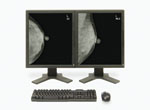
Mammography and breast magnetic resonance imaging (MRI) are tried-and-true methods for spotting and preventing the spread of breast cancer and other abnormalities. Mammography alone detects up to 90 percent of breast cancers in women over 50, according to the U.S. Food and Drug Administration (FDA). Difficult and missed readings remain a prevalent issue for doctors, however, especially when treating younger women. But recent advances in technology are helping to combat the problem and make breast cancer more manageable, both by helping diagnosis for patients and by increasing productivity and accuracy for doctors.
Computer-aided detection (CAD) reviews a patient’s mammogram or MRI after a doctor has made an initial interpretation — although increasingly it is being done before it is physically placed in a doctor’s hands — operating as an additional set of eyes. If the software detects abnormalities on the image of the breast, it flags the region so a radiologist can review and interpret the area in question.
“It’s just like a spell-checker that alerts you to a mistake you may have missed in a document. You will know it is misspelled once you see it, but you will overlook mistakes — it’s hardwired into our systems,” says James Ruiz, M.D., department of radiology, Woman’s Hospital, Baton Rouge, La.
The Woman’s Hospital has used Hologic CAD systems since 2004. While files were previously fed through a machine, the hospital went digital in 2005. Today, its 45,000 yearly screening and diagnostic studies, as well as its floor-view exams, are automatically run through computer-aided technology.
“Mammographic CAD helps us to overcome the inherent difficulty in being perfect,” Ruiz explains, “and provides an aid to help maintain concentration on the task at hand.”
The software for its CAD model, the R2 ImageChecker, is designed using algorithms that are calculated to search for microcalcifications and masses — features commonly associated with breast cancer. The software evaluates characteristics like degree of spiculation, lesion shape, contrast to the surrounding tissue, texture of the lesion and edge texture. As these features become more prominent, so do the software’s marks, indicated as either an asterisk or a triangle, depending on the type of detection.
Ruiz, like many in the field, says CAD’s weakness is its false positive rate. He says that it is similar to when a colleague brings film and asks you to look at a case to help determine whether a finding is significant. Discernment is necessary when looking at CAD’s results.
“Statistically, it’s going to bring me more cases that are unimportant than are important,” he says. “I don’t view that as being necessarily bad — I can ignore those false positives. On the other hand, in certain situations, there will be marks that are true positive that I might not have seen if [the CAD mark] wasn’t there.”
The ImageChecker software comes with sensitivity settings to give users screening flexibility. A region will be marked only if it falls above a selected sensitivity range or operating point. Users can choose a setting for both calcifications and mass detections, giving doctors and radiologists nine sensitivity combination options.
More Pluses than Minuses
Despite the technology’s false positive rate, Ruiz doesn’t see many true drawbacks to using CAD. He finds that problems that arise have more to do with how humans interact with the program.
“CADs are good at spotting microcalcifications, for example, so it would be seductive, but wrong, for a doctor to look for fewer because the CAD is doing it for him. But CADs are not qualified to read the mammogram — that’s what doctors are paid for,” he says. “Any problems blamed on CAD should be more appropriately blamed on the misuse of CAD.”
Furthermore, Ruiz says any drawbacks in productivity that arose with analog modalities have been virtually eliminated with digitalization of computer-aided detection systems, because embedding the system into the modality means “the process became invisible.”
Rachel Brem, M.D., doesn’t see a downside to using computer-aided technology, either. Brem, director of the Breast Imaging and Interventional Center, professor of radiology, vice chair of research and faculty development, The George Washington University (GWU) Medical Center, Washington, D.C., says the technology has become an integral part of the center’s mammography and breast MRI workflow.
“Every case has it; not every case needs it,” she said. “But we know it’s better with it.”
The GWU Medical Center has been using iCAD computer-aided technology for more than 10 years, most recently, the SecondLook Digital for mammography and SpectraLook for breast MRI. SecondLook Digital is compatible with both direct digital and computed-radiography digital mammography systems from leading imaging vendors, including GE Healthcare, Hologic, Siemens Medical Systems, Fujifilm Medical Systems and Philips. It seeks to enhance workflow by enabling DICOM connectivity for acquisition systems, review workstations and picture archive and communication systems (PACS) alike. Brem performed several studies on the effectiveness of computer-aided detection for mammography and found that the technology helps detect about 20 percent more cancers than would be detected without it.
In addition to mammograms, GWU performs five to six breast MRIs every day, all of which include CAD. Brem says MRIs provide information the others don’t because mammography and ultrasound only look at the anatomy, whereas the MRIs examine blood flow and can provide far more sensitivity.
“It also takes an hour, requires an injection and so forth,” she said. “You have to weigh the benefit against the inconvenience. For most women, mammography and ultrasound are sufficient.”
But she says that for women with the breast cancer gene or others in critical situations or with a risk greater than 20 percent, an MRI is recommended. In fact, Brem says 10 percent of women who get a breast MRI before surgery find another cancer, and nowadays, “it’s unusual to do an MRI without CAD.”
SpectraLook, like most breast MRIs armed with computer-aided detection, aims to help doctors evaluate lesions. In particular, Brem says CAD has become an analytic tool to differentiate benign from malignant lesions, interpreting and observing an area’s uptake of contrast with the knowledge that blood goes in and pumps faster in cancer. In her opinion, the SpectraLook stands apart because of its analysis of morphology. She adds that the technology has the “most sophisticated analysis of workflow and integration” for the hospital, including motion correction, subtraction images, multi-planar reconstruction and maximum-intensity projections (MIPs).
Brem compared the helpfulness of computer-aided detection to searching for a lost item in a familiar environment. “How often are you late for work, looking for your keys and you find them sitting right on your table?” she asked. “You see them all along, you just don’t perceive them. [CAD] is a safety net that allows us to diagnose better.”
She looks forward to the future of CAD technology, citing the possibilities of looking past its binary limits and being able to stratify lesions and consider other factors, such as risk and family history. But although expectant about the technology’s prospects, Brem acknowledges the field has come a long way.
“It used to be that many doctors didn’t want to do screenings in the afternoon because they thought they would be too tired to do the readings,” Brem explained. “With CAD, the level of confidence has certainly increased.”