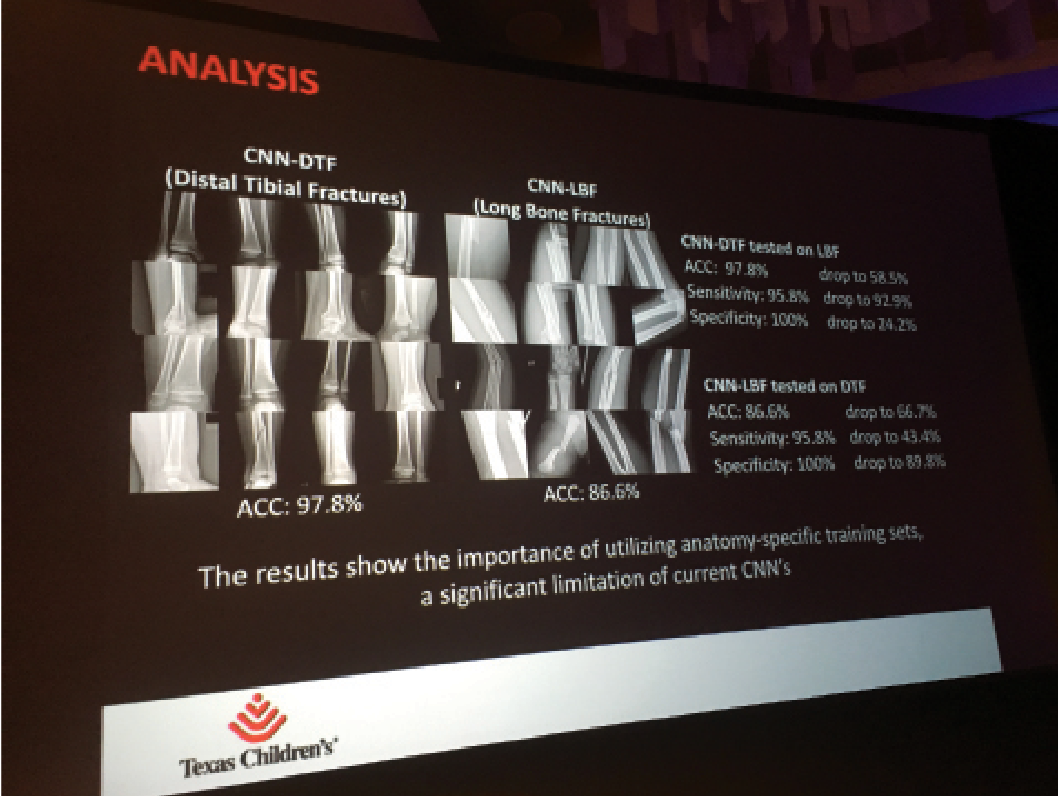
Sudhen Desai, M.D.
Burnout has become a popular buzzword in today’s business world, meant to describe prolonged periods of stress in the workplace leading to feelings of depression and dissatisfaction with one’s occupation. The topic has become so pervasive that the World Health Organization (WHO) addressed it at its 2019 World Health Assembly in Geneva in May, adding burnout to the 11th revision of the International Classification of Diseases (ICD-11) — although classifying it as an “occupational phenomenon” rather than a medical condition.
Healthcare itself is not immune to burnout, and a recent study in Journal of the American College of Radiology demonstrates it is taking a toll on pediatric radiologists in particular. The study surveyed Society of Pediatric Radiology (SPR) members and found nearly two-thirds expressed at least one symptom of burnout. While burnout is a complicated phenomenon and no two people experience it the same way, a commentary on the study suggests artificial intelligence (AI) could help alleviate some of the difficulties that can lead to burnout.
Defining Burnout
Rama S. Ayyala, M.D., is a pediatric radiologist with the Department of Diagnostic Imaging at Rhode Island Hospital-Hasbro Children’s Hospital and Warren Alpert Medical School of Brown University. She wanted to study burnout because she had been interacting with a lot of people in her field who were experiencing it. “A lot of them were leaving medicine and some were even having mental health issues,” she said.
