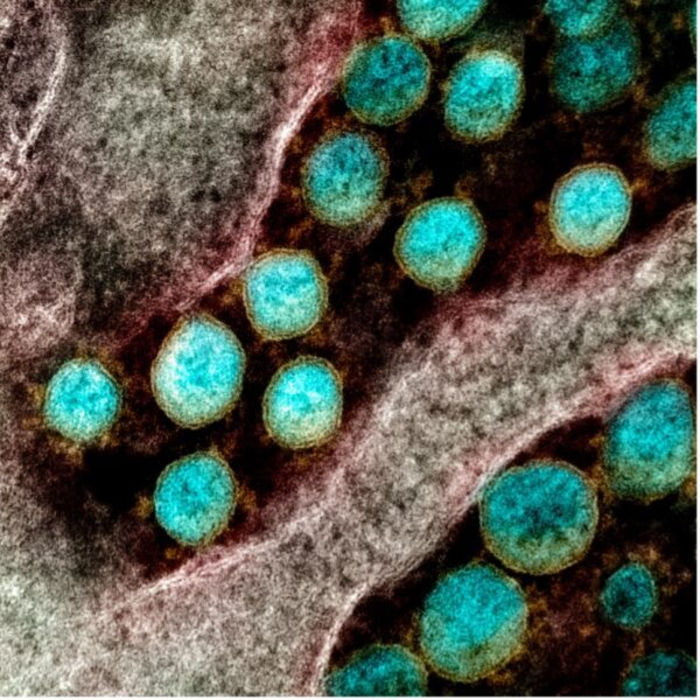
Transmission electron micrograph of SARS-CoV-2 virus particles, isolated from a patient. Image captured and color-enhanced at the NIAID Integrated Research Facility (IRF) in Fort Detrick, Maryland. Image courtesy of NIAID
Clinical scientists used machine learning (ML) models to explore de-identified electronic health record (EHR) data in the National COVID Cohort Collaborative (N3C), a National Institutes of Health-funded national clinical database, to help discern characteristics of people with long-COVID and factors that may help identify such patients using data from medical records.
The findings, published in The Lancet Digital Health, have the potential to improve clinical research on long COVID and inform a more standardized care regimen for the condition.
“Characterizing, diagnosing, treating and caring for long-COVID patients has proven to be a challenge due to the list of characteristic symptoms continuously evolving over time,” said first author Emily R. Pfaff, PhD, assistant professor in the Division of Endocrinology and Metabolism at the UNC School of Medicine. “We needed to gain a better understanding of the complexities of long-COVID, and for that it made sense to take advantage of modern data analysis tools and a unique big data resource like N3C, where many features of long COVID are represented.”
Sponsored by the National Institutes of Health’s National Center for Advancing Translational Sciences (NCATS), the N3C data enclave currently includes information representing more than 13 million people from 72 sites nationwide, including nearly 5 million COVID-19-positive cases. The resource enables rapid research on emerging questions about COVID-19 vaccines, therapies, risk factors and health outcomes.
This new research is part of the National Institutes of Health’s Researching COVID to Enhance Recovery (RECOVER) initiative, which has been recruiting thousands of participants nationwide in order to answer critical research questions about the syndrome to accurately identify who has long-COVID, risk factors for long-COVID, and potential interventions and treatments.
Using the N3C, researchers developed XGBoost machine learning (ML) models to understand patient characteristics and better identify potential long-COVID patients.
Researchers examined demographics, healthcare utilization, diagnoses, and medications for 97,995 adult COVID-19 patients. They used these features on nearly 600 long-COVID patients from three long-COVID specialty clinics to train and test three ML models, which focused on identifying potential long COVID patients in three groups:: among all COVID-19 patients, among patients hospitalized with COVID-19, and among patients who had COVID-19 but were not hospitalized.
The models proved to be accurate in identifying potential long-COVID patients, achieving areas under the receiver operator characteristic curve, a measure of accuracy used by machine learning researchers, of 0.91 (all patients); 0.90 (hospitalized); and 0.85 (non-hospitalized). Patients flagged by the models can be interpreted as “patients warranting care at a long-COVID specialty clinic.” Applying the model to the larger N3C cohort can also achieve the urgent goal of identifying long-COVID patients for clinical trials.
