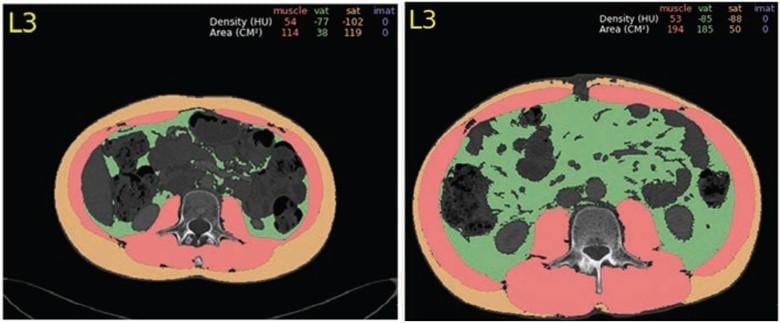
Axial CT images at L3 level in 21-year-old woman (left) and 47-year-old man (right) show automated segmentation of skeletal muscle (coral color), used to determine skeletal muscle area (SMA). SMA measured 114 cm2 in (left) and 194 cm2 in (right). Green indicates automated segmentation of visceral adipose tissue; orange indicates automated segmentation of subcutaneous adipose tissue; blue indicates automated segmentation of intermuscular adipose tissue.
October 30, 2023 — According to the American Journal of Roentgenology (AJR), the conventional skeletal muscle area (SMA) height scaling power of 2 is not supported by allometric modeling and may contribute to sarcopenia overdiagnosis in tall patients.
“Conventional CT analysis that scales cross-sectional area of skeletal muscle by subject height squared perform suboptimally,” wrote first author Louis Blankemeier, MS, from the department of electrical engineering at Stanford University. “Our work evaluates scaling powers stratified by age and sex, and with mortality outcomes, to determine optimal scaling powers for muscle.”
This AJR accepted manuscript by Blankemeier et al. included 16,575 patients (mean age, 56 years; 6,985 men, 9,590 women) who underwent abdominal CT from December 2012 through October 2018. Using automated software to determine SMA at L3, the sample was stratified into 5,459 patients without major medical conditions—via ICD-9 and ICD-10 codes—for determining an optimal height scaling power, and 11,116 patients with major medical conditions for testing this power. The optimal scaling power was determined by allometric analysis (whereby regression coefficients were fitted to log-linear sex-specific models relating height to SMA) and by analysis of statistical independence of SMI from height across scaling powers.
Ultimately, in the test set of 11,116 patients, Cox proportional hazard models incorporating SMI and age predicted all-cause mortality with higher concordance index using the derived optimal height scaling power of 1, rather than the conventional scaling power of 2 in men (0.675 vs. 0.663, p < .001) and women (0.664 vs. 0.653, p < .001).
For more information: www.arrs.org