This sentiment was also born out in the most recent edition of the National Physician Burnout, Depression and Suicide Report, published by Medscape. The report ranks medical subspecialties by the percentage of clinicians reporting feelings of burnout. Radiology ranked seventh in the 2018 Medscape survey — alarming enough by itself, but even more so because it jumped from 18th place in the previous edition.1
Ayyala’s research team surveyed SPR members on their feelings of burnout according to the three components of the Maslach Burnout Inventory (MBI), originally published in 1981:
• Emotional exhaustion (feeling overextended and depleted);
• Depersonalization (a sense of cynicism, and/or a detached or callous attitude toward your job); and
• Lack of personal accomplishment (perceived incompetence with capabilities in the job).
A total of 460 clinicians returned responses out of the 1,453 who were sent the survey, a 32 percent response rate. The prevalence rate for emotional exhaustion among the respondent group was 66 percent and depersonalization was 61 percent.
“People feel like they’ve lost their sense of control,” said Ayyala.
The survey included additional questions to gather more information about the roles and job characteristics of pediatric radiologists, including:
• Gender;
• Full- or part-time employment status;
• How long they have been practicing;
• Practice type;
• Total number of pediatric radiologists in the practice; and
• On-call status and frequency of being on-call.
Out of all the job characteristics surveyed, on-call status seemed to have the single greatest association with increased feelings of burnout. “Many children’s hospitals currently struggle with how to provide 24-hour radiology coverage,” Ayyala stated.
AI Making Way Into Pediatric Imaging
Technology has dramatically changed the way radiologists practice, as remote viewing systems, the evolution of picture archiving and communication systems (PACS) and mobile devices allow them to read images from essentially anywhere. For some radiologists who feel burned out due to their workload, however, technology may be able to help alleviate some of their burden — specifically artificial intelligence (AI).
Michael J. Callahan, M.D., who is the director, computed tomography; division chief, abdominal imaging; medical director, ultrasound; and staff radiologist in the Department of Radiology at Boston Children’s Hospital, wrote a commentary for Pediatric Radiology on Ayyala’s study. The topic of burnout has become a point of focus at recent SPR meetings, and the society is working to find possible solutions to help its members. According to Callahan, AI has been discussed as one approach to help improve efficiency in image interpretation. “Although theoretical solutions exist for a multitude of problems, steps to moderate and manage the effects and consequences of burnout in pediatric radiologists will undoubtedly require in-depth discussions at future SPR meetings,” he wrote.
The use of AI has exploded in radiology as a whole in recent years, with vendors and clinicians exploring its use for everything from image interpretation to worklist sorting and more. Despite the immense interest, AI is taking slightly longer making its way to clinical use in the subspecialty of pediatric radiology. This is largely due to the need for large volumes of data to train algorithms, a feat which is difficult with the generally lower volumes of pediatric imaging, according to Sudhen Desai, M.D., an interventional radiologist at Texas Children’s Hospital.
“A system like Texas Children’s, which has a good amount of inpatient and outpatient volume, has available to itself the ability to create datasets to train models from. That’s one of our luxuries,” he said. “However, there are smaller pediatric institutions that suffer from lack of available data.”
Part of the problem, according to Desai, is that the need for larger volumes of data runs counter to the long-standing protocol of limiting the exposure of children to radiation, advanced through national initiatives such as Image Wisely. While this has placed limitations on the adoption of AI in pediatric radiology, the technology itself may also offer a way forward. Desai said that work is ongoing to train the algorithms with lower numbers of radiographs or cross-sectional imaging. With this information, algorithm architects can employ methods such as feedback learning (teaching the computer to take actions in an environment so as to maximize the concept of a cumulative reward) and transfer learning (using the knowledge gained from one application and applying it to a different but related problem). “So we’re trying to use various tricks and techniques to train our models in a more efficient fashion so that we don’t need tens of thousands of datasets but ... maybe hundreds of datasets, that would allow us to do the work,” Desai said.
Using AI to Combat Burnout
As suggested by Callahan, the role of AI in combating pediatric radiologist burnout may be in streamlining and/or automating some of the processes and tasks that entail a heavy workload burden. Several practitioners are already exploring the use of AI in a clinical pediatric imaging setting, offering many promising applications.
