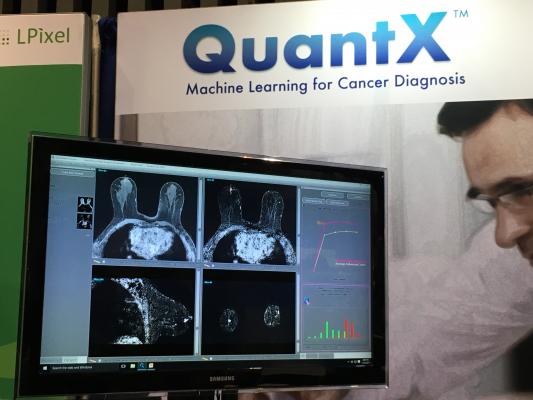
August 13, 2019 — University of California Los Angeles (UCLA) researchers have developed an artificial intelligence (AI) system that could help pathologists read biopsies more accurately and to better detect and diagnose breast cancer.
The new system, described in a study that will be published in JAMA Network Open, helps interpret medical images used to diagnose breast cancer that can be difficult for the human eye to classify, and it does so nearly as accurately or better as experienced pathologists.1
“It is critical to get a correct diagnosis from the beginning so that we can guide patients to the most effective treatments,” said Joann Elmore, M.D., MPH, the study’s senior author and a professor of medicine at the David Geffen School of Medicine at UCLA.
A 2015 study led by Elmore found that pathologists often disagree on the interpretation of breast biopsies, which are performed on millions of women each year.2 That earlier research revealed that diagnostic errors occurred in about one out of every six women who had ductal carcinoma in situ (a noninvasive type of breast cancer), and that incorrect diagnoses were given in about half of the biopsy cases of breast atypia (abnormal cells that are associated with a higher risk for breast cancer).
“Medical images of breast biopsies contain a great deal of complex data and interpreting them can be very subjective,” said Elmore, who is also a researcher at the UCLA Jonsson Comprehensive Cancer Center. “Distinguishing breast atypia from ductal carcinoma in situ is important clinically but very challenging for pathologists. Sometimes, doctors do not even agree with their previous diagnosis when they are shown the same case a year later.”
The scientists reasoned that artificial intelligence could provide more accurate readings consistently because by drawing from a large data set, the system can recognize patterns in the samples that are associated with cancer but are difficult for humans to see.
The team fed 240 breast biopsy images into a computer, training it to recognize patterns associated with several types of breast lesions, ranging from benign (noncancerous) and atypia to ductal carcinoma in situ (DCIS) and invasive breast cancer. Separately, the correct diagnoses for each image were determined by a consensus among three expert pathologists.
To test the system, the researchers compared its readings to independent diagnoses made by 87 practicing U.S. pathologists. While the artificial intelligence program came close to performing as well as human doctors in differentiating cancer from non-cancer cases, the AI program outperformed doctors when differentiating DCIS from atypia — considered the greatest challenge in breast cancer diagnosis. The system correctly determined whether scans showed DCIS or atypia more often than the doctors; it had a sensitivity between 0.88 and 0.89, while the pathologists’ average sensitivity was 0.70. (A higher sensitivity score indicates a greater likelihood that a diagnosis and classification is correct.)
“These results are very encouraging,” Elmore said. “There is low accuracy among practicing pathologists in the U.S. when it comes to the diagnosis of atypia and ductal carcinoma in situ, and the computer-based automated approach shows great promise.”
The researchers are now working on training the system to diagnose melanoma.
For more information: www.jamanetwork.com/journals/jamanetworkopen
Related Digital Pathology Content
VIDEO: Integrating Digital Pathology With Radiology
References
1. Mercan E., Mehta S., Bartlett J., et al. Assessment of Machine Learning of Breast Pathology Structures for Automated Differentiation of Breast Cancer and High-Risk Proliferative Lesions. JAMA Network Open, Aug. 9, 2019. doi:10.1001/jamanetworkopen.2019.8777