
Greg Freiherr has reported on developments in radiology since 1983. He runs the consulting service, The Freiherr Group.
Why Artificial Intelligence Should Not Slip Into the Background
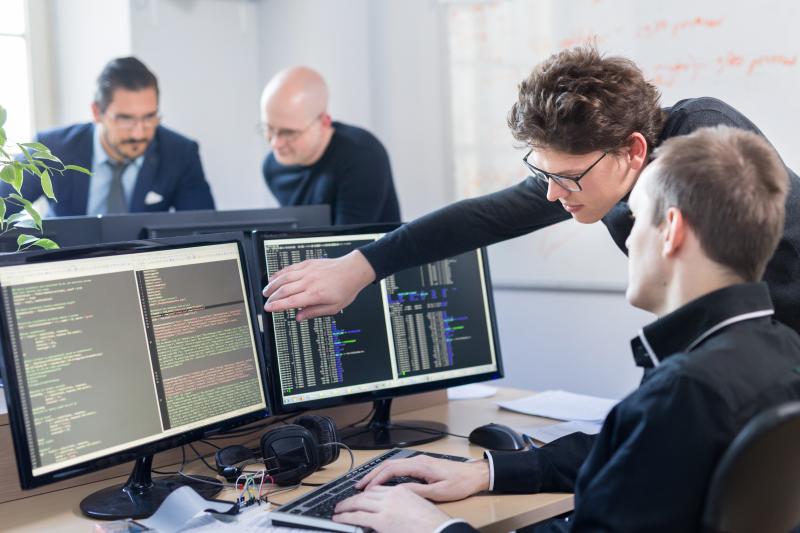
Now that artificial intelligence (AI) has clawed its way into the mainstream, some vendors want us to forget it is there. What really matters, they say, is the end result. Knowing that AI is the reason for the conclusions is a needless distraction, they say.
But being distracted may be better in the long run than not being able to judge the validity of the underlying process. If radiologists want to secure a future as key opinion leaders (KOL), they need to take control of AI now. Here’s why.
To Obscure — Or Not to Obscure
The argument in favor of cloaking AI goes like this: vendors will be tempted to use AI as a marketing tool, as in: “Our competitors don’t have AI — but we do.” This, to some degree, is already happening.
Last year, as I walked the RSNA exhibit floor, vendors repeatedly name-dropped AI into conversations. This happened regardless of the product. When we spoke about PACS and its steroidal doppelganger, enterprise imaging, the mention of AI was all but impossible to avoid.
But a few vendors advocated a different tack. Their executives told me they would prefer AI to drop from sight. What matters, they said, is the bottom line — the effect, either clinical or operational, that AI has on the product. If the machine is more efficient and it makes medical practice less costly, it shouldn’t matter whether smart algorithms were involved. All that should matter is the end result.
The argument seemed to make sense. And, after being barraged by AI claims, I embraced it like a climber at the peak of Mount Everest offered an oxygen mask. Then it hit me.
The argument ASSUMES that the efficiencies coming from AI are without compromise. But what makes radiologists faster and their work less costly? With AI out of sight, it would be difficult to determine whether corners were cut, and certainly if the data underlying the technology was valid.
The Distraction of AI
There is no question that AI can be used as a marketing tool. But that is the price of progress. It has been for decades. Look no further than the computed tomography (CT) slice wars of a few years ago.
Focusing on a specification is easier than understanding the often-complicated technology. But to be accepted, clinical answers derived from the use of machines require validation. And this is where radiologists can come in.
A critical step for radiologists to solidify their positions as future KOLs is to understand AI. Keeping AI from disappearing into the background is a big part of this argument.
Obscuring the thought process underlying a technology is akin to embedding it — and the AI — into “black boxes.” Writing in the March 2018 New England Journal of Medicine, David Magnus, Ph.D., director of the Stanford University Center for Biomedical Ethics, and his Stanford colleagues¹ stated that constructing machine learning systems as black boxes “could lead to ethically problematic outcomes.”
A year later, in a March 2019 ITN podcast (http://bit.ly/3d8D4JH), Anthony Chang, M.D., went a step further. The pediatric cardiologist, acknowledged internationally as an expert in AI, said opacity threatens the credibility, even the adoption of AI. “We have to do our best to make it a glass box — not a black box,” Chang said.
Why Understanding is Essential
Knowing the basics of how AI works — and how it affects the output of smart machines — is critically important, according to Bradley J. Erickson, M.D., a professor of radiology and director of the radiology informatics laboratory at the Mayo Clinic in Rochester, Minn. Speaking in an ITN podcast November 2018 (http://bit.ly/3a0raiZ), he said radiologists need a basic understanding of AI so they will know how it might fool us or give a spurious result.
Erickson’s cautionary statement may apply to AI being trained to interpret medical images, as well as applications such as the selection of tools for image interpretation; ones for fetching and orienting images from prior exams; and ones designed to accelerate the reporting process. The impact on the daily practice of medicine of machines with such operational capabilities could be enormous.
Computer-aided detection software and speech recognition systems have been using AI for years. These are in routine use today. And systems using AI could become even more prevalent in 2020, particularly in radiology, according to some vendors.
Given AI’s current and likely increasing footprint, it is essential that someone be able to verify that its use is better. For Charles E. Kahn, Jr., M.D., a professor and vice chair of radiology at the University of Pennsylvania Perelman School of Medicine in Philadelphia, that “someone” is the radiologist. In an ITN podcast in June 2019 (http://bit.ly/2IYbGQI), Kahn said “it is incumbent on all of us as radiologists, when we implement these systems, that we test them rigorously to make sure they work.”
Radiologists’ Opportunity
Having the knowledge to look competently under the hood of prospective equipment could establish radiologists as being indispensable. If a vendor says an imaging engine has a metaphorical 8 cylinders, radiologists might be called on to count them and to render an opinion about whether those cylinders can power the vehicle as claimed.
Once purchased, the continuing correct operation of AI-enhanced equipment would be essential. Radiologists could play a key role in this.
In short, when it comes to patient health, it makes sense to apply a Russian proverb that Ronald Reagan was fond of quoting: “Trust … but verify.” Radiologists, as the users of AI-enhanced imaging machines, have the inside track to provide that verification.
Greg Freiherr is consulting editor for ITN, and has reported on developments in radiology since 1983. He runs the consulting service, The Freiherr Group.
Reference:
1. Char DS, Shah NH, Magnus D. Implementing Machine Learning in Health Care — Addressing Ethical Challenges NEJM. 2018 Mar 15; 378(11): 981-983 — doi:10.1056/NEJMp1714229