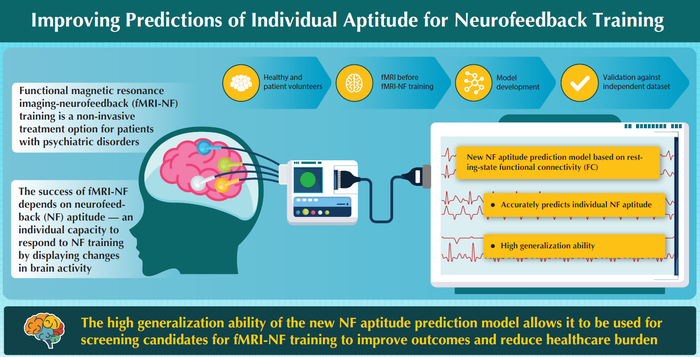
The high generalization ability of the new neurofeedback (NF) aptitude prediction model developed by scientists from NAIST Japan offers a quick, simple and non-invasive method to screen candidates in clinical settings for whom fMRI-NF training would be most beneficial. Image courtesy of Nara Institute of Science and Technology
December 23, 2021 — Advancements in medical science have allowed the treatment of psychiatric disorders like major depressive disorder (MDD) with functional magnetic resonance imaging neurofeedback (fMRI-NF) training. fMRI-NF training is a type of treatment that provides a non-invasive way to control and reinforce brain functions in patients with mental disorders through the use of real-time fMRI monitoring. However, the effectiveness of the treatment is not universal – it is influenced by a parameter called neurofeedback (NF) aptitude.
NF aptitude refers to an individual’s capacity to respond to NF training by displaying changes in brain activity. But NF aptitude varies from individual to individual. Thus, predicting a patient’s NF aptitude becomes important not only for the success of fMRI-NF training, but also to reduce the physical and economic burden on the patient and healthcare system. Thus far, NF aptitude prediction models have focused on specific target regions in the brain, where the NF training was focused. Now, in a new study published in NeuroImage, a group of Japanese scientists, led by Junichiro Yoshimoto from Nara Institute of Science and Technology, Japan, have successfully developed a mathematical model for the prediction of NF aptitude with a high generalization ability.
Speaking about their research, Yoshimoto said, “We applied machine learning, which is an offshoot of artificial intelligence (AI) technology, on data obtained from heathy individuals and patients with major depressive disorder to successfully develop a mathematical model that can predict individual fMRI-NF training aptitude, based on their pre-recorded brain activity at the resting state.”
To arrive at the model, the scientists first studied fMRI images of healthy patients and patients with MDD before fMRI-NF training. They then used these images to calculate the resting state functional connectivity (FC), which describes the correlated or anti-correlated activities in different areas of the brain. They then applied a technique called ‘partial least squares regression’ (PLS) to transform the FC patterns into participants’ NF aptitude. Furthermore, they determined which FCs were most effective for predicting NF aptitude.
They found that the PLS model could be generalized to the independent dataset from other institutes, i.e., it could successfully predict the NF aptitude of individuals based solely on resting-state fMRI scanning. They also found that a part of the brain called the posterior cingulate cortex was the functional hub among the brain regions, suggesting that it plays a major role in NF aptitude. “We believe that our research will help fMRI-NF training become more popular as a non-invasive treatment with minimal side effects for patients with mental health disorders,” concludes Yoshimoto.
Even though the study focused on MDD, the generalizability of the model developed in this study ensures that it can be applied to different neuropsychological disorders, providing hope to patients suffering from mental illnesses and neurological disorders.
For more information: http://www.naist.jp/en/
Related fMRI content:
Functional MRI Provides Encouraging Results in Unresponsive COVID-19 Patient
New MRI Technique Captures Brain Changes in Near-real Time
Machine Learning Uncovers New Insights Into Human Brain Through fMRI