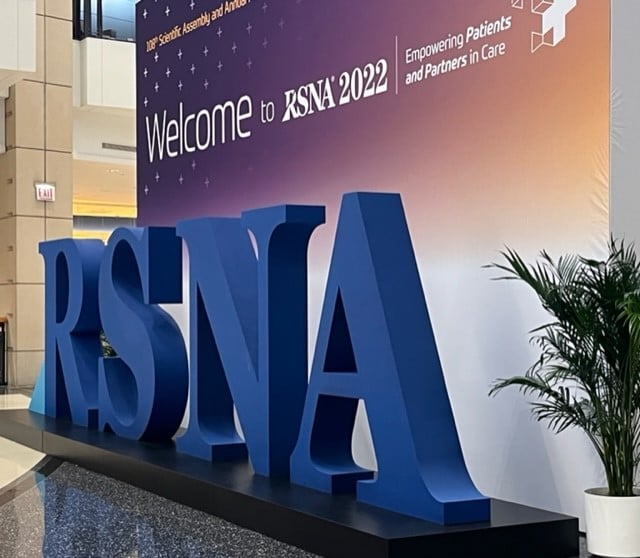
As the Radiological Society of North America Scientific Assembly and Annual Meeting neared its close, attendees at three in-depth sessions on Nov. 30 learned from leading authorities on artificial intelligence (AI), machine learning and the promise, pitfalls and possibilities moving forward.
November 30, 2022 — On the fourth day of RSNA 2022, inside Chicago’s McCormick Place, an estimated 260 separate events took place over 12 hours, while nearly 650 vendor exhibits shed light on the latest products and technology. The Imaging Technology News (ITN) editorial team is once again offering a summary of key sessions which serve as a representative sampling of quality programming at the core of the 108th Radiological Society of North America Scientific Assembly and Annual Meeting.
Attendees at three in-depth sessions heard from leading authorities on the basics of artificial intelligence (AI), got a tutorial aimed at understanding the workings of an Automated Breast Ultrasound system, and gained wide-ranging insight into the development of machine learning in clinical trials and practice for radiation oncology. Here, then, key highlights, with more in-depth coverage in the weeks and issues ahead.
Back to the Basics: Radiology AI in 2022
While this in-depth session will be covered more broadly by ITN in a separate article, the presence of this session — and the esteemed panel of AI experts — on the RSNA 2022 agenda is newsworthy in and of itself. Its place on the schedule is a logical follow up to the popular AI Fireside Chat presented during RSNA 2021, and a reflection of the fast pace of growth in technology seen on the exhibit floor and in practices today.
The panel included four highly-regarded AI specialists:
RSNA 2022 Gold Medal Recipient Katherine P. Andriole, PhD, is an Associate Professor of Radiology at Harvard Medical School, Brigham and Women's Hospital and Director of academic research and education at the Mass General Brigham Data Science Office. A long-standing member of the RSNA Radiology Informatics Committee, Dr. Andriole currently serves on the RSNA Machine Learning Steering and Data Standards sub-committees. She is a faculty member for the RSNA Imaging AI Certificate Program, a subject matter expert for the R&E Foundation's Grant Oversight Committee and serves as co-director of the Imaging AI in Practice Demonstration.
Linda Moy, MD, is Professor of Radiology at the NYU Grossman School of Medicine with additional appointments at the NYU Center for Advanced Imaging Innovation and Research and the NYU Vilcek Institute of Graduate Biomedical Sciences. She is director of breast MRI (clinical and research) throughout the NYU Health Network, and co-leads an international AI team that spans five NYU Langone Health institutions.
Dania Daye MD, PhD, interventional radiologist at Massachusetts General Hospital and faculty member at Harvard Medical School and at the MGH/HST Martinos Center for Biomedical Imaging. Among other leadership roles, she is Co-chair of the Women in Radiology Steering Committee at MGH and current co-chair of the MGH Department of Radiology Diversity, Equity and Inclusion Committee.
Walter F. Wiggins, MD, PhD, is a board certified neuroradiologist, Assistant Professor of Radiology, Neuroradiology at Duke Health. A strategic advisor to Qure.ai, Wiggins is focuses on the use of advanced imaging and image analysis technologies in diagnostic imaging of the brain, head, neck, and spine, with a particular focus on the clinical implementation of artificial intelligence (AI) technologies for medical imaging.
Daye also referenced an RSNA journal Radiology report recently published, “Implementation of Clinical Artificial Intelligence in Radiology: How Decides and How?” As lead author, with Wiggins and others, Daye identified essentials for clinical AI, including: data access; data science; cross modality; and delivery. She and the panel further addressed the need for AI governance, and shared key takeaways on successful implementation of AI.