The models also showed many important features that differentiate potential long-COVID patients from non-long-COVID patients. They focused on patients with a positive COVID diagnosis who were at least 90 days out from their acute infection. Features more commonly identified among potential long COVID patients include post-COVID respiratory symptoms and associated treatments, non-respiratory symptoms widely reported as part of long COVID (such as sleep disorders, anxiety, malaise, chest pain, and constipation), pre-existing risk factors for greater acute COVID severity (such as chronic pulmonary disease, diabetes, and chronic kidney disease), and proxies for hospitalization, suggesting greater severity of acute covid. The study also points out that it is plausible that long-COVID will not ultimately have a single definition, and may be better described as a set of related conditions with their own symptoms, trajectories, and treatments.
“These results speak to the powerful impact of real-world clinical data and the potential capabilities of N3C to help better understand and find solutions for significant public health problems such as long COVID,” said NCATS Acting Director Joni Rutter, PhD.
Josh Fessel, MD, PhD, senior clinical advisor at NCATS and a scientific program lead in RECOVER, added, “Once you’re able to determine who has long COVID in a large database of people, you can begin to ask questions about those people. Was there something different about those people before they developed long COVID? Did they have certain risk factors? Was there something about how they were treated during acute COVID that might have increased or decreased their risk for long COVID?”
The study included how electronic health record (EHR) data is skewed toward patients who make more use of healthcare systems. Pfaff says that it is essential to acknowledge whose data is less likely to be represented – uninsured patients, patients with limited access to or ability to pay for care, or patients seeking care at small practices or community hospitals with limited data exchange capabilities.
“Electronic Health Records (EHRs) only have information for people who go to the doctor,” said Pfaff, who is also Co-Director of the NC TraCS Informatics and Data Science (IDSci) Program. “They also have more information on people who go to the doctor a lot. So, people who don’t have good access to care or people who don’t go to the doctor, we’re just not going to have information about them. So this is a caveat that I offer with every EHR based study that I do. We need to recognize who’s not in the dataset.”
The N3C team continues to refine its models as more real-world data emerges. Their longitudinal data for COVID-19 patients can provide a comprehensive foundation for the development of ML models to identify potential long-COVID patients. As larger cohorts of long-COVID patients are established, future work will include research to identify subtypes of long-COVID, making the condition easier to study and treat.
“Depending on where the research leads, we may find that patients with different presentations of long COVID are different enough to warrant different treatments entirely,” said Pfaff. “So, it’s important for us to determine if long COVID is one disease, or a constellation of related conditions that are also related to having had acute COVID-19.”
With the help of this big data approach, efficient study recruitment efforts can become available to deepen the understanding and complexities of long-COVID. Beyond identifying cohorts for research studies, understanding and validating the relationship between long-COVID and social determinants of health and demographics, comorbidities, and treatment implications will only improve the algorithm in these models as more evidence emerges.
“Research studies, particularly clinical trials, are one of our best tools for gaining understanding of long COVID — its presentation, risk factors, and potential treatments,” said Pfaff. “For the best chance at success, studies need large and diverse groups of participants who qualify, which aren’t easy to find. Using algorithms like the one we’ve created on large clinical datasets can narrow down vast numbers of patients to those who could qualify for a long COVID trial, potentially giving researchers a head start on recruitment, making trials more efficient, and hopefully getting to findings faster.”
This study was funded by NCATS and NIH through the RECOVER Initiative.
For more information: https://ncats.nih.gov
Related Long-COVID Content:
MRI Sheds Light on COVID Vaccine-Associated Heart Muscle Injury
What We Know About Cardiac Long-COVID Two Years Into the Pandemic
VIDEO: Long-term Cardiac Impacts of COVID-19 Two Years Into The Pandemic — Interview with Aaron Baggish, M.D.
VIDEO: Long-COVID Presentations in Cardiology at Beaumont Hospital — Interview with Justin Trivax, M.D.
VIDEO: Cardiac Presentations in COVID Long-haulers at Cedars-Sinai Hospital — Interview with Siddharth Singh, M.D.
Find more COVID news and videos
Related COVID Content:
COVID-19 Fallout May Lead to More Cancer Deaths
Kawasaki-like Inflammatory Disease Affects Children With COVID-19
FDA Adds Myocarditis Warning to COVID mRNA Vaccine Clinician Fact Sheets
CMS Now Requires COVID-19 Vaccinations for Healthcare Workers by January 4
Cardiac MRI of Myocarditis After COVID-19 Vaccination in Adolescents
Small Number of Patients Have Myocarditis-like Illness After COVID-19 Vaccination
Overview of Myocarditis Cases Caused by the COVID-19 Vaccine
Case Study Describes One of the First U.S. Cases of MIS-C
NIH-funded Project Wants to Identify Children at Risk for MIS-C From COVID-19