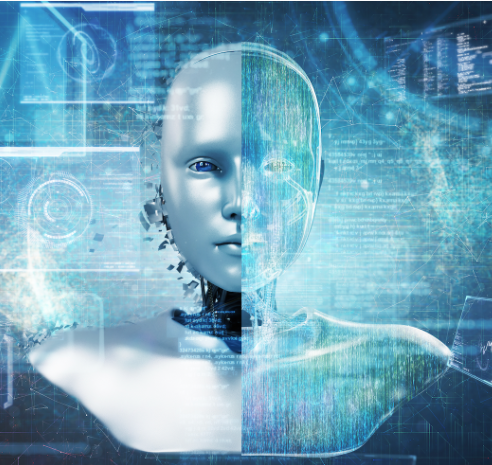
What follows is the second part of our coverage of the “Radiology: Artificial Intelligence Fireside Chat” conducted at RSNA 2021. The in-depth discussion, for which excerpts are presented here, was well-facilitated by Dania Daye, MD, PhD, Massachusetts General Hospital/Harvard Medical School; and Paul Yi, MD., University of Maryland School of Medicine; with RSNA Journal Radiology: AI Editor Charles E. Kahn, Jr., MD, MS, Perelman School of Medicine, University of Pennsylvania. Featured panelists included: John Mongan, MD, PhD, University of California, San Francisco; Jayashree Kalpathy-Cramer, MS, PhD, Athinoula A. Martinos Center for Biomedical Imaging; and Linda Moy, MD, NYU Grossman School of Medicine.
Q: The successes we have seen in AI are clear. There is cutting-edge research emerging, but with every success, we are identifying multiple obstacles. What are key obstacles preventing the translation and implementation of AI?
Dr. Kalpathy-Cramer: There are surely problems that still need to be solved, the largest of which come down to technical issues. Beware of the pitfalls. Build models that are explainable. Again, making sure we are asking the right questions and solving the right problems.
Dr. Moy: We develop models, but they are done in retrospective enriched reader studies, so you have to go back and look at what the population was, see how the model works and retest to try to get external data sets to validate and prove it. It was a struggle with us to pull it out into clinical implementation. There is much to work out, as this is not a simple process. We are very early in this part of trying to figure out how it can be trusted and how to deploy it.
Dr. Mongan: Another challenge is something we are working to overcome, which is with the data science perspective, where there is this hard problem of building a model. Most of us recognize now that the challenges of deployment are at least as large as the challenges of building the model in the first place. Some of these include many of the elements of our workflow and are difficult to differentiate as tightly as we may like to have an efficient experience with the person using it. This is something that a lot of work is going into right now.
Another challenge is what was mentioned, as data scientists, they want to solve the problem that is interesting to them. Algorithms we have available are still coming from that legacy of proof of concept — let’s do something with the data we have available, let’s do something with the problem that is obvious, though it may not necessarily be a problem that has clinical relevance. Or if it does, it may be of small enough relevance that it may be useful, but it may not be useful enough to justify the cost of going through a purchasing and integration effort — particularly because these standards are still in development, and those integrations can be quite costly. There are also some open questions around once you put these out, how are we to monitor them? If it works today, how do we ensure it works a year from now … and monitor it with post deployment surveillance? These are all challenges we are making progress on, but are some of the things slowing down our ability to do this right now.
Dr. Khan: Much of what we have built so far has been very tightly focused. Even if you have a system that looks at chest radiograms, what does it do when it sees another out-of-the-box pulmonary issue? The challenge, and this is a problem that is being approached broadly in the AI community, is this question of ignorance and boundaries of knowledge. It is important for all of us who practice...I am a body imager and if I see a skeletal lesion and do not know what it is, I go see my colleagues in a different part of radiology, and I get their expert opinion on it. Part of that is understanding I am operating at a level that is not in my comfort zone, and I am not seeing anything where the probability of diagnosis is high. AI has a problem like that as well.
So, one of the challenges for us all is to take a deep breath and acknowledge that we want to move this science forward, but we also need to recognize it can do some things in the here and now that are very effective. We can use this technology to do many of the things that can help today, and other things will follow as we gain experience and as our depth of experience of the technology grows. For those old enough to be around when MRI first came around, everyone said we will never need to biopsy ever again, this will give us all we need. Is MRI a useful tool today? Absolutely yes. But some of that was unnatural hype people put behind it and we see a lot of that in radiology today. I am enthusiastic about a lot of things, but I temper my enthusiasm about some of the things we are finding.
Q: What if AI gets it wrong? Who is liable if there is a mistake?
Dr. Mongan: On liability, that is a legal question that is not yet fully established. There will be case law over the course of the next 5-10 years. I doubt physicians and radiologists will be absolved of responsibility. In general, they are considered to have the ultimate care of the patient as the physician. And in general, they are assigned a large chunk, if not all, of the liability, so that should be our anticipation.
Leaving aside the question of liability, there is another question on responsibility. Leaving aside what law says, who SHOULD be taking responsibility? The majority rests with us as physicians as we are responsible for our patient’s care. As part of that, it behooves us to carefully consider the quality of the algorithms they are putting into practice. And to make sure how they were trained, what data they were trained on, how similar that training populations look to our patient populations, both in terms of demographics and in terms of disease prevalence. To make sure that we are comfortable with the way not just that the algorithm works, but that it is integrated in a way that we are comfortable with. Is this an algorithm that will be integrated in a way that the results will go directly to influence how patients will be cared for? Or is it going to be more advisory, and if it is going to come through a radiologist, how much ability does the rad have to verify that the results they are getting are correct? Is it going to be obvious to the rad when the algorithm is failing? So, I think it is for all these reasons we really need to take responsibility, regardless of how the liability question ends up being settled, for the performance of these algorithms moving forward.