ITN spoke with Daye prior to the program, and asked about the goals of the session, to which she shared these insights:
“I think for the last couple of years we have had such an explosion of AI applications we are seeing in the market, and if you look around, there are so many people using AI in their clinical practice. Yet, we still see so many radiologists out there who are not really familiar with the basics of AI. We’ve seen a huge interest in becoming more familiar and really learning about the basics of implementation. So the purpose of this session is trying to get the very basics to the people who are just starting to jump into this area and learn a little bit more about data science, implementation and governance, and really take a look at what is out there and how it can be used to provide an infrastructure to get people started. This is surely the beginning of a very long journey, but we hope to get people to dip their toes in the water, so to speak, and help get them started.”
Classroom-Style Teaching on Cutting Edge AI
An interactive hands-on reading workshop was led by Georgia Giakoumis Spear, MD. As it has been widely reported that real-world results show that adding FDA-approved AI tools to automated breast ultrasound helps physicians increase their reading speed with a great degree of confidence, Spear addressed the benefits of ABUS in supporting radiologists’ workloads, while remaining laser-focused in detection and patient care.
“The whole point of ABUS is to complement mammography, and to detect those interval cancers that mammography cannot find, and which have higher mortality rates,” said Spear to those participating in the training, adding, “So if we find them with ABUS we’re saving lives.”
In this GE Healthcare vendor workshop, a follow-up to her session during RSNA 2021 which was featured in ITN, eight different case studies were presented, with Spear, as instructor, guiding the participants through the ABUS system, incorporating QView CAD technology. Qview Medical, a breast cancer detection pioneer and maker of the FDA-approved artificial intelligence (AI) software system for breast cancer screening, and GE Healthcare announced in Nov., 2021, that QView's QVCAD software can be launched on GE Healthcare's Invenia ABUS 2.0 (Automated Breast Ultrasound), which has an open platform technology that allows the integration of third-party AI tools for the optimization of the reading workflow. Invenia ABUS 2.0 is the first FDA-approved Ultrasound supplemental breast screening technology specifically designed for detecting cancer in dense breast tissue. The powerful AI Assistant leverages intelligent algorithms to assist in detecting breast lesions, helping physicians increase their reading speed with confidence.
A passionate advocate and widely-recognized leader driving ABUS for breast cancer screening and diagnosis, Spear eagerly shared insights, experiences and procedural tips to her “students.” She reinforced the importance of having automated breast ultrasound exams done on the same day as mammograms, helping to reduce recall rates, while improving cancer detection rates.
Plenary on Machine Learning in RO Clinical Trials and Clinical Practice
The key question asked and answered by the high-level panelists in the Plenary session focused on Machine Learning in Radiation Oncology was “How can we use AI in clinical trials?”
Introduced by RSNA Past-President Bruce Haffty, MD, the session presenters offered updates on initiatives presently in play to advance trials supporting radiation oncology, the breadth of work that has been undertaken, and what’s ahead. Four panelists, led by Quynh-Thu Le, MD, Professor, Chair, Department of Radiation Oncology at Stanford, noted the fundamental ways AI is applied to radiation oncology, including: treatment decision; imaging (stimulation); treatment planning; plan approval and QA; radiotherapy delivery; and follow-up care. She presented an overview of the hierarchical relationships between, and definitions of, deep learning, machine learning and artificial intelligence.
Addressing Potential and Pitfalls of ML
“There are data that suggest that AI can optimize eligibility criteria, match patients to trials, and address health equity in trial entry and enrollment,” said, Le. She addressed the role of machine learning in optimizing the workflow of radiation therapy that can enhance education, time saving and consistency.
The presenters shared updates and insights on a range of meaningful developments: automatic segmentation, AI in imaging for personalized therapy, the Trial Pathfinder, the development of the Minimum Common Oncology Data Elements (mCODE), the community which has formed it, CodeX, and the NRG/RTOG, a clinical trial cooperative group for cancer patients.
Other panelists included Felix Feng MD, University of California, San Francisco, whose focus is on pathology, who spoke to the value of clinical trials for evidence information. Feng shared the mapping going on presently within the EHR, and the importance of involvement and support of federal agencies, radiologists, physicists, and addressed some of the reasons why AI tools are promising as biomarkers, specifically noting performance, turnaround time — immediate in a digital world, and access — as it is potentially easier to implement internationally.
Michael Gensheimer, MD, Clinical Associate Professor, Radiation Oncology-Radiation Therapy, Stanford, contributed with an in-depth, technical assessment of how machine learning of digital data including imaging, digital pathology, electronic medical records is being used to risk-stratify patients for clinical trials as well as to predict for treatment benefit.
Ruijiang Li, PhD, DABR, Associate Professor, Department of Radiation Oncology (Medical Physics) Stanford, addressed advances in machine learning and imaging analytics for personalized cancer care. He noted a phrase which has been regularly adopted within Stanford University’s Human-Centered Artificial Intelligence, HAI, which underscores the collective sentiment heard and seen throughout RSNA 2022: “Human in Charge, Machine in the Loop.”
All focused on the shared goals of cancer detection and diagnosis, prediction of response and treatment, and prognosis, and acknowledged the collective consensus that there needs to be a better job done by all involved in collecting data.
For more information: https://www.rsna.org/annual-meeting