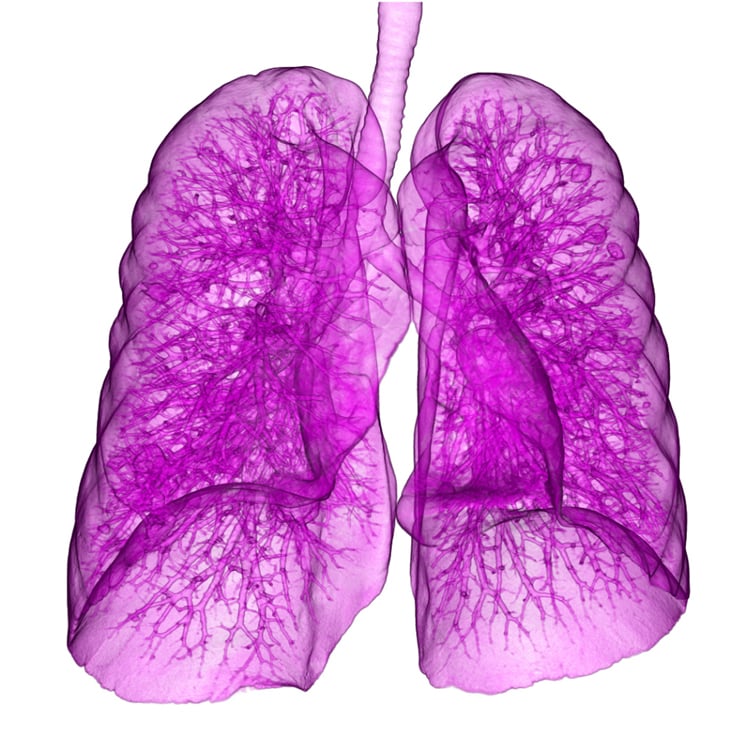
This image, taken with Canon’s Aquilion One, shows a lung with metastases from bowel cancer. Image courtesy of Canon.
In recent years, low-dose computed tomography (CT) screening has emerged as a proven, effective method to detect lung cancer earlier, which can reduce mortality up to 20 percent.1 While the technology has proven effective, numerous research efforts have explored use of another up-and-coming technology — artificial intelligence (AI). This technology may further improve nodule detection, classification and sizing, while also reducing false-positive rates. A session at the 2017 Radiological Society of North America (RSNA) annual meeting explored the potential of AI to aid radiologists in assessing lung cancer diagnoses in CT scans.
Machine Learning Vs. Deep Learning
The key to utilizing AI in radiology lies in understanding the difference between machine learning and deep learning — terms often used interchangeably with artificial intelligence that are very different styles of employing computers to make predictions. AI was one of the hottest topics at RSNA, featured all over the show floor and in sessions. A 2017 survey of peer-reviewed literature underscored this trend. The research team found over 300 articles on the use of deep learning in medical image analysis, most which had been published within the previous year.2
Machine learning involves teaching a computer how to arrive at a particular conclusion, accomplished by feeding it massive amounts of data. At RSNA 2017, one case study highlighted a group of Canadian researchers who developed a machine learning model that could determine whether a single lung nodule was malignant. The model accounted for numerous factors traditionally used to assess lung cancer risk. These include age, sex, family history, presence of emphysema, and various aspects of the nodule itself (size, type, location, number nodules in the scan, etc.). The model was used to assess a development dataset and a validation dataset. The model results for both sets showed excellent discrimination and calibration.3 “You do not directly use the image. You go into this model where you can translate it into a set of numbers, and these numbers go into a formula,” explained Bram van Ginneken, Ph.D., professor of medical image analysis at Radboud University Medical Center and co-chair of the Diagnostic Image Analysis Group, Nijmegen, The Netherlands, who presented several studies at RSNA.
In contrast to the equation-based style of machine learning, deep learning teaches the computer to actually recognize the features of an image rather than translating them into numbers. This is achieved by layering a series of algorithms on top of each other to create what is called an artificial neural network (ANN), modeled after the way the human brain is constructed.4 Such a method was used in a 2015 study to describe nodules based on their solid-core and nonsolid-core characteristics. A separate algorithm was used for each type of nodule, and the results were fused to arrive at three different segmentations — the overall segmentation, the solid core only and the nonsolid part.
“The crucial difference with deep learning is you basically bypass the step with features, and you try to learn features directly from the images. So not only the mapping of the features to prediction, but the features are learned from the image itself,” said van Ginneken.
AI and Lung-RADS
In 2014, the American College of Radiology (ACR) introduced the Lung CT Screening-Reporting and Data System (Lung-RADS) to standardize the reporting and management of screening-detected lung nodules. The guidelines were also implemented to help reduce the number of false-positives from lung cancer screening, which they were demonstrated to do in a 2015 study. The false-positive rate in the study was 13 percent, compared to the 27 percent rate reached in the National Lung Screening Trial (NLST).5
Today, van Ginneken said there are more than 1,500 U.S. facilities certified in Lung-RADS-based screening. The system categorizes lung nodules found in CT scans into one of four classes between 0 (incomplete) and 4 (suspicious) based on their type and size. Each category also lists recommended management procedures, including follow-up low-dose CT screening and/or positron emission tomography(PET)/CT for suspicious findings.
