
Greg Freiherr has reported on developments in radiology since 1983. He runs the consulting service, The Freiherr Group.
Artificial Intelligence: Promise or Pitfall for Radiology?
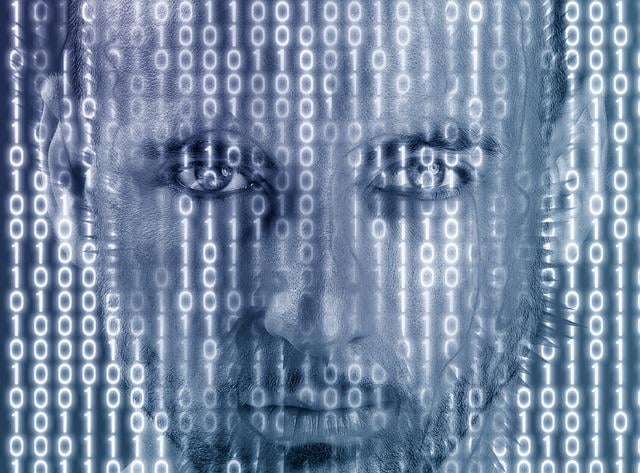
Image courtesy of Pixabay.
Radiologists use visual pattern matching in much the same way referring physicians visually assess their patients. The way radiologists and referring physicians work — and especially how they interact — is radically changing, however, with digitalization of the medical record, the ubiquity of smartphones and the availability of digital photography.
Artificial intelligence (AI) may be both promise and pitfall for radiology in this changing landscape. How it should be considered, however, is as common ground for the whole of medicine to advance.
Today a picture is truly worth a thousand words. The picture of a child’s inflamed ear, snapped on a cell phone and sent digitally to a pediatrician, explains better what is going on than a worried parent could say.
As enterprises are image-enabled, the prospect of physicians being inundated by more data than they can absorb, catalog, archive and recall becomes all but inevitable. This affects not just those outside radiology but within, as radiologists strive to work more closely with care providers whose effectiveness is growing ever more dependent on the efficiency by which images can be managed.
Here is where artificial intelligence may provide common ground. These machines, armed with deep learning (DL) algorithms, promise to make sense of visual patterns and in so doing serve as tools to manage the rising tsunami of medical images — radiological and otherwise.
Radiologists might become more efficient and effective in handling emergent cases (see “Will Artificial Intelligence Find a Home in PACS?”). So too might they use DL algorithms — trained to recognize key bits of information — to comb electronic medical records (EMRs) for information that will give radiologists the context to better understand what they see in patients’ images (see “Watson, Come Here … Radiology Needs You”).
Used as smart tools, these algorithms may improve radiologists’ efficiency, prioritizing cases during the examination that require immediate attention (see “Smart Scanners: Will AI Take the Controls?”). Built into the scanners themselves, these algorithms might improve the efficiency by which examinations are performed, shortening exam times by ending data acquisition when certain thresholds — set by the radiologist — are met, thereby increasing patient throughput and boosting revenues.
Special kinds of artificial intelligence, such as swarm AI, which amplifies human knowledge, might turn radiology groups into super experts, giving them the human-based tools to handle the toughest cases (see “Diagnostic AI: By the People, of the People, for the People”). Further down the road, DL algorithms might be trained to recognize disease patterns, identifying and outlining masses, measuring them, and transposing the measurements into the radiology report, even possibly one day directing the attention of radiologists to suspicious areas in images so as to make efficient use of radiologists’ time, while reducing the possibility that disease might be overlooked (see “Watson, Come Here … Radiology Needs You”).
Yet, here at this diagnostic nexus, is where the promise of AI may turn to pitfall for radiologists. For a very long time, the art of interpreting medical images resided principally with radiologists. DL algorithms threaten their unique position as interpreters of these images.
Hanging in the balance is the “specialness” that has been woven historically into the fabric of radiology. As smart machines acquire some of the interpretive powers of radiologists, they may be harnessed by referring physicians to render radiological insights, further threatening the position of radiologists in medical practice.
Yet this can be reconciled in the broader perspective of collaboration. Radiologists want to become a bigger part of the care management team (see “Radiology: Going Along to Get Along”). DL algorithms that translate and summarize EMRs for radiologists could provide the basis for understanding quickly and efficiently the non-metric, unstructured observations that referring physicians regularly pack into these records. By interpreting medical images in the context of EMR-based data, radiologists could achieve a synergistic understanding of the patient’s condition that transcends their current capability, laying the foundation for them to join the care management team.
Further still, DL algorithms, fashioned into clinical decision tools, might help referring physicians identify and narrow the choices of different scans based on clinical observations in the EMR. Increasing the appropriateness of ordered exams, in turn, could decrease costs for additional testing, as they improve patient care, making radiology an even more integral part of medicine.
While the human species as a whole may have something to fear from artificial intelligence, so have said Elon Musk, Bill Gates and Stephen Hawking (see “Will Smart Medical Machines Take Us to the Eve of Destruction?”), radiologists have more to gain than lose.
Candidly, I doubt seriously that radiologists will ever be replaced by smart machines, no matter how smart machines become. Here are two reasons why.
First, regulatory agencies will not sanction the use — or, in the case of the FDA, the marketing — of machines that confer medical diagnoses on their own (see “Will the FDA Be Too Much for Intelligent Machines?”). Second, if such machines did somehow become commercially available, referring physicians would never accept conclusions or interpretations drawn solely by them. Physicians have balked at the underlying concept of machines forming medical diagnoses, which is one of the prime reasons that the “D” in CAD came to stand for “detection” rather than “diagnosis.”
In short, the deck is stacked against artificial intelligence. Rather than being a threat to radiologists, AI offers a net gain for those in radiology who use smart machines wisely. Most appealingly, deep learning algorithms might be used as a springboard by which radiologists can increase their value to medical colleagues and, in the process, become more involved in care management.
Editor’s note: This is the third blog in a series of four by industry consultant Greg Freiherr on Working With Smart Machines. You can read the first blog, Will Artificial Intelligence Find a Home in PACS?, here; and his second blog, “Watson, Come Here … Radiology Needs You,” here.
Watch the VIDEO “Examples of Artificial Intelligence in Medical Imaging Diagnostics.” This shows an example of how AI can assess mammography images.
Watch the VIDEO “Development of Artificial Intelligence to Aid Radiology,” an interview with Mark Michalski, M.D., director of the Center for Clinical Data Science at Massachusetts General Hospital, explaining the basis of artificial intelligence in radiology.