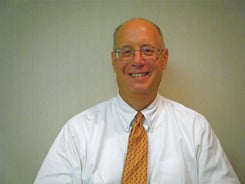
Bill Muhr, M.D., president and CEO of South Jersey Radiology Associates, P.A.
South Jersey Radiology Associates, P.A., (SJRA) is a leading private practice located in the Southern New Jersey corridor near Philadelphia. With nine imaging centers and 45 full- and part-time radiologists, SJRA provides a full complement of medical imaging services — from standard X-rays to the most complex procedures. SJRA also covers three hospitals totaling 500 beds in the Virtua Health System. Bill Muhr, M.D., president and CEO of SJRA, recently spoke about the implementation of the Qualitative Intelligence and Communications System (QICS) offering from peerVue.
Q: What are the challenges of ensuring quality services and workflow in a large practice?
A: As we looked to the future and what we wanted to do as a practice, we broke down the quality processes in radiology into three broad areas: technical or equipment, professional or the radiologist, and customer service. The first and third are, in some respects, easier to deal with; however, the professional can be
challenging within radiology.
As we looked at professional quality measurements, one area we clearly wanted to implement was a peer review process — looking at the quality of the reports generated by our radiologists and capturing that data in a fashion that was easy for the radiologists and not seen as an interference or hindrance to their normal workflow. It was important to maintain efficiency yet have our radiologists embrace peer review.
The American College of Radiology (ACR) developed the radiology peer process, which was initially a paper process and now is electronic. However, it was a relatively small number of cases each day done in a retrospective fashion. Honestly, at the end of the day, people just wanted to get it done and be able to leave for the day.
Q: What led you to investigate a solution for your peer review process?
A: We realized that when we’re reading cases in our routine workflow, we are comparing a current study to an old study. Essentially, we were doing peer review every time, so why not capture that data? So we approached peerVue with the idea to conduct peer review prospectively rather than retrospectively, and integrate the process into our normal workflow. And that was how we started doing it, beginning with a fairly small number and then increasing it each day to the point where the radiologists now see it as a seamless part of their workflow.
Q: Why did you select the QICS solution?
A: The idea of an electronic peer review that seamlessly integrated with our PACS, allowing radiologists to capture the data throughout their workflow, was really the key process we focused on. We looked at several different solutions, but one of the aspects we liked most about QICS was the rules engine, the logic and the flexibility of it.
peerVue was also very interested in our approach to capturing the report quality data. Remember, everyone had been doing only the classic ACR-type peer review. When we spoke to peerVue about integrating what we call “report quality,” they were interested in working with us to develop that process.
Q: How is QICS performing in your organization and what value does it add?
A: The main value is in improving our quality. Clearly that’s the focus of a peer review process. Our radiologists have embraced it and don’t see it as a “gotcha,” but rather that it helps them recognize there are particular areas where they need to improve.
That’s the type of quality improvement that it’s designed to do, to prevent a problem (in reading) ending up as a bad outcome. That is very valuable to me when I go to payers and explain what we are doing to really improve the quality of the product we are delivering to them and their patients.
Also with QICS we have the ability to capture technical quality improvement data. As our enterprise grew, and people were rotating around various sites, it became very difficult to give feedback to the technical staff on a daily basis about the quality of their work. With QICS, we can capture this, not just the negative, but also the positive.
The radiologists can anonymously give the techs valid and quantifiable feedback on the quality of their work to help them improve. By taking out the human element, the radiologist can simply focus on the quality of the delivered product and provide feedback to the manager whose job is structured to deliver that feedback. If you think about the nature of most people, we don’t like to give negative feedback, it’s a challenge. This blind peer review enables our organization to synthesize the information from everyone in the organization.
Q: How does QICS help you improve the delivery of care?
A: peerVue obviously has solutions other than the peer review process; one area is the method of delivering results back to physicians. I like to use the term “urgent results” as the term “critical results” is a misnomer, especially in outpatient facilities. While the results may not be life-threatening, we want the doctor to know that there is something requiring additional follow-up, such as a nodule. This provides a newer, better way to document that communication and ensure those results are getting back to the referring physician. I think even delivering those results and communications direct to patients presents future opportunities as patients assume more control over their own records.
Q: Is there any additional advice for someone who is considering a solution like this?
A: One piece of advice I’d give any practice is to utilize the peer review process as a seamless, normal part of the workflow. Don’t make it an add-on, or something people feel they have to do outside of the normal workflow. Once it is routine, it becomes part of your normal quality. That’s what we need to do in radiology, more routine checklists, just like the pilot who performs his pre-flight checks. We need to do more of that type of thing in medicine.
Case study supplied by peerVue