
Greg Freiherr has reported on developments in radiology since 1983. He runs the consulting service, The Freiherr Group.
The New AI: Why The FDA Is Not Enough
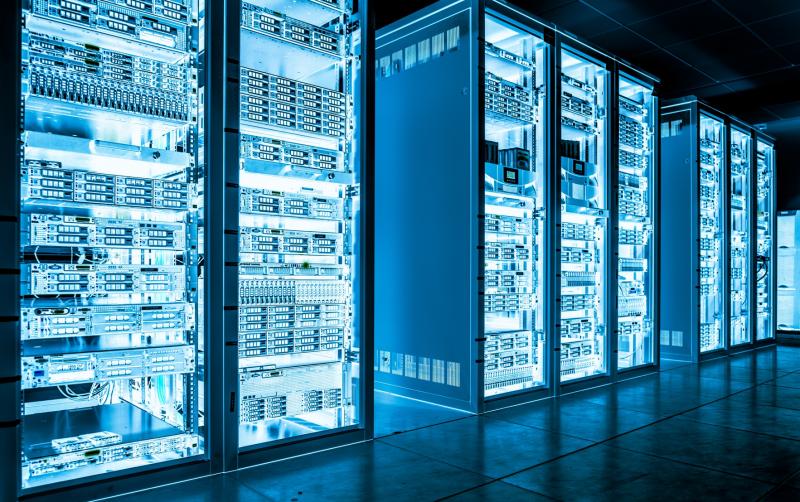
The odds are good that radiologists want to believe in artificial intelligence (AI). The hype from vendors, professional societies and the media has been pointing them in that direction for the last couple years. Unfortunately, if history is a guide, there is a good chance that medical AI will fall short. This must not happen. The potential benefit of AI is too great for it to fail again.
The last time AI flopped was in the mid-1980s, after skyrocketing expectations. Sadly, failure was well within the mainstream of that period.
The medical community and public began the decade agog with antibodies made by patient cells hybridized with cancer, so-called “hybridomas.” These “magic bullets” were supposed to cure cancer. They did not.
“Cold fusion” ended much the same. A lot of sizzle. No steak.
There is a distinct possibility that we are setting ourselves up for the same kind of disappointment as we enter the third decade of the 21st century. Will the current foray into AI end in the same crater that befell the previous attempt? Or in the crater that became the resting place of the first “golden age” of AI, which thudded in the mid- to late-1940s?
Reason To Believe
As it has in these and other ill-considered endeavors, my profession is adding to this threat by stoking expectations about what AI might do. It’s easy to get caught up in the excitement — to herald the positives of AI and its “breakthroughs;” to present the opinions of AI advocates as fact when they are far from it.
While technically accurate in that the quoted and paraphrased statements about AI may indeed have been said by sources, the articles too often have been overly positive. The claims that AI might benefit the practice of medicine and patients are speculative. They are not sure things.
Acknowledging the role of magazines, newspapers and websites in hyping AI is less mea culpa than segue into the far weightier — and more critical — issue of how the medical community can keep from being disappointed. Doing so does not involve the development or validation of these algorithms —
but rather the careful evaluation of them.
As the leaders in medical imaging, upon which much AI effort has focused, radiologists must demand evidence that smart algorithms not only meet their claims but that they produce practical benefit.
What Regulators Do
You might think federal regulators (for example, those at the FDA) would be the ultimate arbiters of product claims. After all, they have been assigned to guard a government-constructed gate to the commercial market. Yet, as incongruous as it may seem, they typically review AI products through a process that compares them to commercial products. This is wrong for two reasons.
First, it is wrong-headed. This regulatory process, which results in a 510(k) clearance, requires that proposed products show “substantial equivalence” to ones already on the market. It is so-named because it refers to section 510(k) of the Federal Food, Drug and Cosmetic (FD&C) Act of 1938. The Medical Device Amendments of 1976 extended the FDA’s control to include medical devices.
By definition, AI products have no market precedents. They use algorithms that learn from data rather than ones that are programmed to perform specific tasks. As such, they are unique. (Although some vendors claim that their products are artificially intelligent even when they do not involve machine learning, for this commentary we will stick to machine learning as a necessary characteristic of AI.)
Second, since the 510(k) clearance process was enacted, the FDA has attempted — particularly in efforts in and around 1998 — to reduce the burden of a growing backlog of device applications. Today, the 510(k) process is a bureaucratic means for the FDA to expeditiously review applications for medical devices.
Consequently, the buyers of AI products, and the media who report on them, may be tempted to — but should not — believe that successfully completing FDA review attests to the value of sellers’ claims. This is unabashedly not the case. By not requiring clinically based evidence, the 510(k) process is typically chosen because it is the least intrusive of any regulatory mechanism and promises vendors the fastest and best return on their investments.
The FDA might accept them into this process because pushing applications through regulation blunts the charge often made by FDA critics, that the agency obstructs progress.
What no one — neither vendor nor regulator — says is that when AI products are reviewed through this process, the benefit of AI algorithms is seldom — if ever — part of the review process. This means the 510(k) clearance of a product for commercial sale is not enough reason for care providers to believe in it. Only the medical community can judge whether an AI product is beneficial.
Determining Value
Caveat emptor, therefore, is — and should be — in effect. The damages that come from making a wrong purchase decision could be to the care of the patient for whom the physician is directly responsible.
With so much at stake, it stands to reason that not only should the claims associated with an AI product be real, but the practical result of those claims should be validated or, at the very least, carefully examined. Further, claims and potential benefits should be vetted by providers before the product is applied. This goes for clinical and non-clinical algorithms alike, because even non-clinical algorithms designed for medical environments may impact patients.
For example, a vendor may claim that an AI algorithm can increase efficiency. A care provider might put such an algorithm into practice to reduce costs by increasing volume and throughput. In so doing, that algorithm might help staff accelerate their schedules. But failure to achieve this objective could make care less convenient for patients. The use of that algorithm, therefore, could impact patients.
Specifically, an algorithm might address patient positioning. Not only might its use affect the speed with which an exam is conducted and how well the staff stays on schedule, it might impact the amount of radiation the patient receives, thereby directly affecting patient safety.
While it may be obvious that AI must be held accountable, you might ask — on what criteria should providers evaluate claims? This gets back to the need for evidence to support claims.
While helpful, anecdotal evidence — stories that describe useful application of an algorithm — should not be considered sufficient. Statistically based evidence is needed to show incontrovertibly that the software lives up to claims — and that its use produces a practical benefit.
If, for example, improved positioning is the claim of an AI program, then the denominator of success should not be narrowly defined, for example, as a reduction in the number of adjustments made in patient positioning. Rather the practical benefit derived from implementing the algorithm should be at least one of the metrics. Is there evidence to indicate that use of the algorithm improves patient positioning so that it takes less time? If so, how might this allow the technologist either to accelerate the schedule or spend more time with the patient? Or — is there evidence that improved patient positioning due to the algorithm results in less patient exposure to radiation (and, if so, how much less)?
Yes, demanding evidence of practical benefit coming from AI sets the bar high. But that is where it needs to be, if AI is to avoid history’s painful lessons.
Related content:
Technology Report: Artificial Intelligence 2018
VIDEO: RSNA Post-game Report on Artificial Intelligence
VIDEO: AI, Analytics and Informatics: The Future is Here