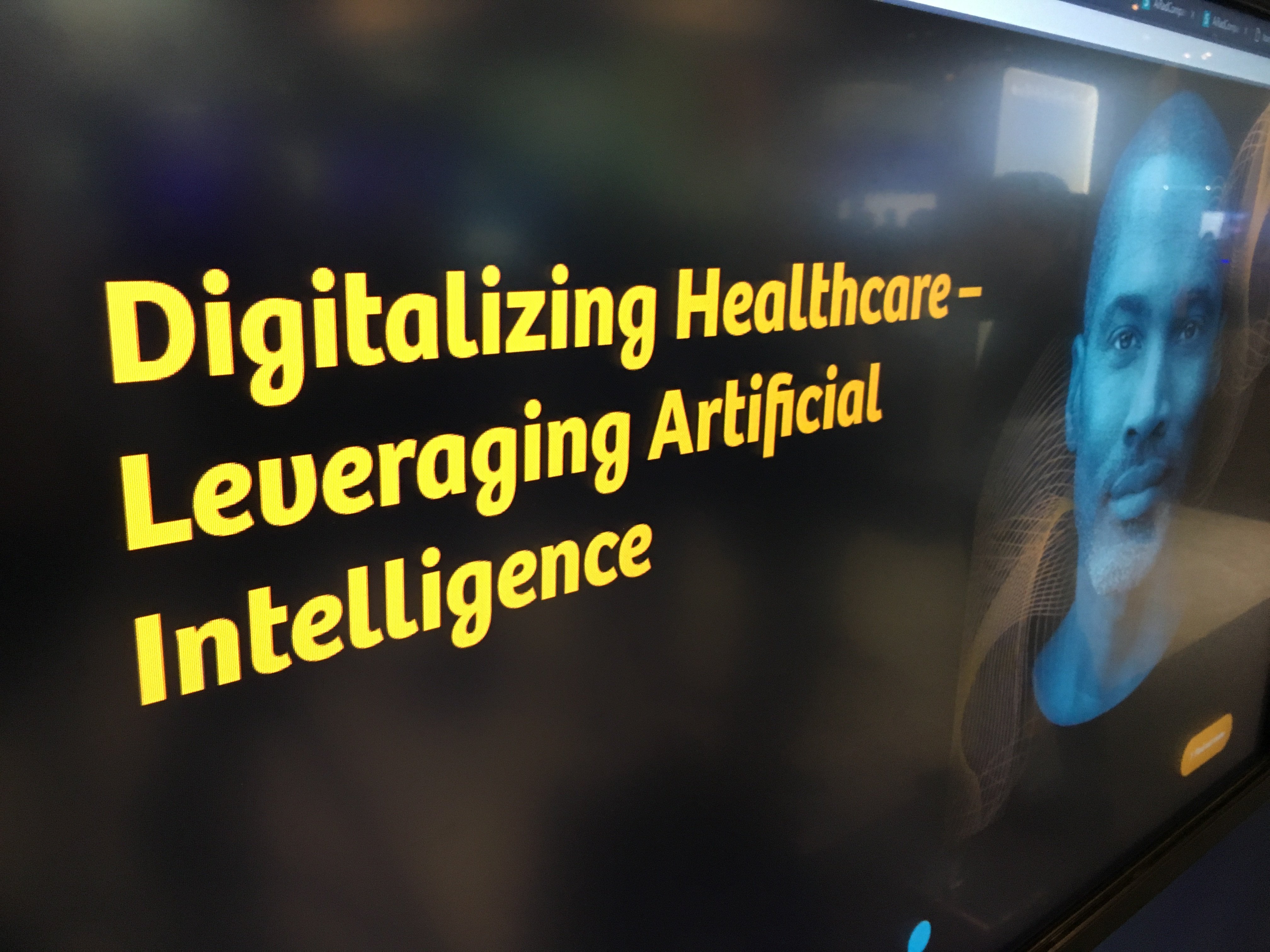
For the last several years, artificial intelligence (AI) has represented the newest, most rapidly expanding frontier of radiology technology. Expo floors at all the major professional society meetings are full of vendors showcasing AI tools they have developed or integrated into their products, billed as efficiency and time-savings aids to help ease the workload of radiologists who are increasingly bogged down by vast amounts of data.
Despite the promises and potential, however, widespread clinical implementation of AI in radiology has yet to occur. Early adopters are providing potential pathways for adoption, and vendors and clinicians continue to work together to ensure AI is actually doing what radiologists need it to do.
Obstacles to Implementation
According to numerous key opinion leaders in the fields of radiology and AI, there are a few main obstacles AI currently faces to widespread adoption.
A profusion of algorithms that are designed for specific applications. Many of the AI algorithms with clinical applications are designed to help radiologists do one very specific thing — estimate bone age on a hand X-ray, predict which patients are likely to develop Alzheimer’s disease and assess the certainty that a lesion detected on a mammogram is cancerous, among countless others. While these are all useful applications and may save radiologists time and effort on that particular task, it can be difficult to justify the expense of purchasing a tool that only has one specific use.
“If you have to look at a separate algorithm for the best bone age, the best pneumothorax, it’s going to be too much,” said Samir S. Shah, M.D., MSCE, vice president of clinical operations for Radiology Partners. “When we do use a plugin, if it has to go back to the server and takes 12 minutes to come back, that’s too much time.”