Bone age detection. Bone age evaluation is one of the most common practices in pediatric radiology. Radiologists examine series of hand radiographs to evaluate a patient’s growth and diagnose developmental disorders. The Radiological Society of North America (RSNA) made this the focus of its first annual Machine Learning Challenge in 2017. Teams of researchers were encouraged to develop their own algorithms for automating bone age detection by using a dataset of hand radiographs compiled from various leading U.S. research institutions. Participants in the challenge were judged on how well the bone age evaluations produced by their algorithms agreed with the expert observers’ notated evaluations. The winning algorithm is now available through the EnvoyAI Exchange platform.
Safwan Halabi, M.D., a clinical associate professor of radiology – pediatric radiology at Stanford Children’s Health, has been using the bone age algorithm in his clinical research. He is currently collaborating with researchers at nine other institutions to validate the algorithm “and it’s looking really good,” he said. “We could eliminate some of our mundane things that we measure [because they] can be automated and sent directly to the electronic health record.”
Pneumonia detection. Halabi’s Stanford colleague Matthew Lungren, M.D., an assistant professor of radiology (pediatric radiology) is part of a group developing an AI algorithm to improve pneumonia detection on chest X-rays. Their model is trained on a National Institutes of Health (NIH) dataset consisting of 112,120 frontal view chest X-rays from 30,805 unique patients. In a preliminary paper published in 2017, the algorithm was found to exceed the performance of an average radiologist in detecting pneumonia.2
Pneumonia detection was the focus of the 2018 RSNA Machine Learning Challenge.
Interpreting tubes and catheters in pediatric X-rays. Another challenge in the world of pediatric imaging is the fact that many of the smallest, sickest patients have a large number of tubes and other apparatus attached to them — feeding tubes, catheters, ECG leads and more. When these patients need to be imaged, the radiologist will typically have to go through each image and identify artifacts that are external to the patient.
Desai stated that research is currently being done to train AI to identify and classify these outside objects in an X-ray. Using adult chest radiographs, the research group added markers for lines and tubes, and that information was fed back into the AI model. The model was then applied to pediatric radiographs, and it was able to identify lines, tubes and other objects “on the order of about 80 percent,” Desai said. The ultimate goal is for the computer to recognize catheters or tubes that are out of position and alert the radiologist.3
Human Element Still Required
These and other applications offer hope for artificial intelligence to act as a workflow organizer for pediatric radiologists experiencing burnout from an intense workload. But while the technology continues to mature, it is critical for the people guiding the technology to carefully consider its application.
“Burnout is a very broad term which can stem from many different causes,” said Stacey Funt, M.D. Funt previously worked as a full-time body radiologist in New York before her own experiences with burnout caused her to reorder her priorities. She now works in radiology part-time while speaking across the U.S. as a health and wellness coach for healthcare providers through her own company, Lifestyle Health LLC. Funt’s experience with burnout stemmed from a perceived loss of connection with other doctors and her patients. Ultimately, she believes that the most helpful technological solutions are those that can help improve communication and connection across the healthcare continuum. This could be especially helpful for the subset of radiologists who identified being on-call as a potential source of burnout. “I think one source of stress for radiologists that technology can address, especially when on-call, is when you need to contact referring physicians to relay critical information but you can't reach anyone. Another is if you have a really tough case that you are not sure of but don't have anyone present to run it by. So it all depends on the issues for each person, and that’s variable,” she concluded.
Related AI Coverage:
VIDEO: Editor’s Choice of the Most Innovative New Artificial Intelligence Technologies at RSNA 2018
VIDEO: Technology Report: Artificial Intelligence
How to Market Healthcare Artificial Intelligence Software
References:
1. Medscape National Physician Burnout and Depression Report 2018. https://www.medscape.com/slideshow/2018-lifestyle-burnout-depression-6009235#3 Accessed Aug. 19, 2019
2. Rajpurkar P., Irvin J., Zhu K., et al. CheXNet: Radiologist-Level Pneumonia Detection on Chest X-Rays with Deep Learning.arXiv.org, Nov. 14, 2017. https://arxiv.org/abs/1711.05225 Accessed Aug. 19, 2019
3. Yi X., Adams S., Babyn P., Elnajmi A. Automatic Catheter and Tube Detection in Pediatric X-ray Images Using a Scale-Recurrent Network and Synthetic Data. Journal of Digital Imaging, published online April 10, 2019. https://doi.org/10.1007/s10278-019-00201-7