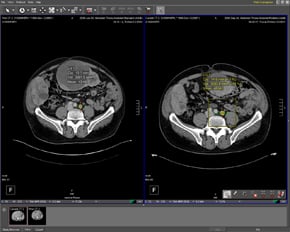
Lymph nodes are a primary site of metastatic spread for almost all malignant tumors. Tracking changes to lymph node burden and lymph node staging has become increasingly important in diagnostics, as evidenced by the recent revision of RECIST criteria in early 2009. Clinicians recognize that lymph node staging is central to cancer patients’ treatment course and care management.
Lymph nodes are present throughout the body, often in close proximity to, or in direct contact with, organs, vasculature and intestinal structures. As a result, it is often difficult for human readers and computer software to delineate lymph nodes or accurately detect and measure lesions.
Understanding the metabolic processes and changes to the biophysical properties of lymph nodes can provide valuable insight into patients’ health and their tumor progression. The extent of metastatic cell infiltration affects predictions of patients’ survival outcomes and therapeutic options.
Current methods for assessing lymph nodes rely on anatomic imaging and manual measurements, whether on a computer screen or on a physical medium. This approach is very reader-dependent and has inherent inaccuracies, which tend to yield results that are more qualitative than quantitative and offer little information about tumor response therapies and volumetrics. The shortcomings of such methods are largely attributable to the limitations of available imaging devices and elucidate the need for more integrated and quantitative techniques – techniques suited to accurately stage and monitor lymph nodes in cancer management in a reader-independent way.
The standard practice in assessing changes in lymph nodes is to manually measure the largest diameter of a lymph node on an axial slice and compare it to a previous scan. This approach has a limited ability to accurately measure the sensitivity and specificity of volumetric changes, especially for examining small lesions where a change of 2-3 mm (which is within typical inter-reader variation) can represent a doubling in volume. Additionally, accurate identification of metastatic tissue is sometimes challenging as metastases can be present in non-enlarged lymph nodes, and further not all enlarged lymph nodes are malignant. It is apparent that reader-independent measurement tools that quantify results can provide greater insight into volumetric changes through improved accuracy and sensitivity, while allowing for shorter follow-up intervals for patients undergoing systemic therapy and more reliable early detection of non-responders.
This review establishes the need for more accurate and sensitive lymph node imaging techniques and discusses how computer aided diagnosis (CAD) tools can address this need. Definiens LymphExpert, an image analysis application recently introduced by Definiens, streamlines the analytic process by simultaneously combining images while providing both volumetric and quantitative information.
Primary Imaging Tools
Most primary tumors are staged using the American Joint Committee on Cancer classification to assess the local extent and size of the primary tumor (T), regional lymph node involvement (N), or distant metastasis (M).
Previously, bipedal lymphography was the standard test for assessing and staging lymph nodes. This technique, which evaluated and categorized lymph nodes based on changes in internal architecture, was invasive and failed to consistently display lymph nodes above the level of the second lumbar vertebrae and outside the retroperitoneum. Bipedal lymphography was replaced by the more versatile cross-sectional imaging techniques (MRI and CT) that are able to display images and assess tumors with much greater reliability and accuracy.
Medical sonography (ultrasonography) is an ultrasound-based diagnostic imaging technique, which uses real-time tomographic images to visualize the size and structure of anatomical features and pathological lesions. Ultrasound is widely available, easy to use and ideal for imaging and assessing cervical lymph nodes in patients with head and neck cancer. However, it is not ideal for imaging lymph node groups located in the mediastinum, retroperitoneum and deep pelvis.
Cross-sectional imaging with either CT and MRI employs a combined imaging modality dependent on lymph node anatomy rather than function and physiology. While lymph node positioning within the body cannot be easily deciphered in ultrasound, images derived from cross-sectional techniques provide a three-dimensional vantage point. This noninvasive technique is quick, well tolerated and is the imaging technique of choice for assessing primary tumors. Cross sectional imaging evaluates lymph node size, density, borders and signal intensity. The contrast provided by cross sectional imaging can compensate for the sensitivity and specificity deficiencies of ultrasound in assessing deeply embedded lymph nodes and distinguishing nodes from surrounding tissue.
However, because MRI and CT employ node size as the primary staging criterion, and size alone is not enough to differentiate between benign and malignant nodes, it can be difficult to establish a specific threshold value determined by size and signal. A low size threshold value provides higher sensitivity with low specificity while a higher size threshold value lowers the sensitivity but improves specificity. A traditional size approach frequently overlooks metastasis, particularly when the metastasis involves only microscopic or partial infiltration of the lymph node. Further, benign inflammatory or infectious lymph node enlargement can lead to incorrect characterization, making MRI, on the basis of size criterion alone, no different or slightly worse than CT in the assessment of regional lymph node metastasis.
