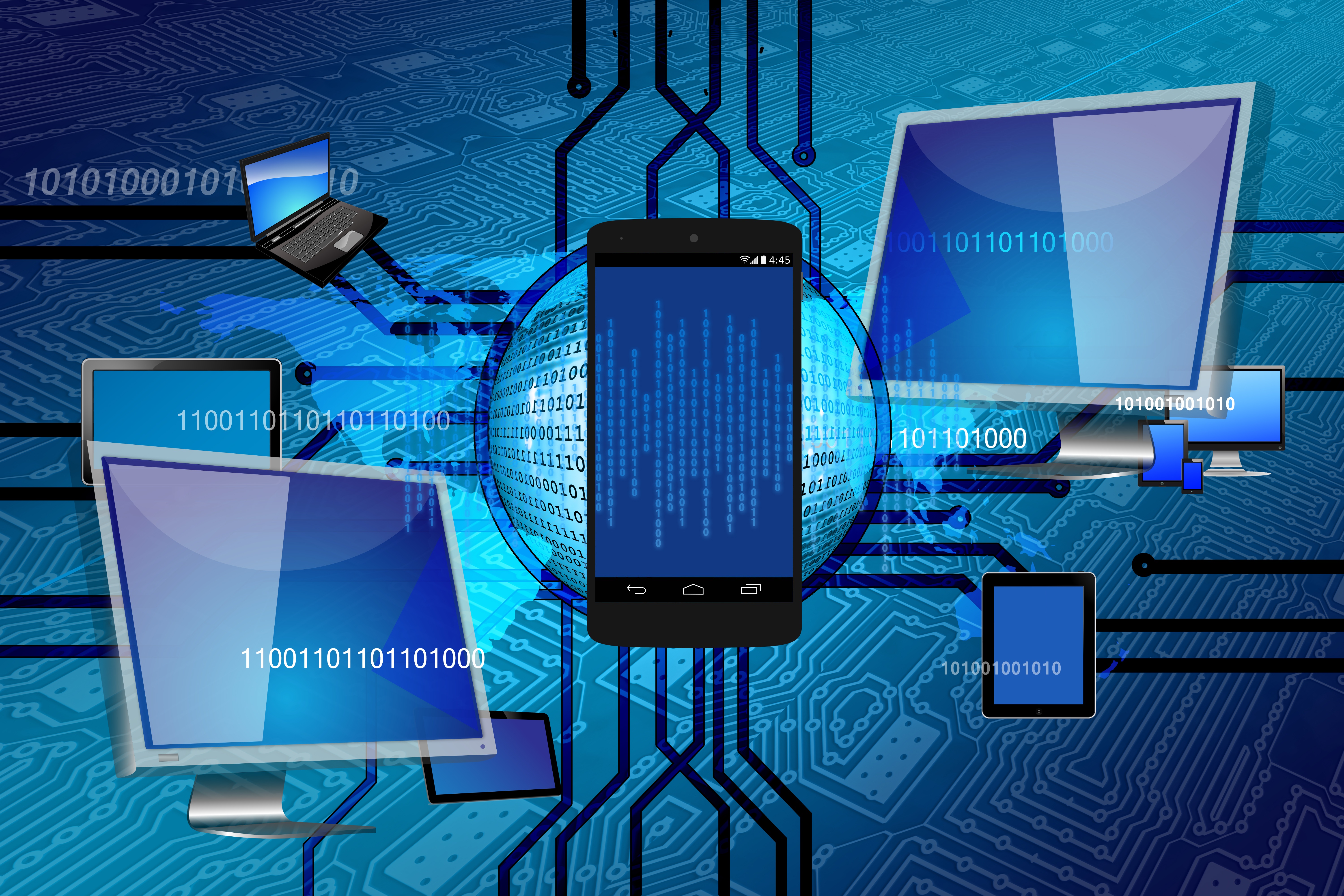
Image courtesy of Pixabay
No technology concept is going to be more discussed, dissected or debated in healthcare this year than artificial intelligence (AI). In the world of radiology and imaging, AI has the potential to dramatically impact productivity, precision and outcomes. In fact, one of the most prominent use cases for AI applications in healthcare may be in imaging. However, several factors will impact the mainstream adoption of AI in 2018.
AI has captured the imagination of the healthcare sector in the transition from a fee-for-service era to a value-based care era. The book The Big Unlock (Amazon) discusses how the digital transformation of healthcare will depend on efficiently harnessing data using emerging technologies and advanced analytical methods. One of the critical emerging technologies enabling the “big unlock” is AI.