Dr. Moy: In cases where they have reviewed CAD systems, it is the radiologist that is responsible. In those instances, there was thought that because CAD did not find it, therefore one could not diagnose it, therefore one is not responsible. There are also cases where there is a problem and they choose to ignore it…This was seen to not hold up in court. This comes to daily decisions on patient care. So, it does seem that radiologists will be the main people responsible for using the AI product.
Dr. Khan: Willie Sutton was asked why he robbed banks, and he said “That’s where the money is.” So, if the question is who will be sued, it will be where the money is: the radiologist, the health system, as well as the developer/builder/and vendor too.
Q: On the issue of privacy in AI and how to address it. There is much controversy around these questions. Who owns the data? Who should own the data?
Dr. Khan: To some extent it depends on where you are in the world. The EU has different practices. A lot of us have been sensitized in the U.S. that if you are going to use patient data, even when looking at 100,000 chest X-rays, what do we owe any given patient? So, we do not yet know the expectations. Many institutions include blanket statements in general consent forms. In the same way we allow cases to be used for teaching purposes, patients understand they are seeing students, for example, this is the same with understanding how their data will be used ethically in an attempt to further the goals of health care.
Dr. Mongan: Great questions, moral and ethical, as well as legal. There is established law in every state in the country as to who owns the data and it varies quite a bit. Historically it has not really mattered…no one was concerned because the data did not have a lot of value. Now we are entering an arena where that data has a lot of potential value, so we are likely to see changes in future about data ownership as this comes more to the fore because it matters more to people.
There are many aspects to this. It is important to us as a radiology community to try and communicate to the general public that this data is not just a way for companies to get rich, but this is a value to society. If we decide to set this up in a way that the person individually owns their data and the only way is to get individual signatures, that is an insurmountable task, and we as a society will be denying ourselves the benefits that could come from potential advantages that can come from AI. I agree with others who have noted it gets sticky when it comes to companies making money, but it is important to recognize that nothing in medicine really has a broad impact until there is a company behind it. You have to have companies standing behind these efforts who are signing contracts and providing support and this is the only way to get to where we have broad impact on the way we are delivering radiology and these new advances in health care.
Q: What are the biggest pitfalls for data privacy and how can they be addressed?
Dr. Khan: There are a number of concerns about that, and we are looking at how to de-data our files, although it is very time consuming. The amount of Patient Health Information (PHI) present in radiology is relatively small. There are techniques with text data that allows you to change a name, for those who have released data sets. It costs a lot of money to be reviewed by humans. It becomes a very detailed effort to try to ensure the information is stripped out.
Dr. Mongan: A pitfall or barrier to being able to do work with these data sets is that we are in an environment where the standard for de-identification is 100%. Because there are all these places that PHI can hide…we need to start thinking whether 100% is the right threshold for what de-identification should be? Because if that is where we draw the line, it makes assembling these data sets oftentimes prohibitively expensive. And we are going to, to a large extent, bar ourselves from making these advances. With different countries who may draw the line at different thresholds there is a tradeoff.
Countries that say absolute privacy is key, they will not advance as fast with these technologies. So, we need to think about privacy not as always needing to be 100%, but as something that has a tradeoff and has both benefits and costs.
Dr. Moy: We have developed a breast US data set of 5.4 million images. Many groups have come to us asking if our team can share some of that data set. We wanted to, but the cost of us going through each so the PHI is removed is prohibitively expensive and, as a result, we are not able to offer that.
Kalpathy-Cramer: It is important to consider all of this, and we need to have a rational conversation about what privacy is.
Dr. Khan: What are we to do when something happens and there are big consequences? Could you have an insurance program that basically says we are going to de-identify these images and 95% are clean, but the issue is for one or two that sneak through and cause a penalty? Can that be covered with an insurance policy? If we’re looking at a million images and ten leak through, is a small policy cheaper than spending a dollar on every single case where the file needs cleaning?
Another thing is that we do not ever share images of data outside of our own institutions, but we can pass the model around. Also, we should recognize that Google or Apple seem to know precisely where I am and what I search for…we have been scanned and analyzed online. So, it is the case that there is a lot of information that we very readily give up to various companies. These are all good questions and things we need to keep working on. As much as we talk about the tragedy of the commons, there is also the loss of the opportunity of the commons. As a society, we may lose our ability to organize to recognize opportunities, to serve the greater good.
Q: What are you most excited about now and looking down the pipeline at the next frontier in AI?
Dr. Kahn: I think one of the things that is exciting about AI is its ability to guide us to normal. We’re taking steps that allow us to create a range and establish what is normal for a 65-year-old versus 30-year-old, and maybe it has to do with patient height, etc. All kinds of information that helps us make a decision at what percentile it is and see what is normal or abnormal. This is the kind of thing where we can be helped by using this technology, and I think you get toward decision medicine, which is exciting.
Dr. Moy: Focusing on trying to equalize health care disparities in the world. So, yes, you can have a great AI model, but it is training people how to position the probe, etc. Then we can say we are able to provide a relatively standard level of healthcare irrespective of country or wealth, etc.
Dr. Mongan: What I am looking forward to in the coming years is moving from the point where panels at conferences like this stop talking about the potential, and start realizing that potential. This means actually seeing more impacts and measuring them, discussing them, recognizing the challenges, and getting these things worked out. These are hard problems we are working on but we are making a lot of progress.
We are poised right on the brink of this translational revolution of bringing these things out of the lab and out of the data science bench and into the clinic. We are seeing this with the AI Imaging in Practice demo, and in coverage of radiology AI in practice. So I’m looking most forward to it ceasing to be a purely theoretical thing, and being something that is ubiquitous in our everyday practice, improving the lives of radiologists and our patients.