Functional imaging, 18F-FDG Positron Emission Tomography (18F-FDG PET), uses small amounts of radioactive material to visualize and measure body functions such as intracellular glucose metabolism, blood flow and oxygen utilization, which differentiate between healthy and diseased tissues. For cancer, PET is the most powerful imaging tool for disease management as it is able to find and identify more areas of active cancer. PET imaging most often uses the glucose analog 18F-FDG as a radioactive tracer to greatly improve staging of malignancies, such as lung cancer. , F-FDG PET is distinct from sonography and cross sectional imaging in its ability to assess tumor response and infiltration. The disadvantage of this technique is that it provides limited quantifiable morphological information due to its physically limited spatial resolution. This makes it difficult to pinpoint the anatomical localization of increased glucose uptake and tumors that have glucose metabolism sub-detection levels. In addition, distinguishing between an inflammatory disease and a malignancy has proven to be a challenge because inflammatory states also exhibit increased glucose metabolism. This shortcoming has been addressed by using different tracers and combining morphological and functional imaging into a dual modality known as 18F-FDG PET/CT.
With technical improvements in imaging modalities, the advent of computer-aided diagnostic tools (CAD) is helping to improve the accuracy and sensitivity of diagnostic image analysis.
CAD is an interdisciplinary technology that combines elements of artificial intelligence with digital and radiological image processing. Because CAD systems are used primarily for evaluating noteworthy structures and sections of tissue its utility is based on highly complex pattern recognition. After several thousand radiological images have been copied to a CAD server, they are scanned for suspicious structures. This provides an accurate secondary reading for clinicians, and gives them the opportunity to benefit from accurate quantification tools.
However, most CAD software applications fail to provide clinicians with supplemental, highly accurate volumetric measurements that can provide additional insight beyond manual diameter measurements. Clinical studies conducted by the Radiology Department of the University of Munich indicate that Definiens LymphExpert recently introduced by Definiens is capable of processing images from different modalities simultaneously, providing accurate measurements of volumetric changes in lymph nodes. The application automatically segments, extracts and quantifies lymph nodes that are so small that the average human reader cannot reliably detect changes in size, while also providing the volumetric measurements that other methods of imaging lack. This allows for increased detection performance regarding the number of detected lymph nodes as well as size changes.
CAD Holds Promise
Recent advancements have seen systemic therapies, such as chemotherapy, receptor therapy and antibodies, among others, become more effective. However, the successes of these therapies are specific to tissue type, receptor expression and other properties of tumors – properties that often change through the course of the disease. Therapy monitoring has therefore become crucial, especially in lymph nodes, where minute changes can be indicative of stage changes in the cancer.
The most common approach to detecting patients’ non-responsiveness to therapies is to monitor changes in the size of lesions over time. The more accurate the measurement of size and volume of lesions, and the more comprehensively such data can be quantified, the earlier non-responders can be detected and a change to regimen of therapy and/or therapy scheme can be made. In this light, computer-aided tools like CAD offer significant promise to support clinicians in the rapid, accurate assessment of cancer staging.
References:
1. From RECIST to PERCIST: Evolving Considerations for PET Response Criteria in Solid Tumors. J Nucl Med 2009 50: 122S-150S (DOI: 10.2967/jnumed.108.057307).
2. American Joint Committee on Cancer, American College of Surgeons. AJCC Cancer Staging Manual. 5th ed. Fleming ID, Cooper JS, Henson DE, et al., eds. Philadelphia, PA: American Cancer Society; 1997.
3. Guermazi A, Brice P, Hennequin C, Sarfati E. Lymphography: an old technique retains its usefulness. Radiographics. 2003;23:1541-1558.
4. Baatenburg de Jong RJ, Rongen RJ, De Jong PC, et al. Screening for lymph nodes in the neck with ultrasound. Clin Otolaryngol. 1988;13:5–9.
5. Kau RJ, Alexiou C, Laubenbacher C, Werner M, Schwaiger M, Arnold W. Lymph node detection of head and neck squamous cell carcinomas by positron emission tomography with fluorodeoxyglucose F 18 in a routine clinical setting. Arch Otolaryngol Head Neck Surg. 1999;125:1322–1328.
6. Dwamena BA, Sonnad SS, Angobaldo JO, Wahl RL. Metastases from non-small cell lung cancer: mediastinal staging in the 1990s: meta-analytic comparison of PET and CT. Radiology. 1999;213:530–536.
7. Pieterman RM, van Putten JW, Meuzelaar JJ, et al. Preoperative staging of non-small-cell lung cancer with positron-emission tomography. N Engl J Med. 2000;343:254–261.
8. Bipat S, Glas AS, van der Velden J, Zwinderman AH, Bossuyt PM, Stoker J. Computed tomography and magnetic resonance imaging in staging of uterine cervical carcinoma: a systematic review. Gynecol Oncol. 2003;91:59–66.
9. Anzai Y, Piccoli CW, Outwater EK, et al. Evaluation of neck and body metastases to nodes with ferumoxtran 10-enhanced MR imaging: phase III safety and efficacy study. Radiology. 2003;228:777–788.
10. Antoch G, Stattaus J, Nemat AT, et al. Non-small cell lung cancer: dual-modality PET/CT in preoperative staging. Radiology. 2003;229:526–533.
11. Sohn KM, Lee JM, Lee SY, et al. Comparing MR imaging and CT in the staging of gastric carcinoma. AJR. 2000;174:1551–1557.
12. Curtin HD, Ishwaran H, Mancuso AA, et al. Comparison of CT and MR imaging in staging of neck metastases. Radiology. 1998;207:123–130.