However, the notion of AI means different things to different people. Very often, we see the terms AI, cognitive, deep learning and machine learning used interchangeably and confusingly. There are significant differences in the definitions of these terms; however at a broad level, these terms are used to indicate the application of technology and analytical methods to analyze vast amounts of data and provide insights that help clinicians improve healthcare outcomes, reduce costs of care, and enhance the healthcare experience for patients and providers alike.
The Electronic Front
The U.S. healthcare system has gone through a massive digitization of patient medical records in the past eight years, fueled by over $30 billion of taxpayer money in Meaningful Use incentives. The good news is that we now have a near-total penetration of electronic health record (EHR) systems in the country and a national electronic backbone for patient medical records. The bad news is that an estimated 80 percent of all healthcare data is unstructured data (text, images and, increasingly speech) that’s barely analyzed today. Considering every hospital generates around 50 petabytes of data annually, we are talking about enormous amounts of data, of which, reportedly, a mere 3 percent is analyzed, tagged or made actionable. Add to this the tsunami of data coming from non-medical sources (wearables, genetic testing data, internet of things data), and we are well past the point where traditional technologies and analytical methods can make sense of all the data and generate the insights in real time needed to support diagnostic and treatment decisions.
All this doesn’t even begin to address the problems of a shortage of physicians, nurses and radiologists, and the associated challenges of burnout experienced by overworked clinicians who feel burdened by inefficient processes as well as not-very-user-friendly system interfaces.
Enhancing Healthcare Delivery
Early examples are emerging of technology firms taking a serious shot at applying AI to solve some of the more complex problems of healthcare delivery. At the Radiological Society of North America’s (RSNA) 2017 annual conference, arguably the most discussed emerging technology was AI, with several major technology firms announcing new AI-enabled platforms and forging partnerships to jointly address the unlocking of actionable insights from imaging and other data sources. Examples include imaging technologies leader GE Healthcare partnering with visual computing technologies company Nvidia to boost its AI capabilities, and speech technologies leader Nuance announcing an open AI platform for diagnostic imaging to promote AI-led applications in the radiology community.
The stated goal is the same for everyone: enhancing healthcare delivery through the use of advanced analytics and AI.
Technology Industry Challenges
There are a couple of significant challenges that the technology industry has to address before AI can go mainstream.
The hype overhang. As with any new technology, AI is struggling to establish itself as a viable technology, partly due to being in its early stages, and partly due to the indiscriminate “AI-washing” by technology vendors, which makes enterprises leery of claims about the promised benefits of the technology. In other words, the hype overhangs reality by a significant margin at this point.
Enterprise readiness for new technologies. A recent survey by the University of Pittsburgh Medical Center (UPMC) and the Pittsburgh-based Center for Connected Medicine (CCM) on healthcare technology investments for 2018 indicates that AI is a very low priority, with healthcare executives looking at AI as what it is today — a promising technology in early stages. No matter what vendors may say, healthcare executives will make investments in AI only when they are ready. The teacher may be ready, but the student is not.
Other challenges for AI relate to perceptions of the impact of AI on productivity and jobs. Faced with a backlash against the “job-killing” potential of AI, many technology providers have taken to couch AI in less threatening terms such as adaptive intelligence and augmented intelligence, hoping to assuage concerns by positioning AI as something that will complement a radiologist’s work, and not replace them altogether. The truth is that AI will eliminate some types of work, but will greatly enhance other types of work. We don’t know yet where the equilibrium will be.
Making AI Mainstream
While some sectors, such as e-commerce and consumer financial services, are heavy adopters of AI, healthcare has lagged a bit; this is not surprising because healthcare has traditionally never been at the bleeding edge of information technology. Healthcare likes to see a new technology or concept play out in other sectors and try a few pilots with a limited focus to establish viability before large-scale adoption. In the meantime, we need test beds for technology innovation and poster children for its benefits. In that regard, early efforts by the leaders and pioneers among technology vendors and forward-thinking healthcare institutions are our best hope in the near term for AI to go mainstream.
Paddy Padmanabhan is the author of The Big Unlock: Harnessing Data and Growing Digital Health Businesses in a Value-Based Care Era (available on Amazon). He is the CEO of Damo Consulting Inc., a healthcare growth advisory firm based in Chicago.