Van Ginneken demonstrated how artificial intelligence can be employed to help radiologists classify nodules under Lung-RADS. “Basically you have to do three things — you have to find all the nodules, you have to determine their type and you have to measure them accurately — then you can simplify the rules and know what to do next on the screening protocols,” he told his RSNA audience.
One study van Ginneken participated in used a deep learning system to classify lung nodules in a CT scan. The system analyzed the areas of the scan that might harbor 00a nodule and extracted 2-D views at nine different orientations, then the research team trained the neural network to fuse these views together to create a 3-D image.6 “We were very impressed by the results,” van Ginneken said. “It worked much better than our classical machine learning-based model detector.”
Category 4X. A recent update to Lung-RADS introduced Category 4X, which allows radiologists to upgrade category 3 or 4 nodules with additional features or imaging findings that increase the suspicion of malignancy. The purpose of this change was to more carefully distinguish between benign and malignant subsolid nodules (SSNs). The efficacy of this expanded classification was demonstrated in a 2017 study where 15-24 percent of a group of 374 Category 3, 4A and 4B SSNs were upgraded to Category 4X by a group of experienced chest radiologists, with a malignancy rate of 46-57 percent per observer.7
Van Ginneken was part of a follow-up study that used the same dataset from the National Lung Cancer Trial to compare the malignancy risk estimation between a computer model and human observers. The researchers asked 11 radiologists to assess a set of 300 chest CT scans, featuring 62 proven malignancies and two subsets of randomly selected baseline scans. The results indicated the performance of the model and the human observers was equivalent for risk assessment of malignant and benign nodules of all sizes.8
The study did find, however, that the radiologists were superior to the computer model for differentiating malignant nodules from size-matched benign modules.8
Sharing Data to Improve AI
While these and other studies have demonstrated the great potential of artificial intelligence to supplement lung cancer detection, the scientific community is looking outward to define the next evolution of the technology. The nature of AI has encouraged the owners of large datasets to share their information with the public in an effort to spark further innovation and develop more advanced models.
Van Ginneken and his colleagues previously organized such an effort, launching the Lung Nodule Analysis (LUNA) challenge in the spring of 2016. Supplying lung CT scans from the Lung Image Database Consortium and Image Database Resource Initiative (LIDC-IDRI), the organizers invited the larger research community to develop new AI algorithms in either a nodule detection or a false positive reduction track. Van Ginneken noted that more than 3,000 groups have already downloaded the data and worked
with it. “This also shows that in the data science and deep learning community, there’s a lot of interest to work on these medical problems,” he said. The organizers stopped processing new LUNA16 submissions in January.
The 2017 edition of the Kaggle Data Science Bowl — an annual competition organized by Booz Allen Hamilton and data analytics company Kaggle — also focused on applying AI algorithms to lung cancer detection. Contest organizers sought AI algorithms designed to 1) identify when lesions are cancerous, and 2) reduce false positive rates. A total of $1 million in prize money was offered to the top 10 teams, and the algorithms were to be made open-source to allow access to all interested parties. The top three teams out of nearly 10,000 participants were announced last May.
References
- Aberle D.R., Adams A.M., Berg C.D., et al. “Reduced Lung-Cancer Mortality With Low-Dose Computed Tomographic Screening.” New England Journal of Medicine, Aug. 4, 2011. DOI: 10.1056/NEJMoa11028732.
- Litjens G., Kooi T., Bejnordi B.E., Setio A.A.A., et al. “A Survey on Deep Learning in Medical Image Analysis.” Medical Image Analysis, July 26,2017. DOI: https://doi.org/10.1016/j.media.2017.07.005
- McWilliams A., Tammemagi M.C., Mayo J.R., Roberts H., et al. “Probability of Cancer in Pulmonary Nodules Detected on First Screening CT.” New England Journal of Medicine. Sept. 5, 2013. DOI: 10.1056/NEJMoa1214726
- Grossfeld B. “A Simple Way to Understand Machine Learning vs Deep Learning.” Zendesk.com, July 18, 2017. https://www.zendesk.com/blog/machine-learning-and-deep-learning/
- Pinsky P.F., Gierada D.S., Black W., Munden R., et al. “Performance of Lung-RADS in the National Lung Screening Trial: A Retrospective Assessment.” Annals of Internal Medicine. April 7, 2015. DOI: 10.7326/M14-2086
- Ciompi F., Chung K., van Riel S.J., Setio A.A.A., et al. “Towards automatic pulmonary nodule management in lung cancer screening with deep learning.” Nature Scientific Reports. April 19, 2017. doi:10.1038/srep46479
- Chung K., Jacobs C., Scholten E.T., Goo J.M., et al. “Lung-RADS Category 4X: Does It Improve Prediction of Malignancy in Subsolid Nodules?” Radiology. July 2017. https://doi.org/10.1148/radiol.2017161624
- Van Riel S.J., Ciompi F., Winkler Wille M.M., Dirksen A., et al. “Malignancy risk estimation of pulmonary nodules in screening CTs: Comparison between a computer model and human observers.” PLOS One, Nov. 9, 2017. https://doi.org/10.1371/journal.pone.0185032