Lack of access to sufficient data to train algorithms. The niche focus of many current AI algorithms, according to Khan Siddiqui, M.D., founder and CEO of AI company HOPPR, is due to a lack of the massive data sets that would be required to train larger algorithms. Early AI developers have focused on data that is freely available in large quantities to help solve one problem at a time. “But that’s not how we practice,” Siddiqui said. “Unless you’re a highly specialized radiologist in a high-tier academic institution where you only see one kind of scan, that’s not how most radiologists work.”
Many current AI algorithms do not fit easily into the existing workflow of radiology. Ultimately, with so many algorithms available and not enough data to train algorithms that might have broader use, today’s radiologists are often left with AI algorithms that do not easily fit into their existing workflow. This often forces providers to take a piecemeal approach to building a library of applications. Even if they are only interested in one algorithm, there is no guarantee that it will integrate seamlessly into their workflow.
Getting AI and PACS to Work Together
In most radiology departments and practices, workflow runs through the picture archiving and communication system (PACS), as this is where all of the imaging data and associated reports are kept. All image viewing, reporting and sharing is done through the PACS, and each vendor’s PACS has different functionality. Many of today’s artificial intelligence algorithms are being developed independent of a particular PACS, which has made it difficult to provide AI solutions that will work for everyone.
“If we look at the history of introducing advanced tools into radiology, we didn’t see very good adoption. It wasn’t until they got incorporated directly into the viewers, etc., that they got used,” said R. Kent Hutson, M.D., CPE, neuroradiologist with Radiology Partners and the director of imaging informatics for Matrix Radiology. Hutson was one of the panelists in a recent webinar on breaking through the bottlenecks to successful translation of AI into clinical radiology. “You can’t graft on extra plugins and expect radiologists to use those [plugins].”
“You’ve got to modify your product to all the existing PACS systems, because we’re now dealing with major health systems that are using PACS that are inconfigurable with anything else,” added Shah, highlighting the need for AI developers to adapt their strategy.
Such partnerships will likely require modifications from the PACS vendors as well, with the goal of creating a simple, unified interface between all products. “With the move toward VNA [vendor neutral archives], I think it opens up the opportunity for better user experience with the image viewers. The current software just is not up to task to integrate well with the tools we’re proposing,” said Hutson.
Integrating AI With Modalities
Another possible avenue for those interested in radiological applications of AI to pursue is integrating algorithms directly into the imaging systems themselves. This will require developers and clinicians to figure out how to help the algorithms communicate with the DICOM (Digital Imaging and Communications in Medicine) standard-based technical systems and data at the heart of radiology today.
The international body behind DICOM created the working group WG-23: Artificial Intelligence/Application Hosting, whose mission is to “identify or develop the DICOM mechanisms to support AI workflows, concentrating on the clinical context. This includes the interfaces between application software and the back end DICOM infrastructure.”
The organization behind the IHE (Integrating the Healthcare Enterprise) standard also put out a pair of proposals in 2019 on how to integrate AI into the radiologist workflow. “They’re baby steps, but they’re very important, foundational steps so we can get agreement amongst all these various companies that are trying to do this, and interoperability between their systems,” said Hutson.
Examples of how new algorithms could operate directly inside an imaging system include:
• Reorienting X-ray images for the PACS so that radiologists can view them in a simple, reproducible fashion;
• Enhancing reproduction and sharing of ultrasound exam data. Shah, a teleradiologist by trade, said he receives scans of hand-written notes from an ultrasound technologist, which Shah then reads into his dictaphone. “I’m dictating the same way I did 20 years ago,” he said. “The opportunities for error are just compounded when you’re doing that kind of workflow;” and
• Identifying echocardiograms that need to be redone before being sent out to the referring cardiologist.
“So I see AI in the entire continuum — not just workflow but going all the way back to acquiring the images from the very beginning,” said Anthony Chang, M.D., MPH, MS, MBA, a pediatric cardiologist and the chairman and founder of AIMed.
Building AI Onto Existing Networks
While AI is not yet a clinically useful tool in every radiology practice, larger facilities and provider institutions are demonstrating ways to make the technology dovetail with and enhance their existing programs and processes.
Radiology Partners, a large physician-led and physician-owned radiology practice in the U.S., wanted a technological solution to decrease the amount of variability in imaging reporting among its physicians, particularly around incidental findings. “We looked out at the industry and we couldn’t find anyone that was doing that — something that could scale our best practices to help our radiologists,” said Nina Kottler, M.D., vice president of clinical operations for Radiology Partners. “So we had to go externally to create something.”
The resulting solution, dubbed RecoMD, sits on top of the practice’s existing voice recognition system, which already employs natural language processing (NLP), a form of AI, for dictation. RecoMD sifts through the information as the radiologist is dictating and identifies any information that may indicate an incidental finding that could require follow-up. It takes the dictated information, combines it with metadata from the radiology report and creates
a recommendation.
“It’s not so helpful if you go back after I dictate a report and say, ‘Hey, go back and change this’ or ‘You made the wrong recommendation,’” Kottler said. “What you really want is someone who can tell you that as you’re doing the report.”